A parameter-optimization framework for neural decoding systems.
Frontiers in neuroinformatics(2023)
摘要
Real-time neuron detection and neural activity extraction are critical components of real-time neural decoding. They are modeled effectively in dataflow graphs. However, these graphs and the components within them in general have many parameters, including hyper-parameters associated with machine learning sub-systems. The dataflow graph parameters induce a complex design space, where alternative configurations (design points) provide different trade-offs involving key operational metrics including accuracy and time-efficiency. In this paper, we propose a novel optimization framework that automatically configures the parameters in different neural decoders. The proposed optimization framework is evaluated in depth through two case studies. Significant performance improvement in terms of accuracy and efficiency is observed in both case studies compared to the manual parameter optimization that was associated with the published results of those case studies. Additionally, we investigate the application of efficient multi-threading strategies to speed-up the running time of our parameter optimization framework. Our proposed optimization framework enables efficient and effective estimation of parameters, which leads to more powerful neural decoding capabilities and allows researchers to experiment more easily with alternative decoding models.
更多查看译文
关键词
data stream mining,dataflow,neural decoding,parameter optimization,real-time image processing
AI 理解论文
溯源树
样例
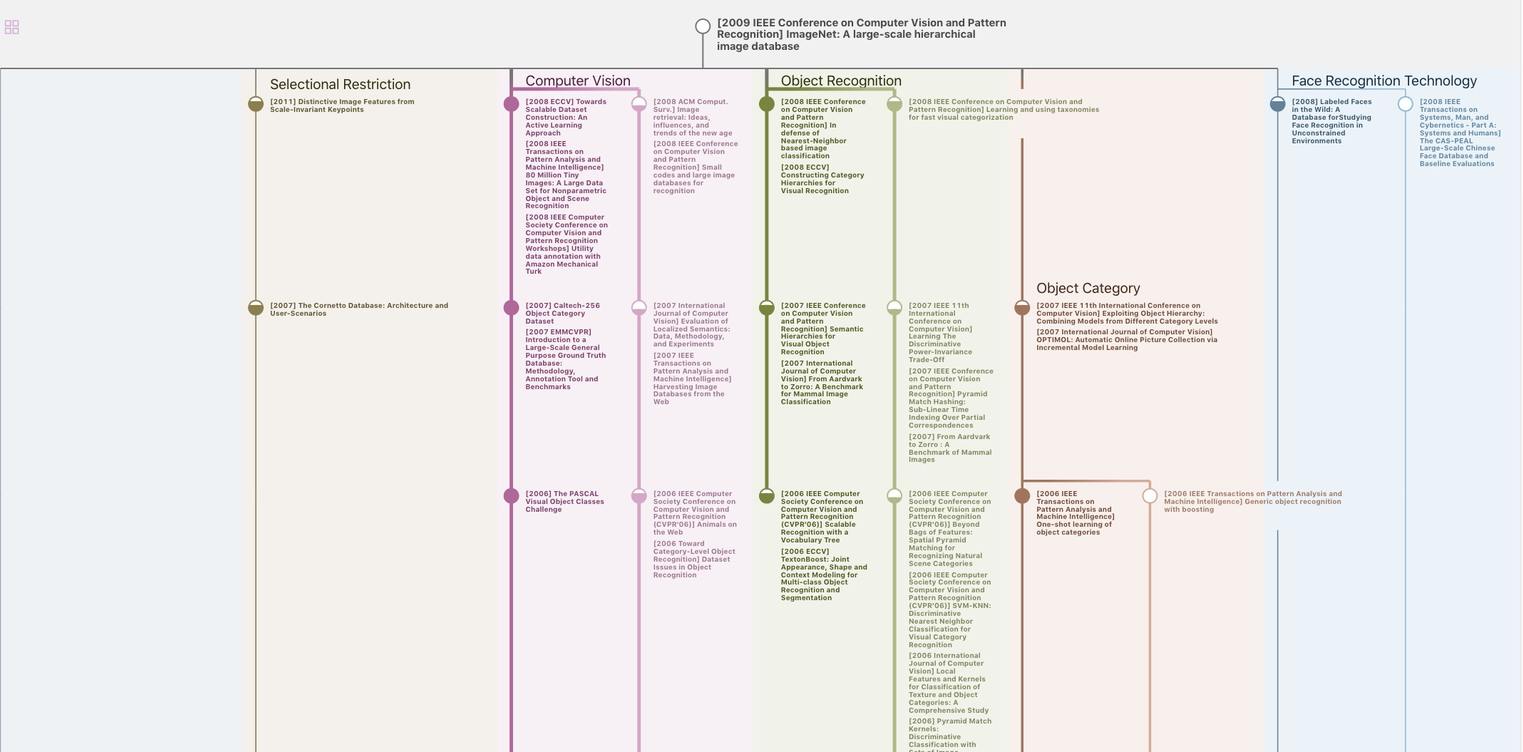
生成溯源树,研究论文发展脉络
Chat Paper
正在生成论文摘要