Pitfalls and opportunities for applying latent variables in single-cell eQTL analyses
Genome biology(2023)
摘要
Using latent variables in gene expression data can help correct unobserved confounders and increase statistical power for expression quantitative trait Loci (eQTL) detection. The probabilistic estimation of expression residuals (PEER) and principal component analysis (PCA) are widely used methods that can remove unwanted variation and improve eQTL discovery power in bulk RNA-seq analysis. However, their performance has not been evaluated extensively in single-cell eQTL analysis, especially for different cell types. Potential challenges arise due to the structure of single-cell RNA-seq data, including sparsity, skewness, and mean-variance relationship. Here, we show by a series of analyses that PEER and PCA require additional quality control and data transformation steps on the pseudo-bulk matrix to obtain valid latent variables; otherwise, it can result in highly correlated factors (Pearson's correlation r = 0.63 ~ 0.99). Incorporating valid PFs/PCs in the eQTL association model would identify 1.7 ~ 13.3% more eGenes. Sensitivity analysis showed that the pattern of change between the number of eGenes detected and fitted PFs/PCs varied significantly in different cell types. In addition, using highly variable genes to generate latent variables could achieve similar eGenes discovery power as using all genes but save considerable computational resources (~ 6.2-fold faster).
更多查看译文
关键词
Single-cell RNA-seq,Pseudo-bulk,Latent variable,PEER factors,Principal component analysis,Normalization,eQTL mapping
AI 理解论文
溯源树
样例
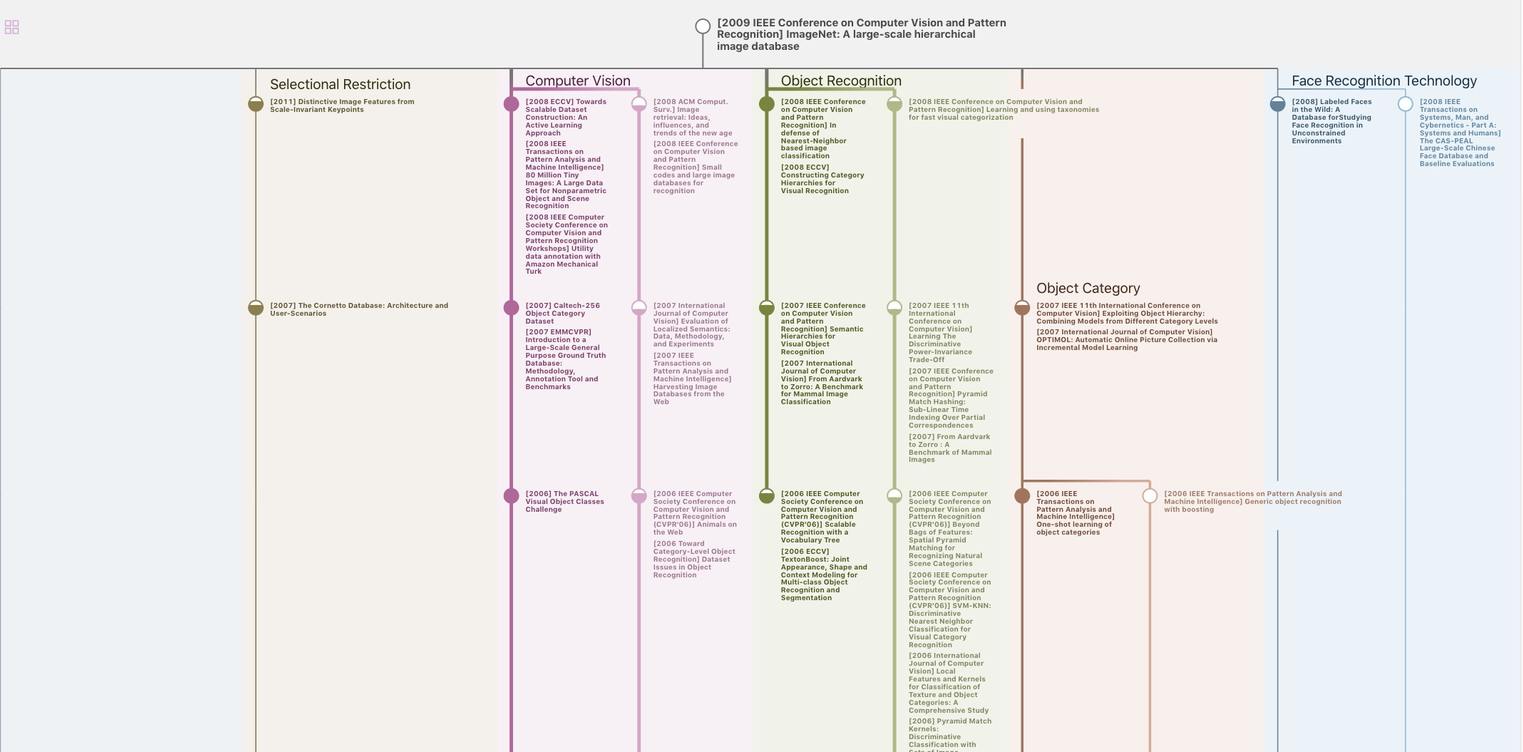
生成溯源树,研究论文发展脉络
Chat Paper
正在生成论文摘要