An artificial neural network-based data filling approach for smart operation of digital wastewater treatment plants
Environmental research(2023)
摘要
With the prevalence of digitization, smart operation has become mainstream in future wastewater treatment plants. This requires substantial and complete historical data for model construction. However, the data collected from the front-end sensor contained numerous missing dissolved oxygen (DO) values. Therefore, this study proposed a framework that adaptively adjusted the structure of embedded filling models according to the missing situation. Long short-term memory and gated recurrent units (GRU) were embedded for experiments, and some standard filling methods were selected as benchmarks. The experimental dataset indicated that the K-nearest neighbor could achieve good filling results by traversing the parameters. The effect obtained by the method proposed in this study was slightly better, and GRU was better among the three embedded models. Analysis of the filling results for each DO column revealed that the effect was highly correlated with the dispersion of DO data. The experimental results for the entire dataset demonstrated that the filling effect of the proposed method was significantly better and more stable than the others. The proposed model suffered from the problem of insufficient interpretability and long training time. This study provides an efficient and practical method to solve the intricate missing DO and lays the foundation for the smart operation of wastewater treatment plants.
更多查看译文
关键词
Wastewater treatment process,Data missing,Adaptive filling framework,Data filling,Smart operation
AI 理解论文
溯源树
样例
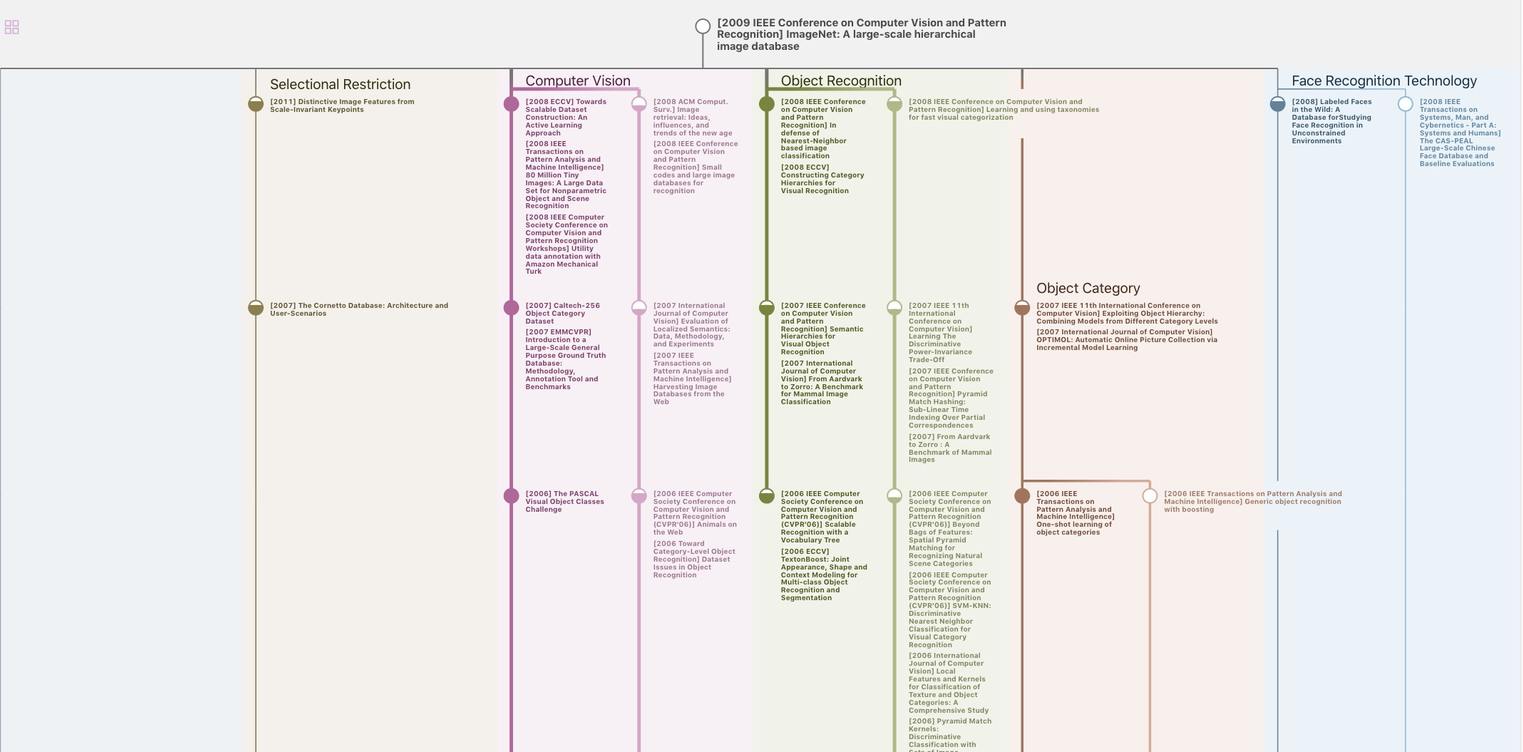
生成溯源树,研究论文发展脉络
Chat Paper
正在生成论文摘要