Investigating genes associated with heart failure, atrial fibrillation, and other cardiovascular diseases, and predicting disease using machine learning techniques for translational research and precision medicine.
Genomics(2023)
摘要
Cardiovascular disease (CVD) is the leading cause of mortality and loss of disability adjusted life years (DALYs) globally. CVDs like Heart Failure (HF) and Atrial Fibrillation (AF) are associated with physical effects on the heart muscles. As a result of the complex nature, progression, inherent genetic makeup, and heterogeneity of CVDs, personalized treatments are believed to be critical. Rightful application of artificial intelligence (AI) and machine learning (ML) approaches can lead to new insights into CVDs for providing better personalized treatments with predictive analysis and deep phenotyping. In this study we focused on implementing AI/ML techniques on RNA-seq driven gene-expression data to investigate genes associated with HF, AF, and other CVDs, and predict disease with high accuracy. The study involved generating RNA-seq data derived from the serum of consented CVD patients. Next, we processed the sequenced data using our RNA-seq pipeline and applied GVViZ for gene-disease data annotation and expression analysis. To achieve our research objectives, we developed a new Findable, Accessible, Intelligent, and Reproducible (FAIR) approach that includes a five-level biostatistical evaluation, primarily based on the Random Forest (RF) algorithm. During our AI/ML analysis, we have fitted, trained, and implemented our model to classify and distinguish high-risk CVD patients based on their age, gender, and race. With the successful execution of our model, we predicted the association of highly significant HF, AF, and other CVDs genes with demographic variables.
更多查看译文
关键词
Artificial intelligence,Atrial fibrillation,Cardiovascular diseases,Gene expression,Heart failure,Machine learning,Predictive analysis
AI 理解论文
溯源树
样例
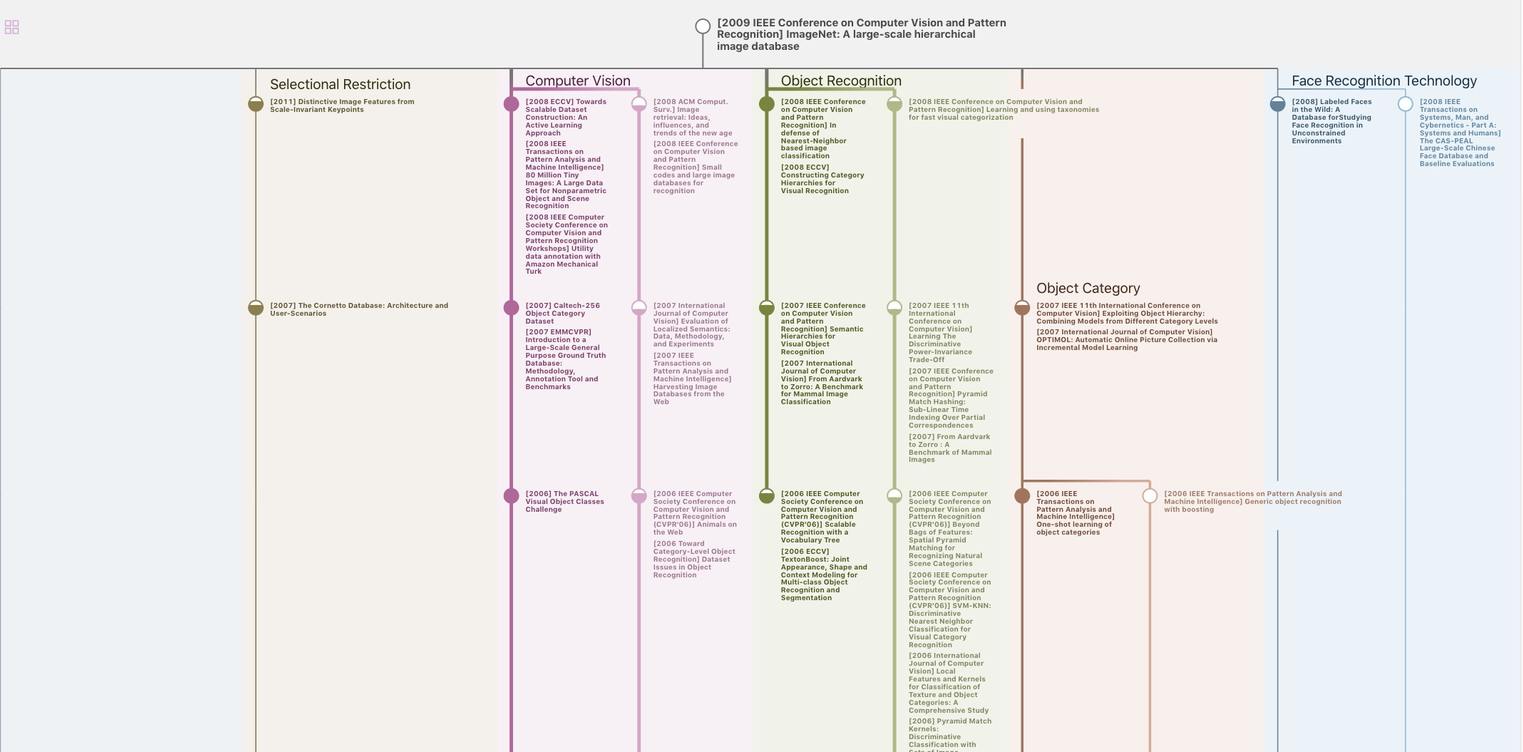
生成溯源树,研究论文发展脉络
Chat Paper
正在生成论文摘要