CO2 and CH4 observation-based budgets in support to future Copernicus CO2 emissions Monitoring and Verification Support (CO2MVS) capacity user communities
crossref(2023)
摘要
<p>Knowledge of the spatial distribution of the fluxes of greenhouse gases and their temporal variability as well as flux attribution to natural and anthropogenic processes is essential to monitoring the progress in mitigating anthropogenic emissions under the Paris Agreement and to inform its Global Stocktake.</p> <p>This study identifies, quantifies and explains possible divergences between global inventories, atmospheric inversions, process-based models, and national inventories submitted to the UNFCCC. The focus is on the EU27, the world’s largest emitters, or other smaller regions that can be used to draw generic results or lessons. The analysis builds on the methodological approach developed previously in the EU-funded VERIFY project’s GHG syntheses (Petrescu et al., 2020, 2021a, 2021b, 2022 and McGrath et al., 2022). Most of the data products are from the VERIFY project, with a gradual inclusion of EU-funded CoCO<sub>2</sub> project’s specific products as the project evolves. Reported inventory-based emissions and removals are generally estimated using bottom-up statistical inventory estimates. Top-down, observation-based estimates are required by multiple stakeholders and at multiple scales to verify bottom-up emission estimates. These estimates are performed at different scales for a variety of applications: the global and continental scale for science purposes, country scale for reporting to the UNFCCC, sub-country scale for urban planning, and point sources like large power plants for verification (Pinty et al., 2019). Several examples will be provided for different sectors of CO<sub>2</sub>/CH<sub>4</sub> budgets to illustrate (e.g., EU27) the aforementioned divergences as well as the current level of convergence between methods.</p> <p>A key conclusion across all components analysed is the difficulty of harmonising datasets into a comparable format. The tradition of comparing datasets as published is easy, but problematic and potentially misleading. To reconcile differences between alternative datasets requires a much deeper understanding of each dataset, such as the system boundaries, methods, and input data sources. Often the necessary data is not available or time consuming to access. A systematic reconciliation and comparison often requires a close dialogue between analysts, data providers, and modelers.</p> <p>Building on our experiences, we discuss a Decision Support Blueprint, outlining potential mechanisms and tools to provide diverse, but targeted, information to the relevant users wanting to reconcile these different datasets. The blueprint is informed by several user consultation meetings and workshops. The blueprint will help tailor a Decision Support System (DSS), as a component of the Copernicus CO<sub>2</sub>MVS, to help inventory agencies, governments and their initiatives (e.g. Covenant of Mayors, C40, ICLEI), industry, NGOs, and other interested actors to utilize the expanding observation datasets to support monitoring and verification activities.</p>
更多查看译文
AI 理解论文
溯源树
样例
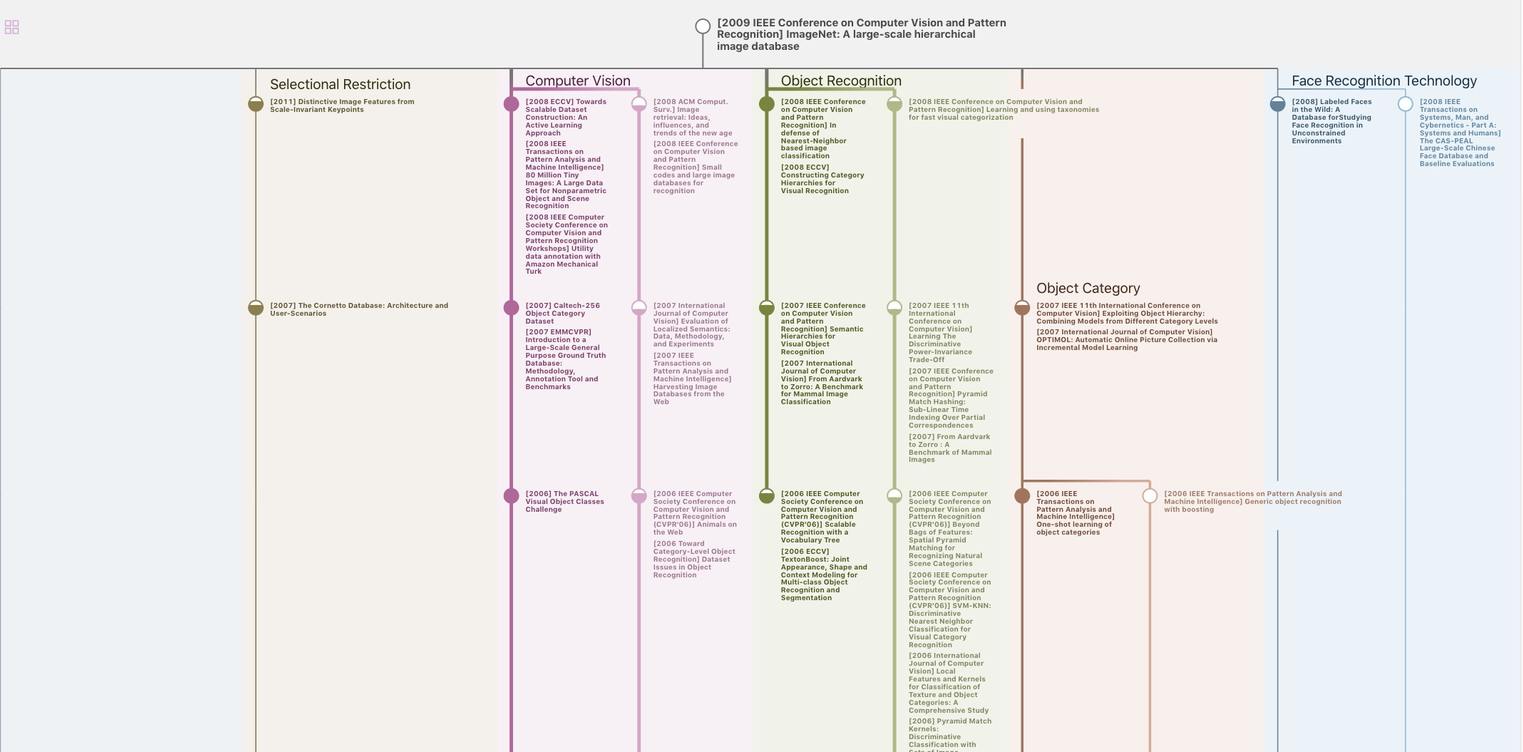
生成溯源树,研究论文发展脉络
Chat Paper
正在生成论文摘要