Recovering Sparse and Interpretable Subgroups with Heterogeneous Treatment Effects with Censored Time-to-Event Outcomes
arxiv(2023)
摘要
Studies involving both randomized experiments as well as observational data typically involve time-to-event outcomes such as time-to-failure, death or onset of an adverse condition. Such outcomes are typically subject to censoring due to loss of follow-up and established statistical practice involves comparing treatment efficacy in terms of hazard ratios between the treated and control groups. In this paper we propose a statistical approach to recovering sparse phenogroups (or subtypes) that demonstrate differential treatment effects as compared to the study population. Our approach involves modelling the data as a mixture while enforcing parameter shrinkage through structured sparsity regularization. We propose a novel inference procedure for the proposed model and demonstrate its efficacy in recovering sparse phenotypes across large landmark real world clinical studies in cardiovascular health.
更多查看译文
关键词
interpretable subgroups,outcomes,sparse,treatment effects,time-to-event
AI 理解论文
溯源树
样例
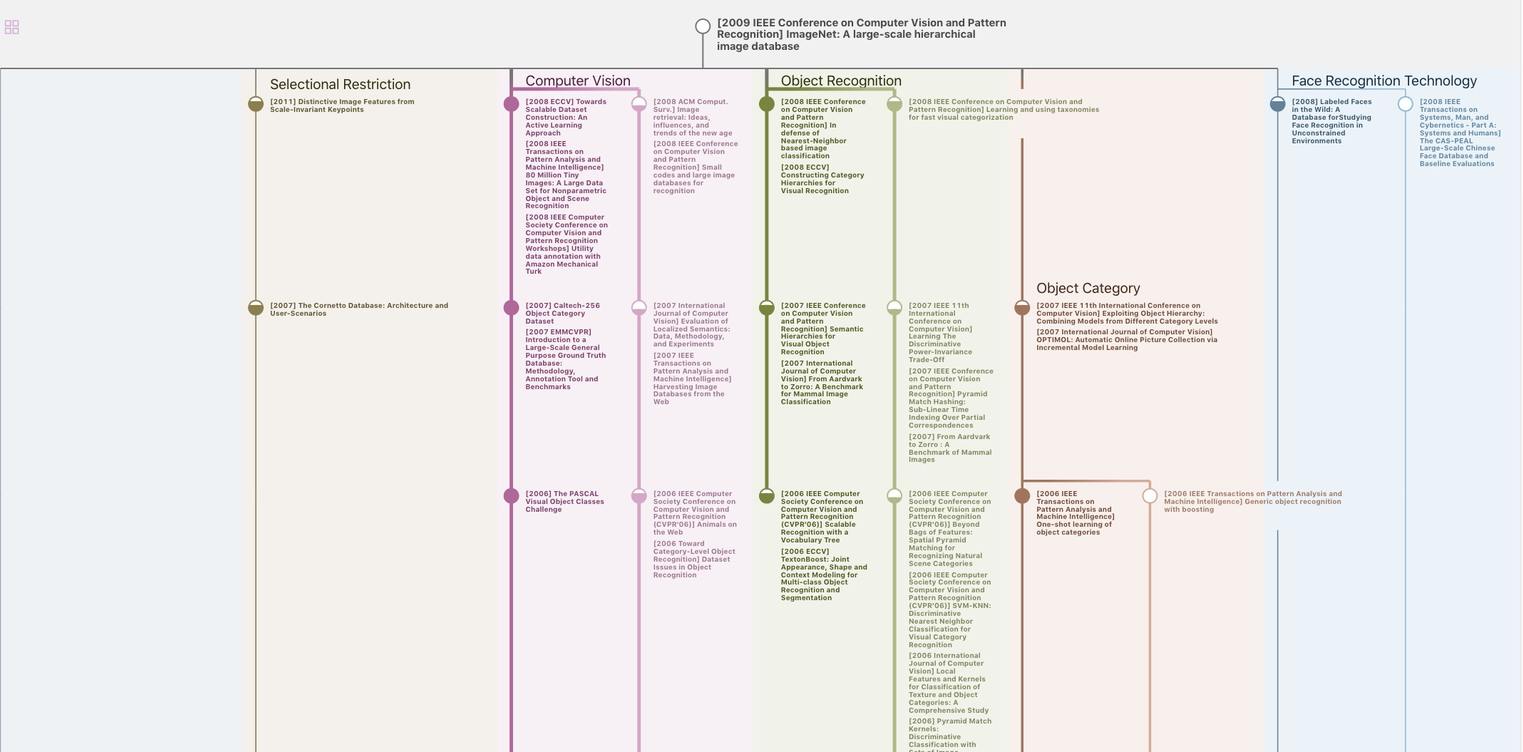
生成溯源树,研究论文发展脉络
Chat Paper
正在生成论文摘要