Velocity loss is a flawed method for monitoring and prescribing resistance training volume with a free-weight back squat exercise
European journal of applied physiology(2023)
摘要
Purpose The aim of this study was to examine the goodness of fit, prediction accuracy, and stability of general and individual relationships between velocity loss and the percentage of completed repetitions out of maximum possible (VL-% repetitions ) in the free-weight back squat exercise. The effects of sex, training status and history, as well as personality traits, on the goodness of fit and the accuracy of these relationships were also investigated. Methods Forty-six resistance-trained people (15 females and 31 males) performed a one-repetition maximum (1RM) test, and two repetitions to failure (RTF) tests, 72 h apart. RTF tests were performed with 70, 80, and 90% of 1RM with 10 min inter-set rest. Results The findings question the utility of using general and individual VL-% repetitions relationships to prescribe training volume with free-weight back squats as (1) the agreement in the % repetitions completed until reaching a given velocity loss threshold across two consecutive testing sessions was unacceptable, regardless of the load used; and (2) the ability of general and individual VL-% repetitions relationships to predict % repetitions in a subsequent testing session were poor (absolute errors > 10%). Sex, training status and history, and personality traits did not affect the goodness of fit of general and individual VL-% repetitions relationships or their prediction accuracy, suggesting potential generalisability of those findings among resistance-trained populations. Conclusions VL-% repetitions relationships do not seem to provide any additional benefits compared to costless, traditional methods and hence should not be used for monitoring and prescribing resistance training with a free-weight back squat exercise.
更多查看译文
关键词
Exercise monitoring,Exercise prescription,Fatigue,Resistance training,Velocity-based training
AI 理解论文
溯源树
样例
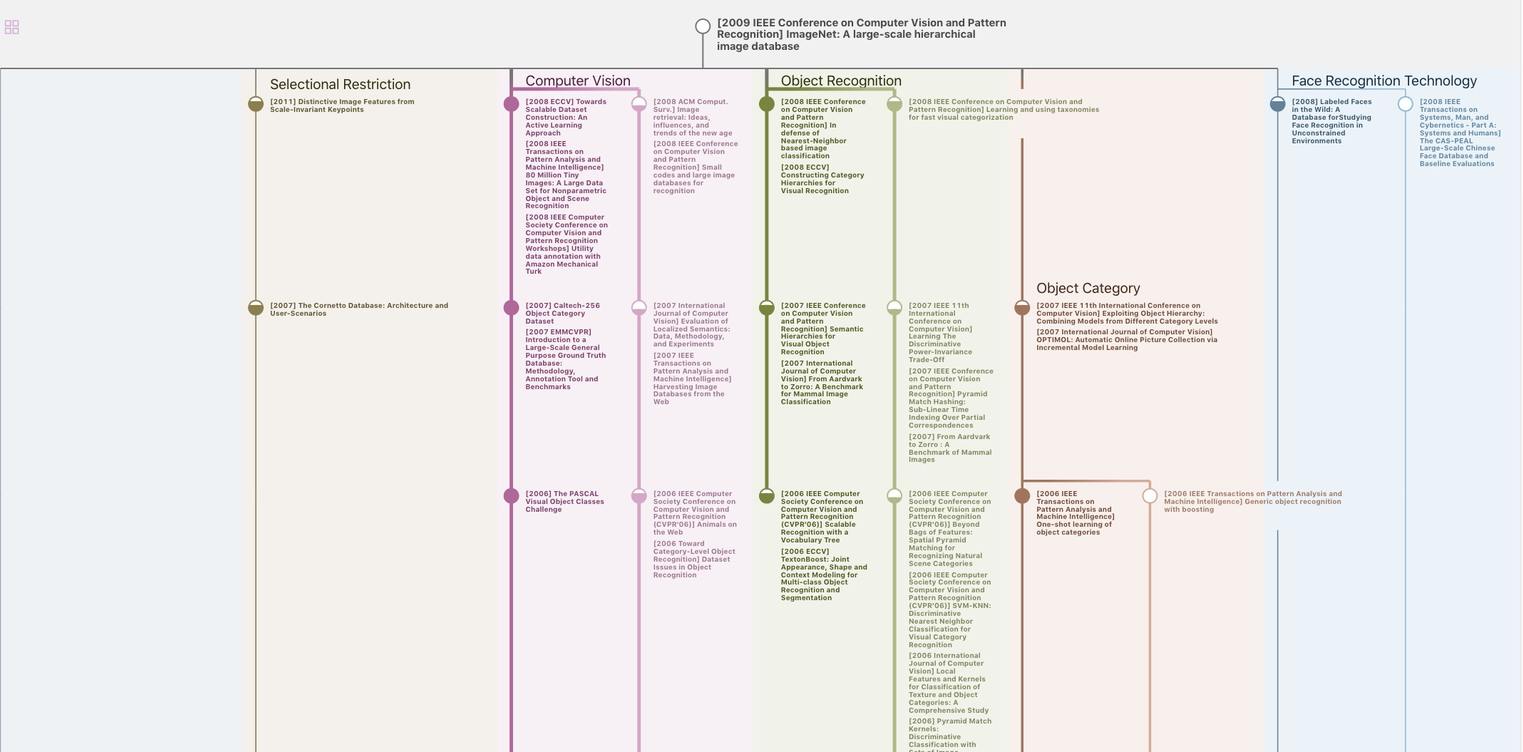
生成溯源树,研究论文发展脉络
Chat Paper
正在生成论文摘要