A Transformer-based Deep Learning Algorithm to Auto-record Undocumented Clinical One-Lung Ventilation Events
arxiv(2023)
摘要
As a team studying the predictors of complications after lung surgery, we have encountered high missingness of data on one-lung ventilation (OLV) start and end times due to high clinical workload and cognitive overload during surgery. Such missing data limit the precision and clinical applicability of our findings. We hypothesized that available intraoperative mechanical ventilation and physiological time-series data combined with other clinical events could be used to accurately predict missing start and end times of OLV. Such a predictive model can recover existing miss-documented records and relieves the documentation burden by deploying it in clinical settings. To this end, we develop a deep learning model to predict the occurrence and timing of OLV based on routinely collected intraoperative data. Our approach combines the variables' spatial and frequency domain features, using Transformer encoders to model the temporal evolution and convolutional neural network to abstract frequency-of-interest from wavelet spectrum images. The performance of the proposed method is evaluated on a benchmark dataset curated from Massachusetts General Hospital (MGH) and Brigham and Women's Hospital (BWH). Experiments show our approach outperforms baseline methods significantly and produces a satisfactory accuracy for clinical use.
更多查看译文
关键词
deep learning algorithm,deep learning,ventilation,transformer-based,auto-record,one-lung
AI 理解论文
溯源树
样例
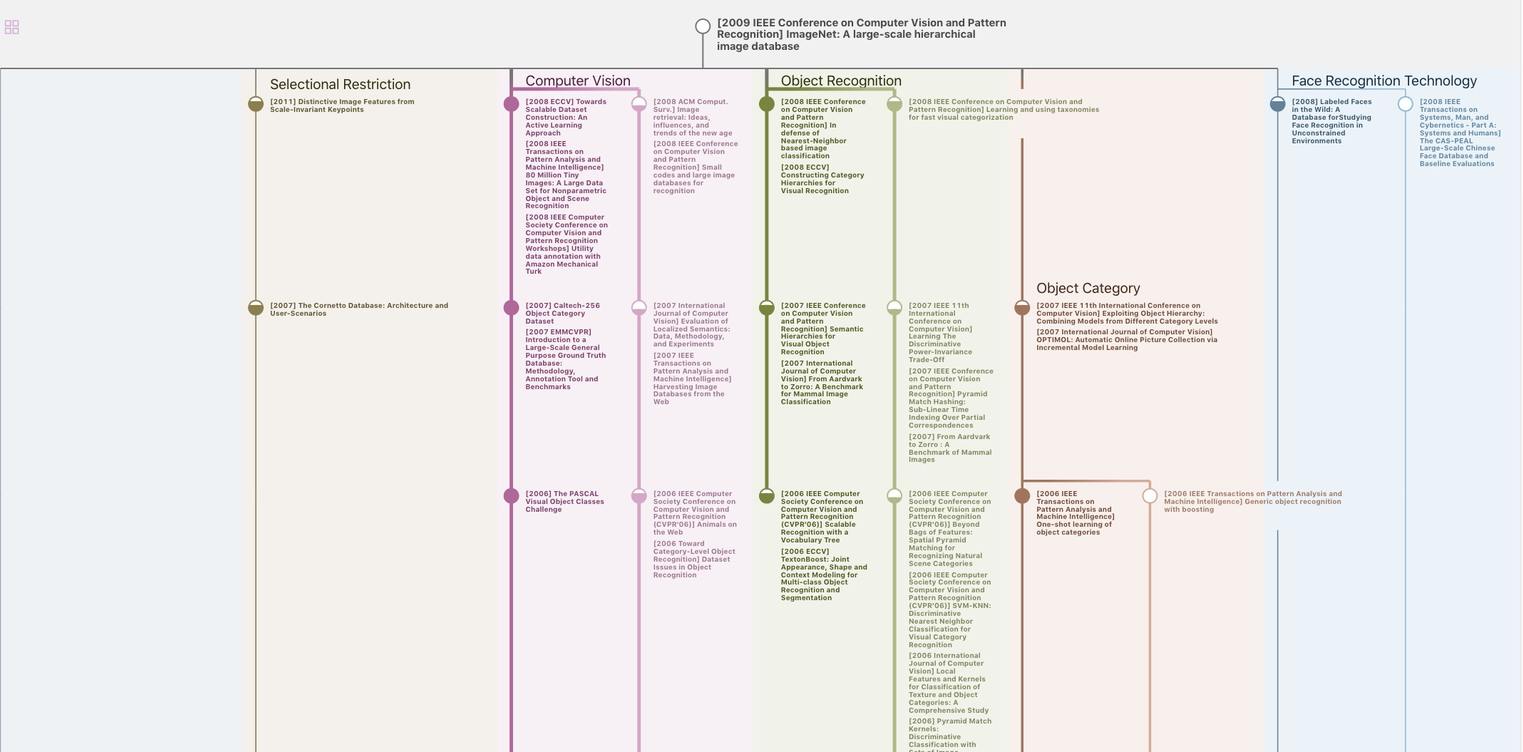
生成溯源树,研究论文发展脉络
Chat Paper
正在生成论文摘要