Bayesian Structure Scores for Probabilistic Circuits
arxiv(2023)
摘要
Probabilistic circuits (PCs) are a prominent representation of probability distributions with tractable inference. While parameter learning in PCs is rigorously studied, structure learning is often more based on heuristics than on principled objectives. In this paper, we develop Bayesian structure scores for deterministic PCs, i.e., the structure likelihood with parameters marginalized out, which are well known as rigorous objectives for structure learning in probabilistic graphical models. When used within a greedy cutset algorithm, our scores effectively protect against overfitting and yield a fast and almost hyper-parameter-free structure learner, distinguishing it from previous approaches. In experiments, we achieve good trade-offs between training time and model fit in terms of log-likelihood. Moreover, the principled nature of Bayesian scores unlocks PCs for accommodating frameworks such as structural expectation-maximization.
更多查看译文
关键词
probabilistic circuits,structure
AI 理解论文
溯源树
样例
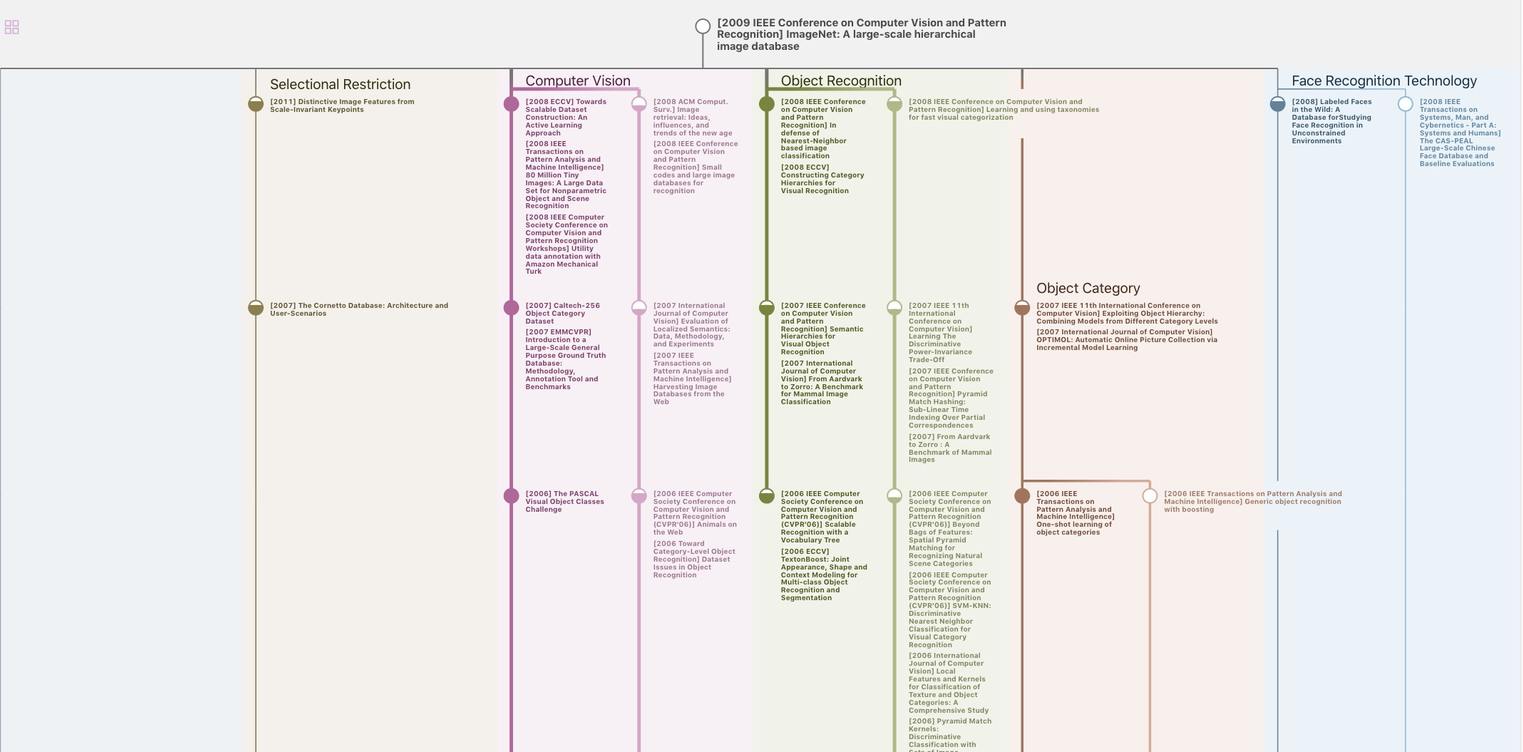
生成溯源树,研究论文发展脉络
Chat Paper
正在生成论文摘要