Solving Recurrent MIPs with Semi-supervised Graph Neural Networks
arxiv(2023)
摘要
We propose an ML-based model that automates and expedites the solution of MIPs by predicting the values of variables. Our approach is motivated by the observation that many problem instances share salient features and solution structures since they differ only in few (time-varying) parameters. Examples include transportation and routing problems where decisions need to be re-optimized whenever commodity volumes or link costs change. Our method is the first to exploit the sequential nature of the instances being solved periodically, and can be trained with ``unlabeled'' instances, when exact solutions are unavailable, in a semi-supervised setting. Also, we provide a principled way of transforming the probabilistic predictions into integral solutions. Using a battery of experiments with representative binary MIPs, we show the gains of our model over other ML-based optimization approaches.
更多查看译文
关键词
recurrent mips,neural networks,semi-supervised
AI 理解论文
溯源树
样例
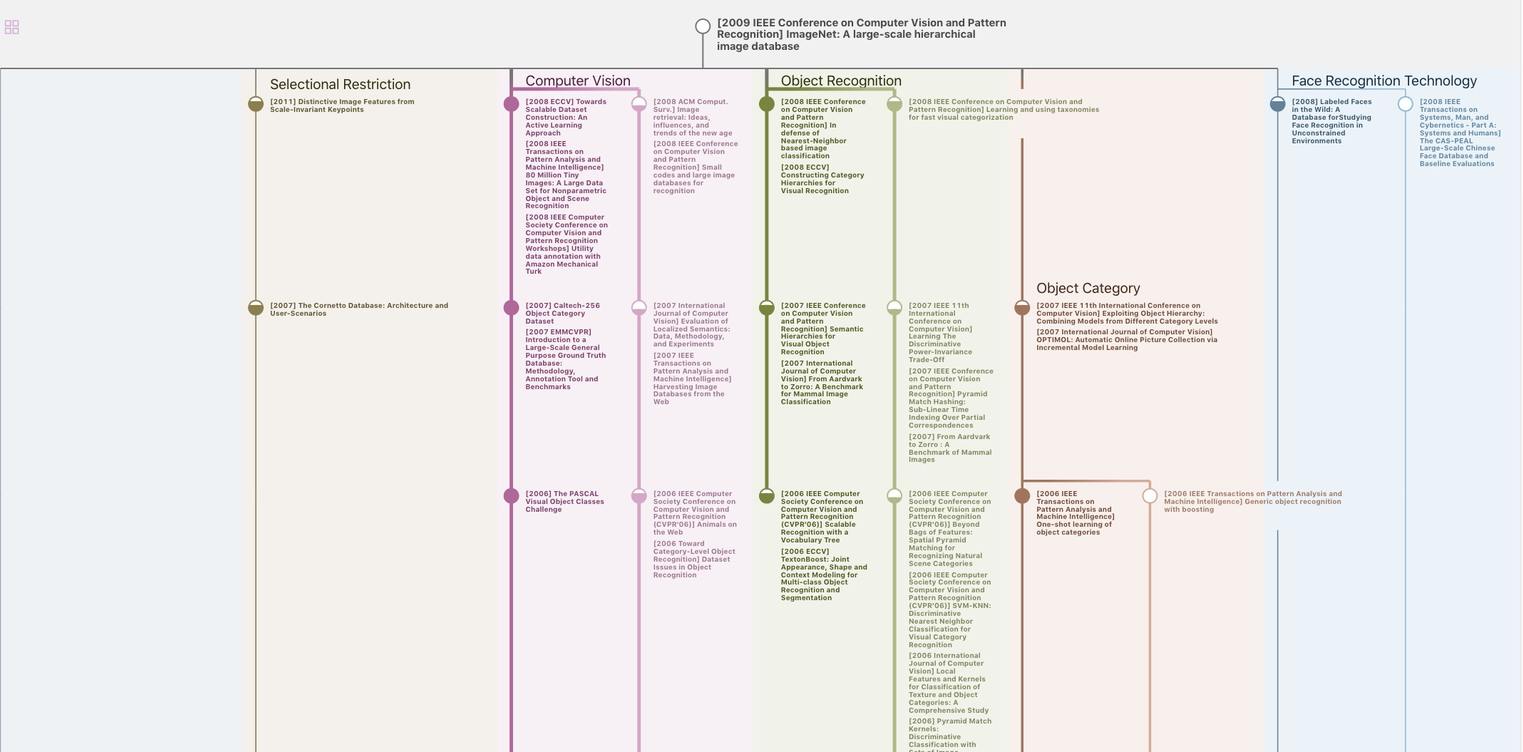
生成溯源树,研究论文发展脉络
Chat Paper
正在生成论文摘要