Factual Consistency Oriented Speech Recognition
arxiv(2023)
摘要
This paper presents a novel optimization framework for automatic speech recognition (ASR) with the aim of reducing hallucinations produced by an ASR model. The proposed framework optimizes the ASR model to maximize an expected factual consistency score between ASR hypotheses and ground-truth transcriptions, where the factual consistency score is computed by a separately trained estimator. Experimental results using the AMI meeting corpus and the VoxPopuli corpus show that the ASR model trained with the proposed framework generates ASR hypotheses that have significantly higher consistency scores with ground-truth transcriptions while maintaining the word error rates close to those of cross entropy-trained ASR models. Furthermore, it is shown that training the ASR models with the proposed framework improves the speech summarization quality as measured by the factual consistency of meeting conversation summaries generated by a large language model.
更多查看译文
关键词
factual consistency,speech recognition
AI 理解论文
溯源树
样例
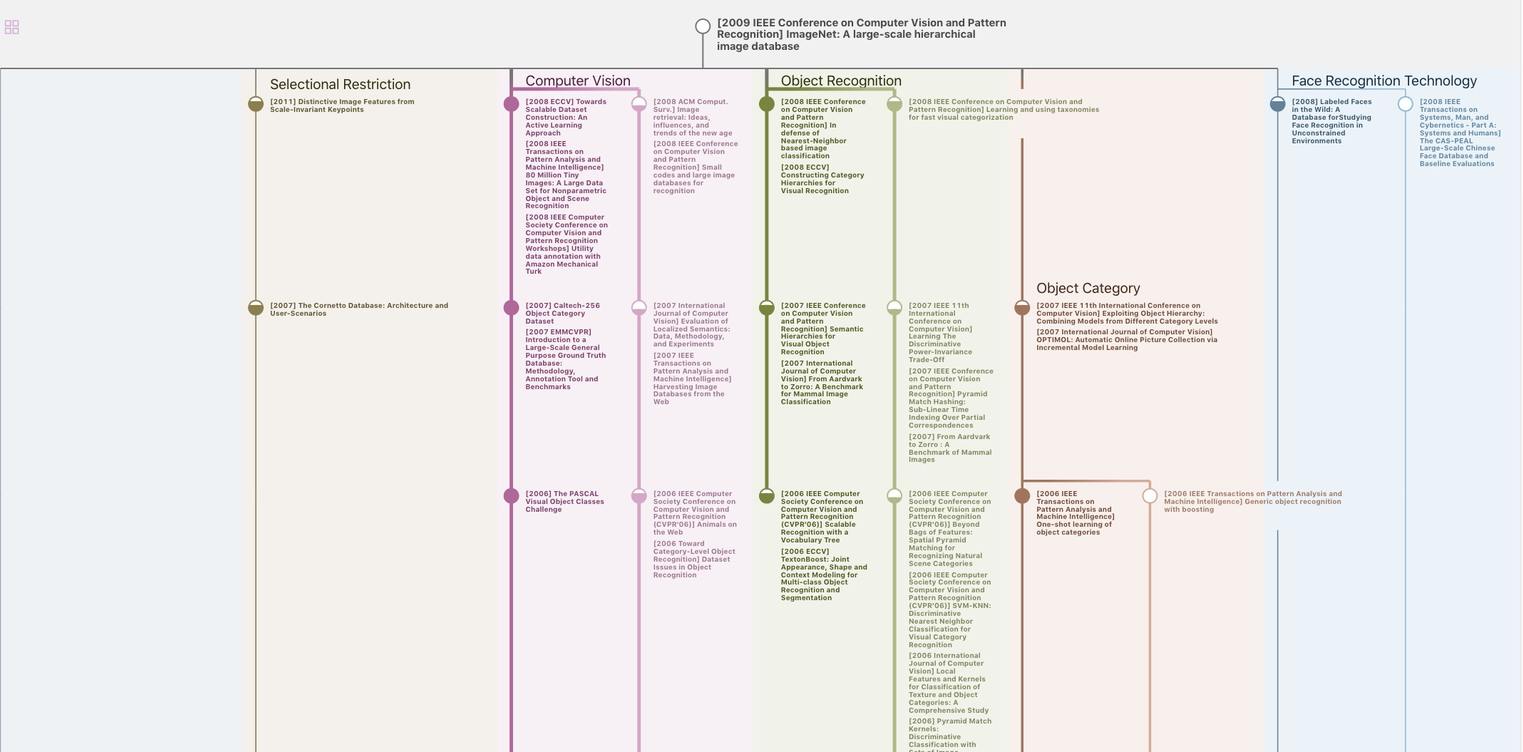
生成溯源树,研究论文发展脉络
Chat Paper
正在生成论文摘要