Connecting Vibrational Spectroscopy to Atomic Structure via Supervised Manifold Learning: Beyond Peak Analysis.
Chemistry of materials : a publication of the American Chemical Society(2023)
摘要
Vibrational spectroscopy is a nondestructive technique commonly used in chemical and physical analyses to determine atomic structures and associated properties. However, the evaluation and interpretation of spectroscopic profiles based on human-identifiable peaks can be difficult and convoluted. To address this challenge, we present a reliable protocol based on supervised manifold learning techniques meant to connect vibrational spectra to a variety of complex and diverse atomic structure configurations. As an illustration, we examined a large database of virtual vibrational spectroscopy profiles generated from atomistic simulations for silicon structures subjected to different stress, amorphization, and disordering states. We evaluated representative features in those spectra via various linear and nonlinear dimensionality reduction techniques and used the reduced representation of those features with decision trees to correlate them with structural information unavailable through classical human-identifiable peak analysis. We show that our trained model accurately (over 97% accuracy) and robustly (insensitive to noise) disentangles the contribution from the different material states, hence demonstrating a comprehensive decoding of spectroscopic profiles beyond classical (human-identifiable) peak analysis.
更多查看译文
关键词
vibrational spectroscopy,supervised manifold learning,atomic structure
AI 理解论文
溯源树
样例
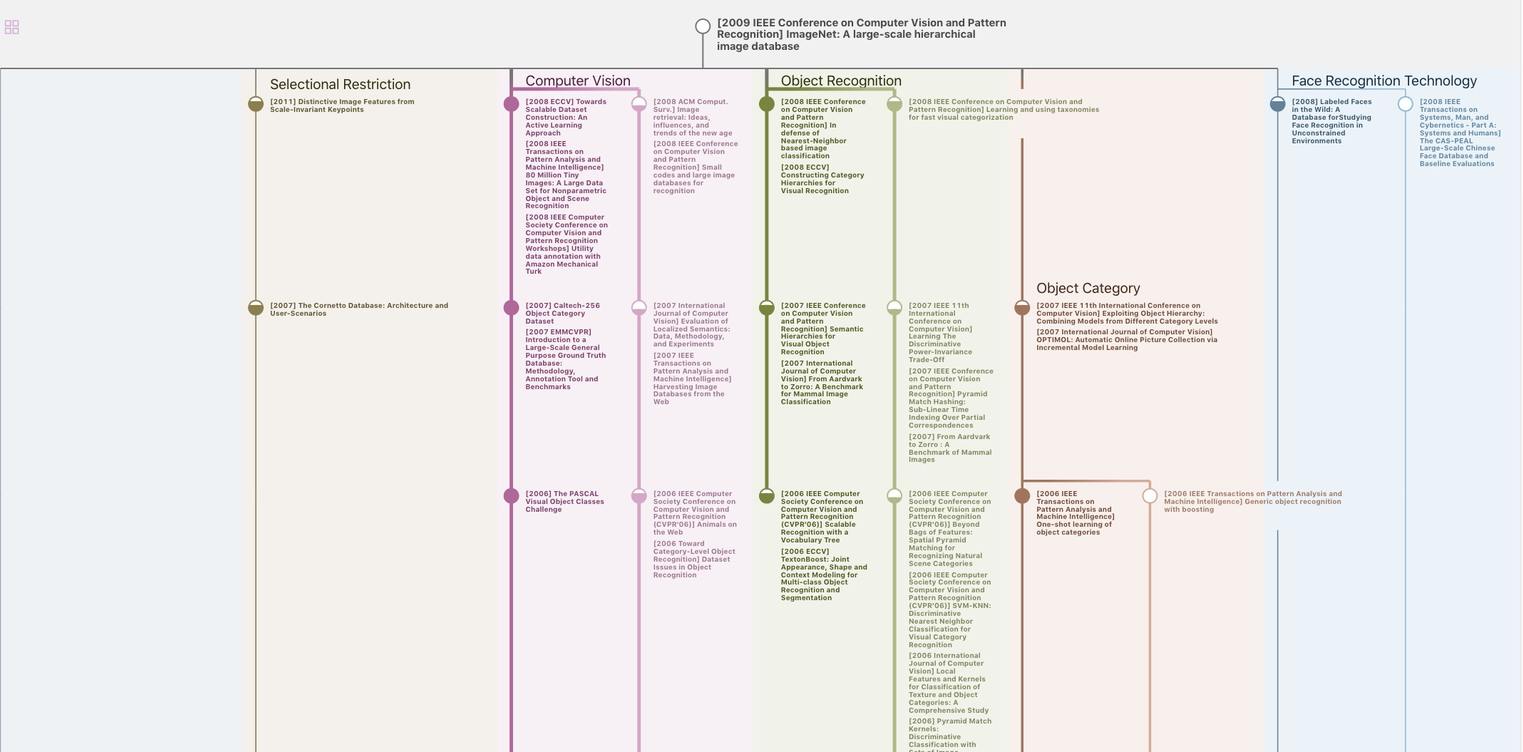
生成溯源树,研究论文发展脉络
Chat Paper
正在生成论文摘要