Mitigating Unnecessary Handovers in Ultra-Dense Networks through Machine Learning-based Mobility Prediction
arxiv(2023)
摘要
In 5G wireless communication, Intelligent Transportation Systems (ITS) and automobile applications, such as autonomous driving, are widely examined. These applications have strict requirements and often require high Quality of Service (QoS). In an urban setting, Ultra-Dense Networks (UDNs) have the potential to not only provide optimal QoS but also increase system capacity and frequency reuse. However, the current architecture of 5G UDN of dense Small Cell Nodes (SCNs) deployment prompts increased delay, handover times, and handover failures. In this paper, we propose a Machine Learning (ML) supported Mobility Prediction (MP) strategy to predict future Vehicle User Equipment (VUE) mobility and handover locations. The primary aim of the proposed methodology is to minimize Unnecessary Handover (UHO) to ensure reliable communication in vehicular communication systems. We evaluate and validate our approach on a downlink system-level simulator. We predict mobility using Support Vector Machine (SVM), Decision Tree Classifier (DTC), and Random Forest Classifier (RFC). Simulation results demonstrate a notable 30% decrease in handover times and a considerable enhancement in QoS, maintaining the advantage of 5G UDN networks.
更多查看译文
关键词
5G UDN,Mobility Prediction,Machine Learning,Handover Reduction
AI 理解论文
溯源树
样例
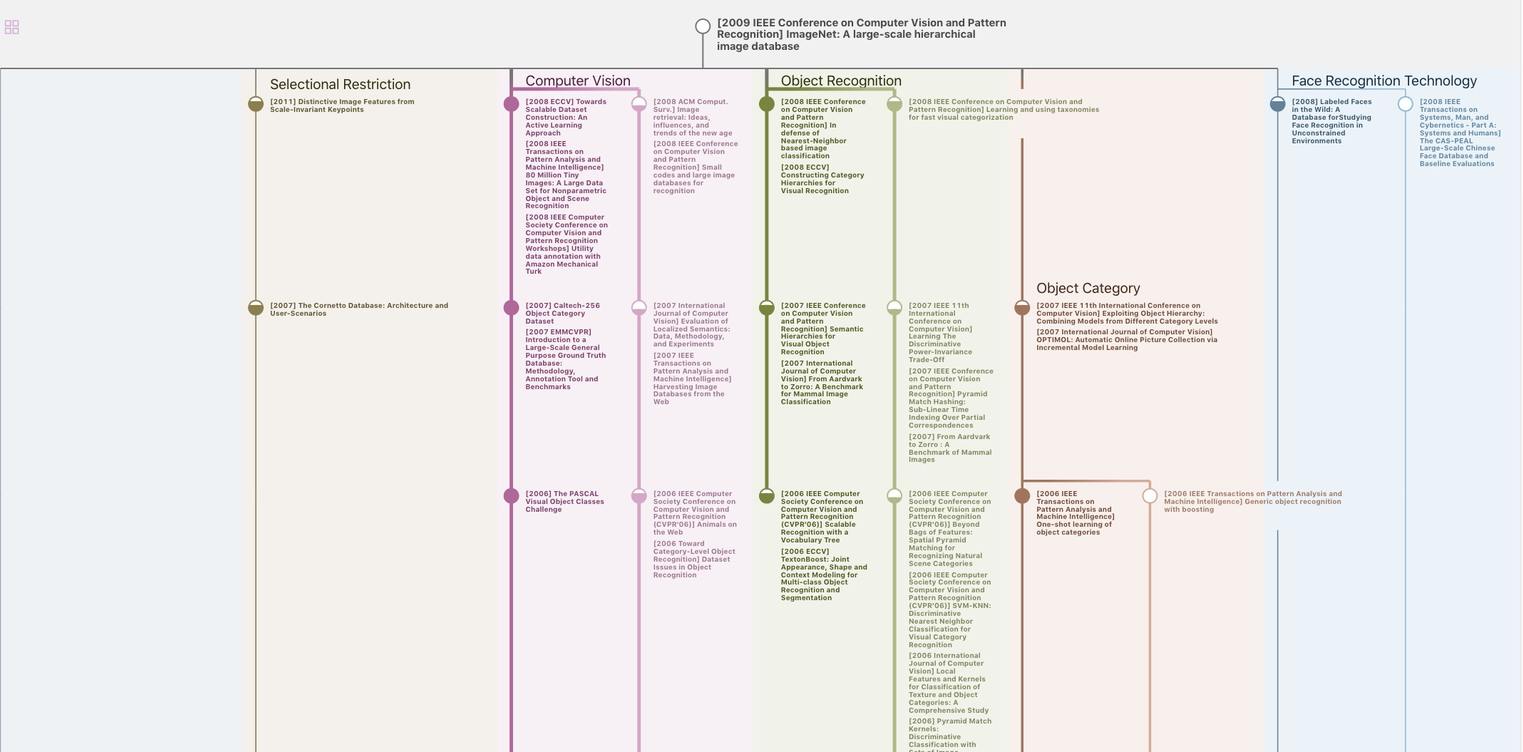
生成溯源树,研究论文发展脉络
Chat Paper
正在生成论文摘要