Fault Analysis of Bearings in Various States Based on Continuous Wavelet and Improved Residual Network
2022 2nd International Conference on Electronic Information Technology and Smart Agriculture (ICEITSA)(2022)
摘要
The bearing fault signals are complex and difficult to collect, which leads to a limited number of fault samples, and it is difficult to analyze the changeable bearing faults with conventional methods. In this paper, an automatic signal feature extraction method by improved residual network and wavelet transform is presented, which is suitable for bearing fault analysis in various states. Continuous wavelet transform (CWT) can obtain enough time-frequency images by superposing the original vibration signals. Improve the residual network, increase the attention mechanism and better deal with complex features. Transfer learning is applied to various working states, and the common features of bearing faults concealment are extracted and learned, so as to improve the applicability of diagnosis. The prediction ability of the bearing is tested in various working states, which proves the applicability of our fault analysis method. The problem of low accuracy of small sample identification caused by lack of actual fault data is solved.
更多查看译文
关键词
Fault Analysis,CWT,Improved Residual Network,Transfer Learning,Bearing
AI 理解论文
溯源树
样例
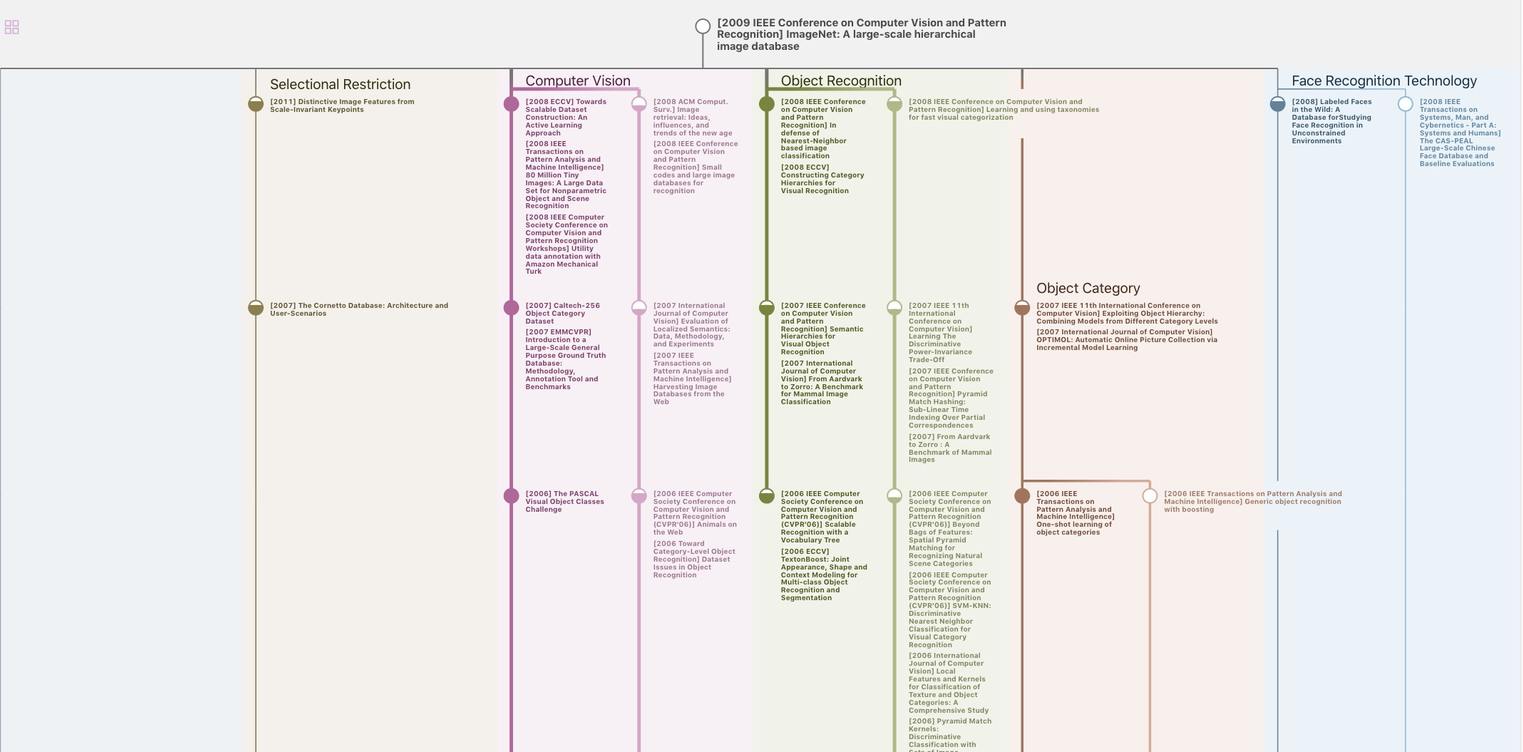
生成溯源树,研究论文发展脉络
Chat Paper
正在生成论文摘要