Performance Improvement of Machine Learning Model Using Autoencoder to Predict Demolition Waste Generation Rate
Sustainability(2023)
摘要
Owing to the rapid increase in construction and demolition (C&D) waste, the information of waste generation (WG) has been advantageously utilized as a strategy for C&D waste management. Recently, artificial intelligence (AI) has been strategically employed to obtain accurate WG information. Thus, this study aimed to manage demolition waste (DW) by combining three algorithms: artificial neural network (multilayer perceptron) (ANN-MLP), support vector regression (SVR), and random forest (RF) with an autoencoder (AE) to develop and test hybrid machine learning (ML) models. As a result of this study, AE technology significantly improved the performance of the ANN model. Especially, the performance of AE (25 features)-ANN model was superior to that of other non-hybrid and hybrid models. Compared to the non-hybrid ANN model, the performance of AE (25 features)-ANN model improved by 49%, 27%, 49%, and 22% in terms of the MAE, RMSE, R-2, and R, respectively. The hybrid model using ANN and AE proposed in this study showed useful results to improve the performance of the DWGR ML model. Therefore, this method is considered a novel and advantageous approach for developing a DWGR ML model. Furthermore, it can be used to develop AI models for improving performance in various fields.
更多查看译文
关键词
artificial intelligence,autoencoder,demolition waste,hybrid model,machine learning,waste management
AI 理解论文
溯源树
样例
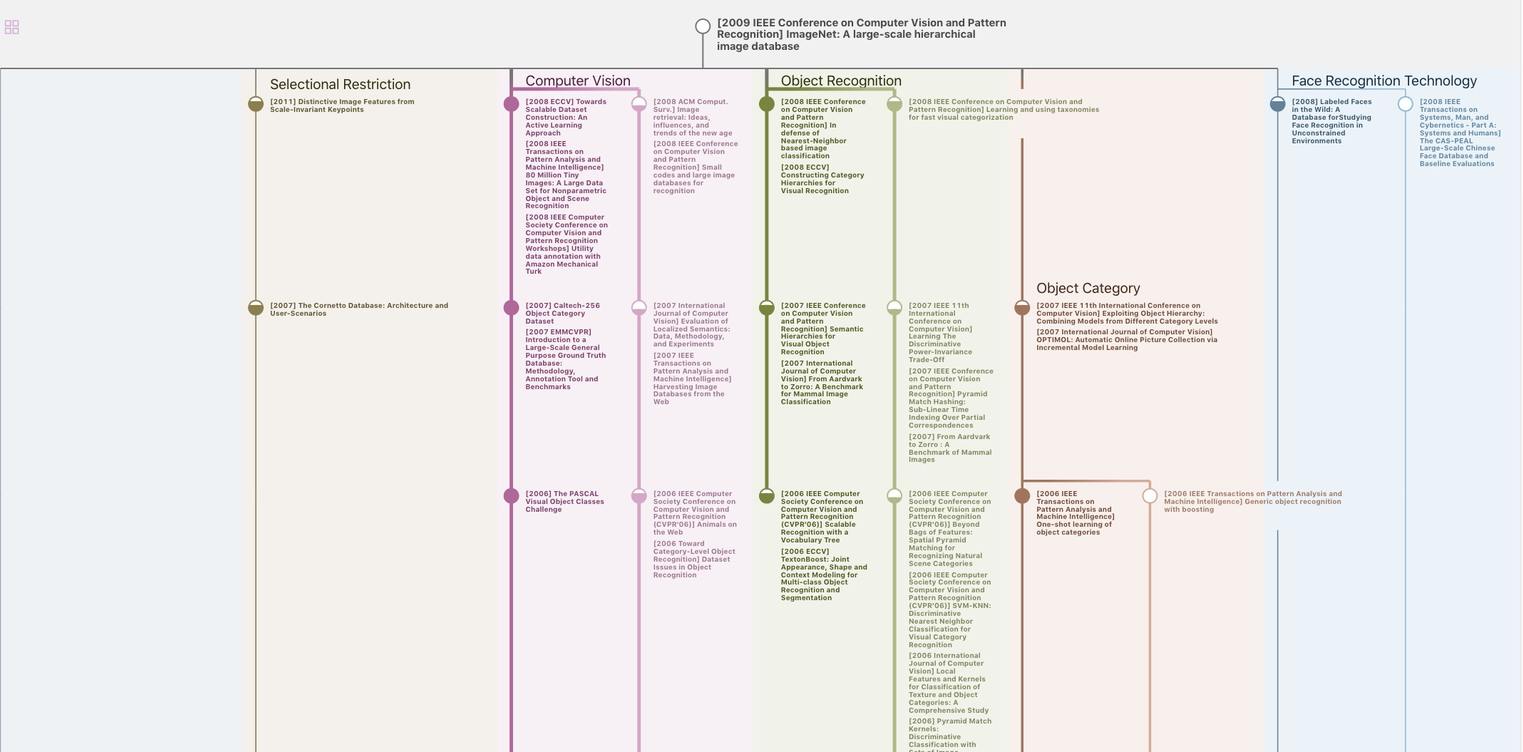
生成溯源树,研究论文发展脉络
Chat Paper
正在生成论文摘要