Efficient and scalable de novo protein design using a relaxed sequence space
biorxiv(2023)
摘要
Deep learning techniques are being used to design new proteins by creating target backbone geometries and finding sequences that can fold into those shapes. While methods like ProteinMPNN provide an efficient algorithm for generating sequences for a given protein backbone, there is still room for improving the scope and computational efficiency of backbone generation. Here, we report a backbone hallucination protocol that uses a relaxed sequence representation. Our method enables protein backbone generation using a gradient descent driven hallucination approach and offers orders-of-magnitude efficiency enhancements over previous hallucination approaches. We designed and experimentally produced over 50 proteins, most of which expressed well in E. Coli, were soluble and adopted the desired oligomeric state along with the correct composition of secondary structure as measured by CD. Exemplarily, we determined 3D electron density maps using single-particle cryo EM analysis for three single-chain de-novo proteins comprising 600 AA which closely matched with the designed shape. These have no structural analogues in the protein data bank (PDB), representing potentially novel folds or arrangement of domains. Our approach broadens the scope of de novo protein design and contributes to accessibility to a wider community.
### Competing Interest Statement
The authors have declared no competing interest.
更多查看译文
AI 理解论文
溯源树
样例
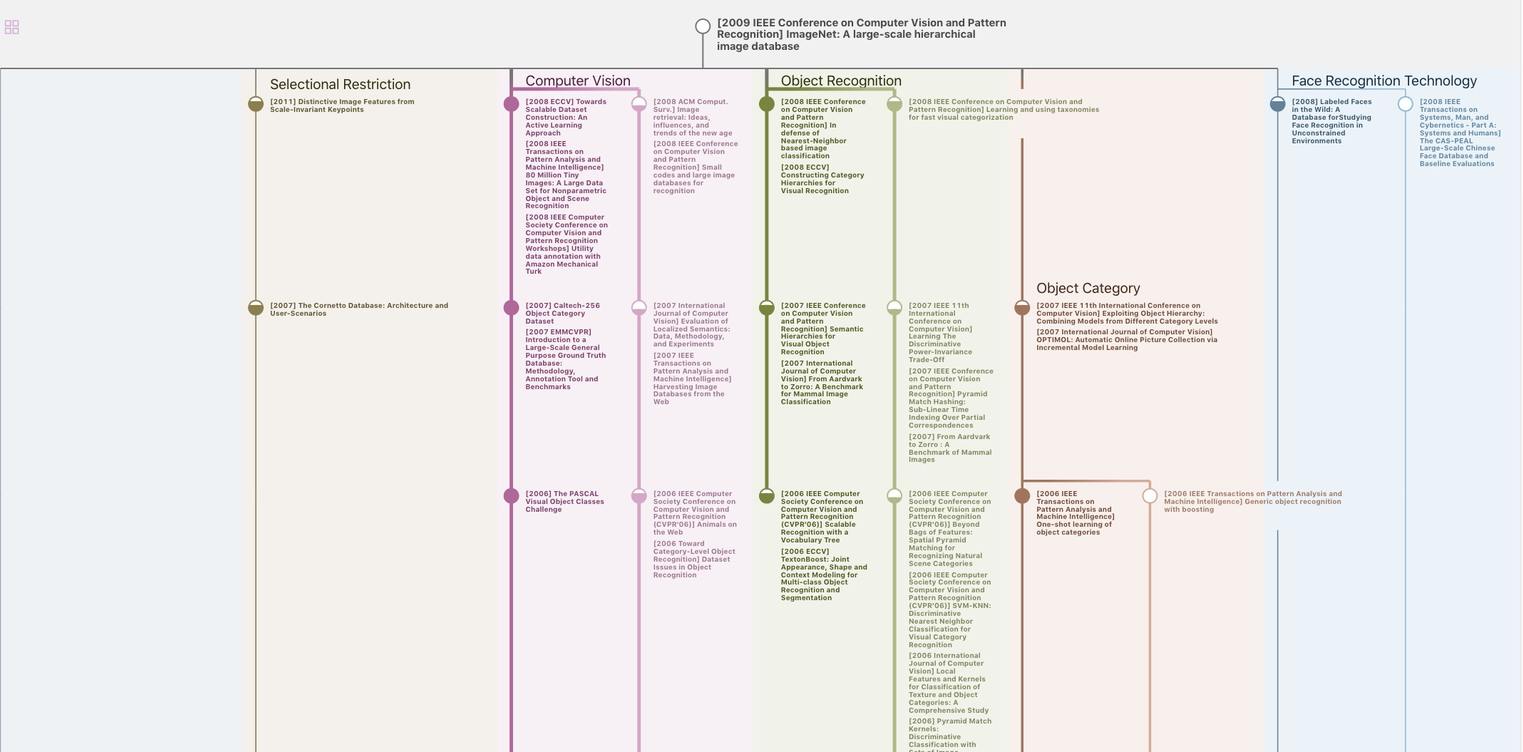
生成溯源树,研究论文发展脉络
Chat Paper
正在生成论文摘要