Coded Matrix Computations for D2D-enabled Linearized Federated Learning
arxiv(2023)
摘要
Federated learning (FL) is a popular technique for training a global model on data distributed across client devices. Like other distributed training techniques, FL is susceptible to straggler (slower or failed) clients. Recent work has proposed to address this through device-to-device (D2D) offloading, which introduces privacy concerns. In this paper, we propose a novel straggler-optimal approach for coded matrix computations which can significantly reduce the communication delay and privacy issues introduced from D2D data transmissions in FL. Moreover, our proposed approach leads to a considerable improvement of the local computation speed when the generated data matrix is sparse. Numerical evaluations confirm the superiority of our proposed method over baseline approaches.
更多查看译文
关键词
federated learning,d-enabled
AI 理解论文
溯源树
样例
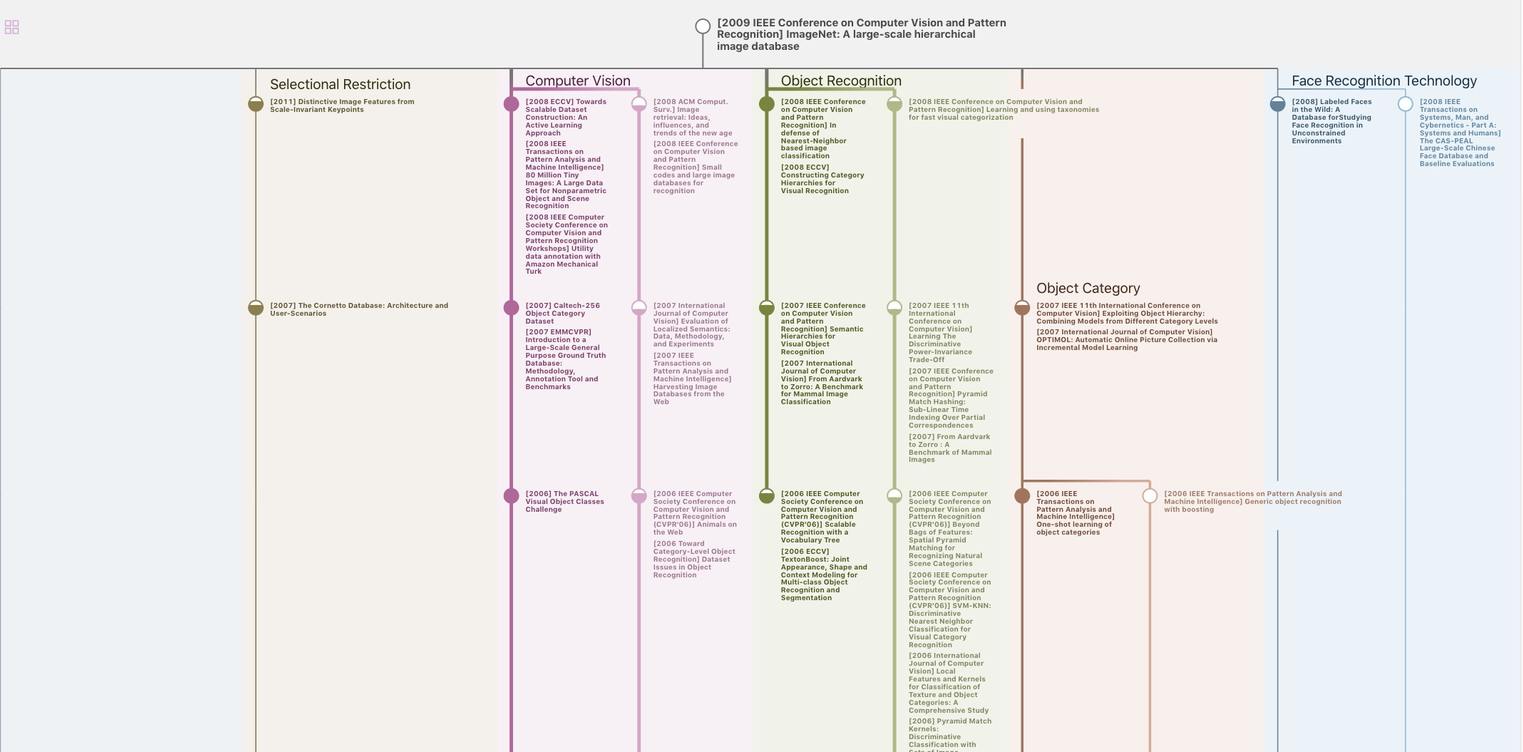
生成溯源树,研究论文发展脉络
Chat Paper
正在生成论文摘要