Improving the generalization via coupled tensor norm regularization
arXiv (Cornell University)(2023)
摘要
In this paper, we propose a coupled tensor norm regularization that could enable the model output feature and the data input to lie in a low-dimensional manifold, which helps us to reduce overfitting. We show this regularization term is convex, differentiable, and gradient Lipschitz continuous for logistic regression, while nonconvex and nonsmooth for deep neural networks. We further analyze the convergence of the first-order method for solving this model. The numerical experiments demonstrate that our method is efficient.
更多查看译文
关键词
generalization,norm
AI 理解论文
溯源树
样例
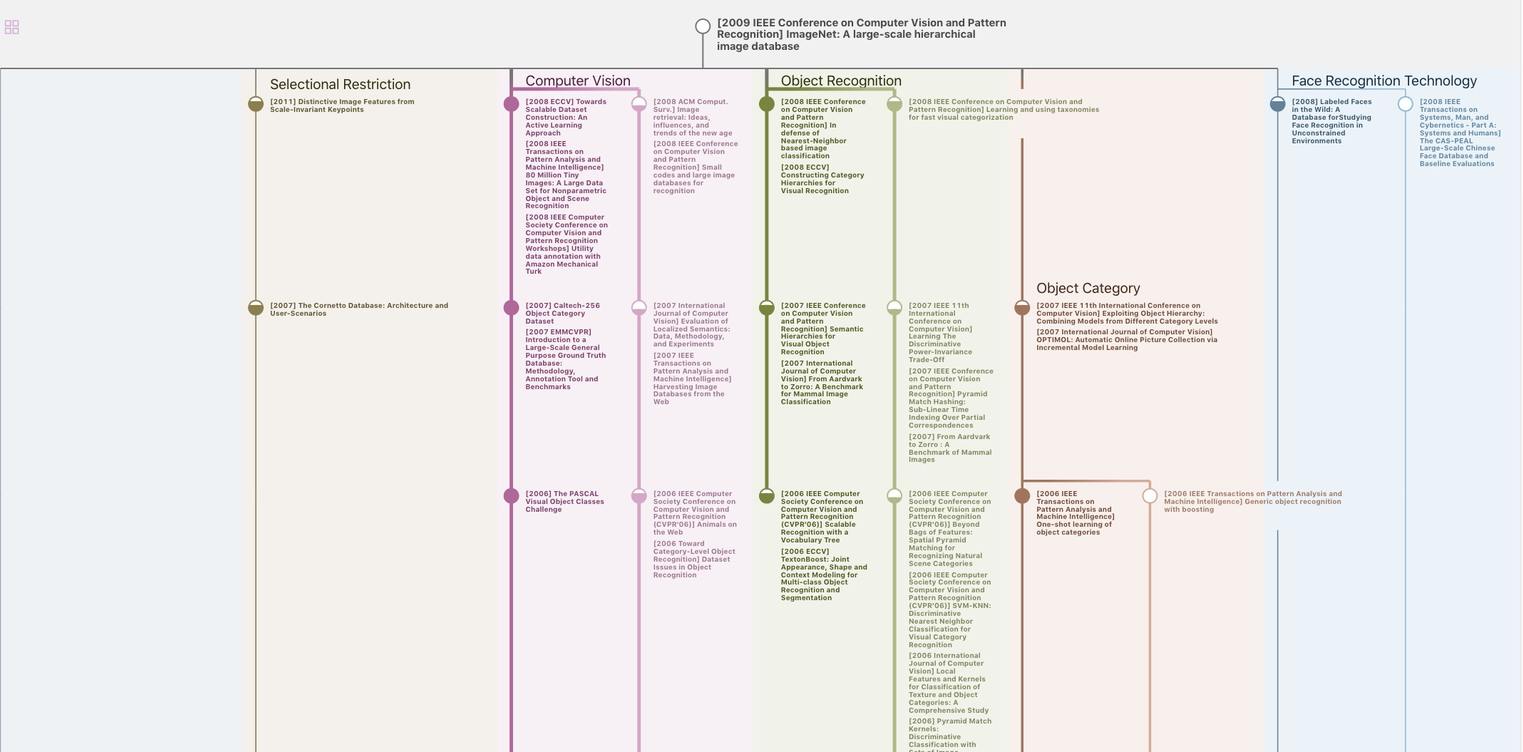
生成溯源树,研究论文发展脉络
Chat Paper
正在生成论文摘要