A Survey of Adversarial Attack and Defense Methods for Malware Classification in Cyber Security
IEEE Communications Surveys & Tutorials(2023)
摘要
Malware poses a severe threat to cyber security. Attackers use malware to achieve their malicious purposes, such as unauthorized access, stealing confidential data, blackmailing, etc. Machine learning-based defense methods are applied to classify malware examples. However, such methods are vulnerable to adversarial attacks, where attackers aim to generate adversarial examples that can evade detection. Defenders also develop various approaches to enhance the robustness of malware classifiers against adversarial attacks. Both attackers and defenders evolve in the continuous confrontation of malware classification. In this paper, we firstly summarize a unified malware classification framework. Then, based on the framework, we systematically survey the Defense-Attack-Enhanced-Defense process and provide a comprehensive review of (i) machine learning-based malware classification, (ii) adversarial attacks on malware classifiers, and (iii) robust malware classification. Finally, we highlight the main challenges faced by both attackers and defenders and discuss some promising future work directions.
更多查看译文
关键词
Cyber security,malware,malware classification,adversarial examples,adversarial robustness
AI 理解论文
溯源树
样例
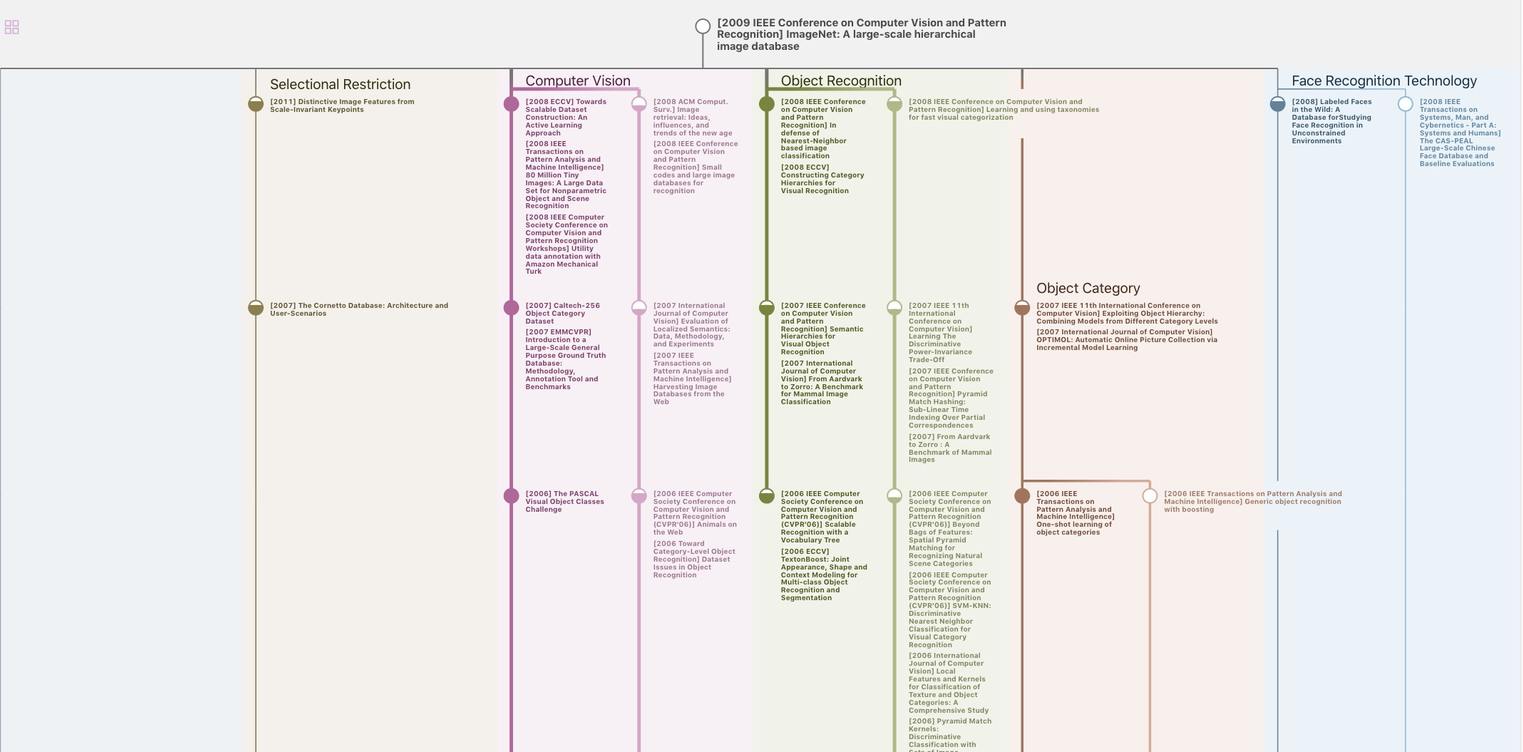
生成溯源树,研究论文发展脉络
Chat Paper
正在生成论文摘要