Nested Grassmannians for Dimensionality Reduction with Applications.
The journal of machine learning for biomedical imaging(2022)
摘要
In the recent past, nested structures in Riemannian manifolds has been studied in the context of dimensionality reduction as an alternative to the popular principal geodesic analysis (PGA) technique, for example, the principal nested spheres. In this paper, we propose a novel framework for constructing a nested sequence of homogeneous Riemannian manifolds. Common examples of homogeneous Riemannian manifolds include the n-sphere, the Stiefel manifold, the Grassmann manifold and many others. In particular, we focus on applying the proposed framework to the Grassmann manifold, giving rise to the nested Grassmannians (NG). An important application in which Grassmann manifolds are encountered is planar shape analysis. Specifically, each planar (2D) shape can be represented as a point in the complex projective space which is a complex Grassmann manifold. Some salient features of our framework are: (i) it explicitly exploits the geometry of the homogeneous Riemannian manifolds and (ii) the nested lower-dimensional submanifolds need not be geodesic. With the proposed NG structure, we develop algorithms for the supervised and unsupervised dimensionality reduction problems respectively. The proposed algorithms are compared with PGA via simulation studies and real data experiments and are shown to achieve a higher ratio of expressed variance compared to PGA.
更多查看译文
关键词
Dimensionality Reduction,Grassmann Manifolds,Homogeneous Riemannian Manifolds,Shape Analysis
AI 理解论文
溯源树
样例
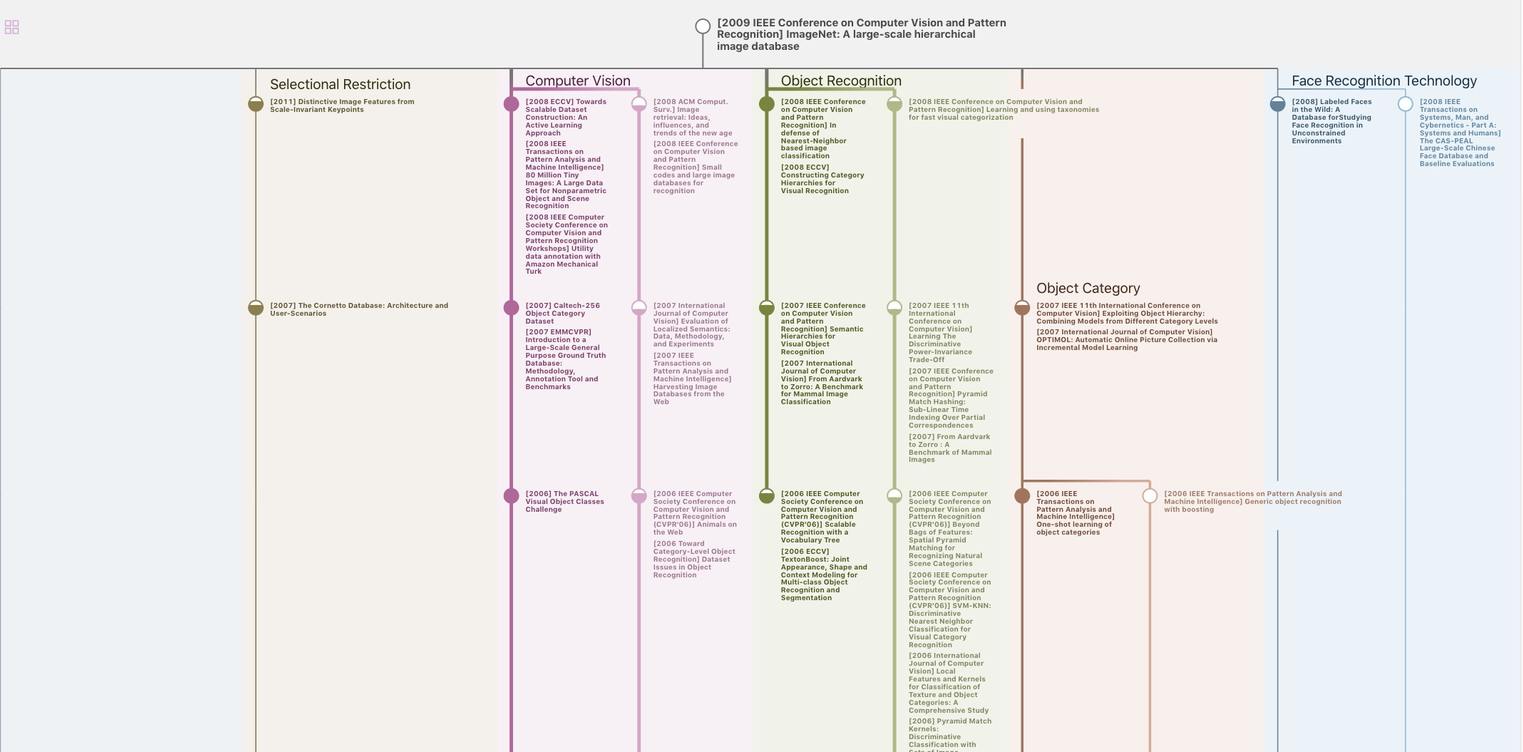
生成溯源树,研究论文发展脉络
Chat Paper
正在生成论文摘要