Understanding the Impact of Competing Events on Heterogeneous Treatment Effect Estimation from Time-to-Event Data
arxiv(2023)
摘要
We study the problem of inferring heterogeneous treatment effects (HTEs) from time-to-event data in the presence of competing events. Albeit its great practical relevance, this problem has received little attention compared to its counterparts studying HTE estimation without time-to-event data or competing events. We take an outcome modeling approach to estimating HTEs, and consider how and when existing prediction models for time-to-event data can be used as plug-in estimators for potential outcomes. We then investigate whether competing events present new challenges for HTE estimation -- in addition to the standard confounding problem --, and find that, because there are multiple definitions of causal effects in this setting -- namely total, direct and separable effects --, competing events can act as an additional source of covariate shift depending on the desired treatment effect interpretation and associated estimand. We theoretically analyze and empirically illustrate when and how these challenges play a role when using generic machine learning prediction models for the estimation of HTEs.
更多查看译文
关键词
heterogeneous treatment
AI 理解论文
溯源树
样例
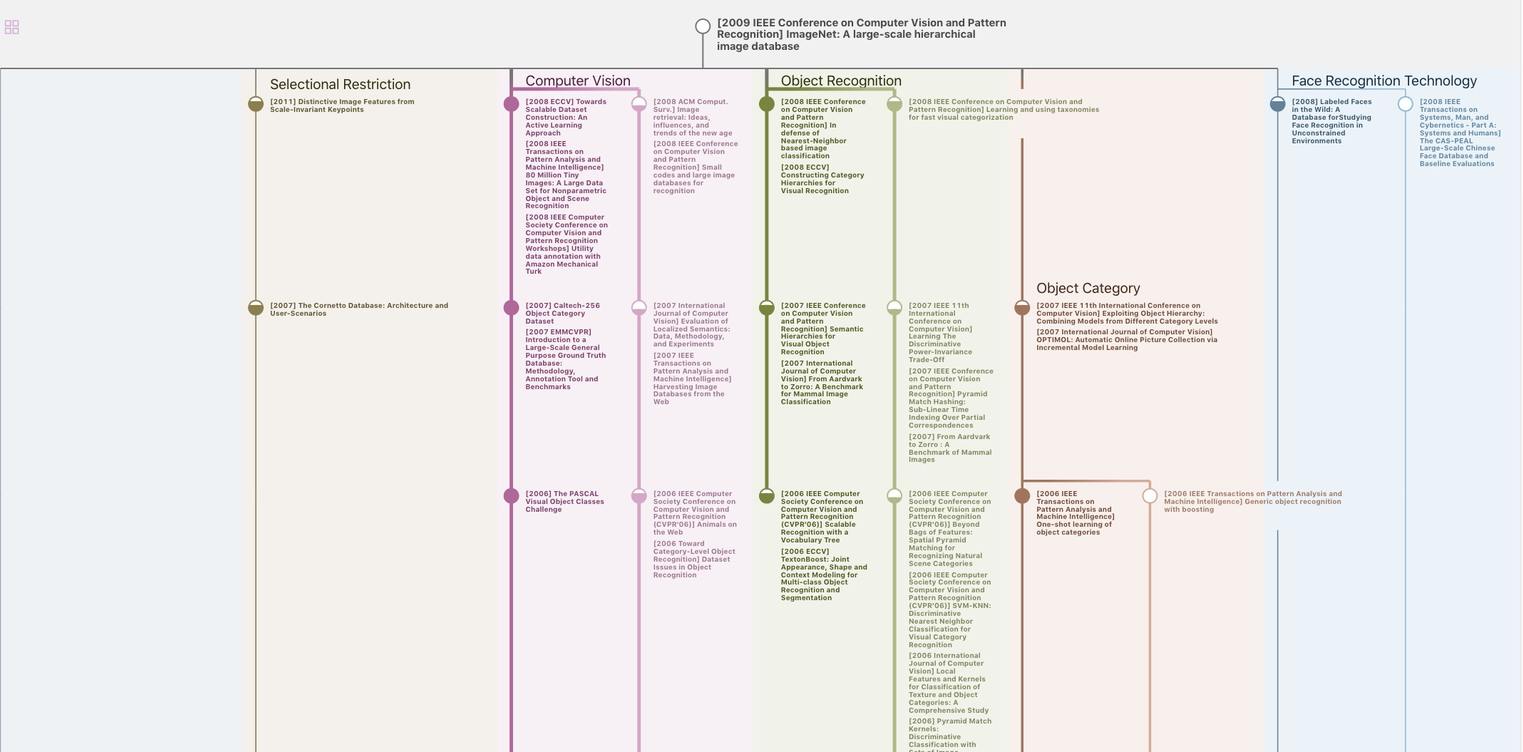
生成溯源树,研究论文发展脉络
Chat Paper
正在生成论文摘要