Forecasting SDN End-to-End Latency Using Graph Neural Network
2023 International Conference on Information Networking (ICOIN)(2023)
摘要
Accurately predicting end-to-end delay is a challenging but essential problem in the networking field. By improving the delay estimation accuracy, we can distribute traffic more efficiently to enhance the networking performance future, such as satisfying packet latency requirements and improving user experience. This paper aims to predict an end-to-end delay in Software Defined Networking (SDN). We use a GNN-based model named Spatial-Temporal Graph Convolutional Network (STGCN). Benefiting the ability to capture spatial and temporal dependencies of traffic data, STGCN outperforms the baseline methodology and other three machine learning techniques, Multiple Linear Regression (MLR), Extreme Gradient Boosting (XGBOOST) and Random Forest (RF). Notably, our GNN-based methodology can achieve 97.0%, 95.9%, 96.1%, and 63.1% less root mean square error (RMSE) than the baseline predictor, MLR, XGBOOST and RF, respectively.
更多查看译文
关键词
GNN,SDN,End-to-end Delay Estimation
AI 理解论文
溯源树
样例
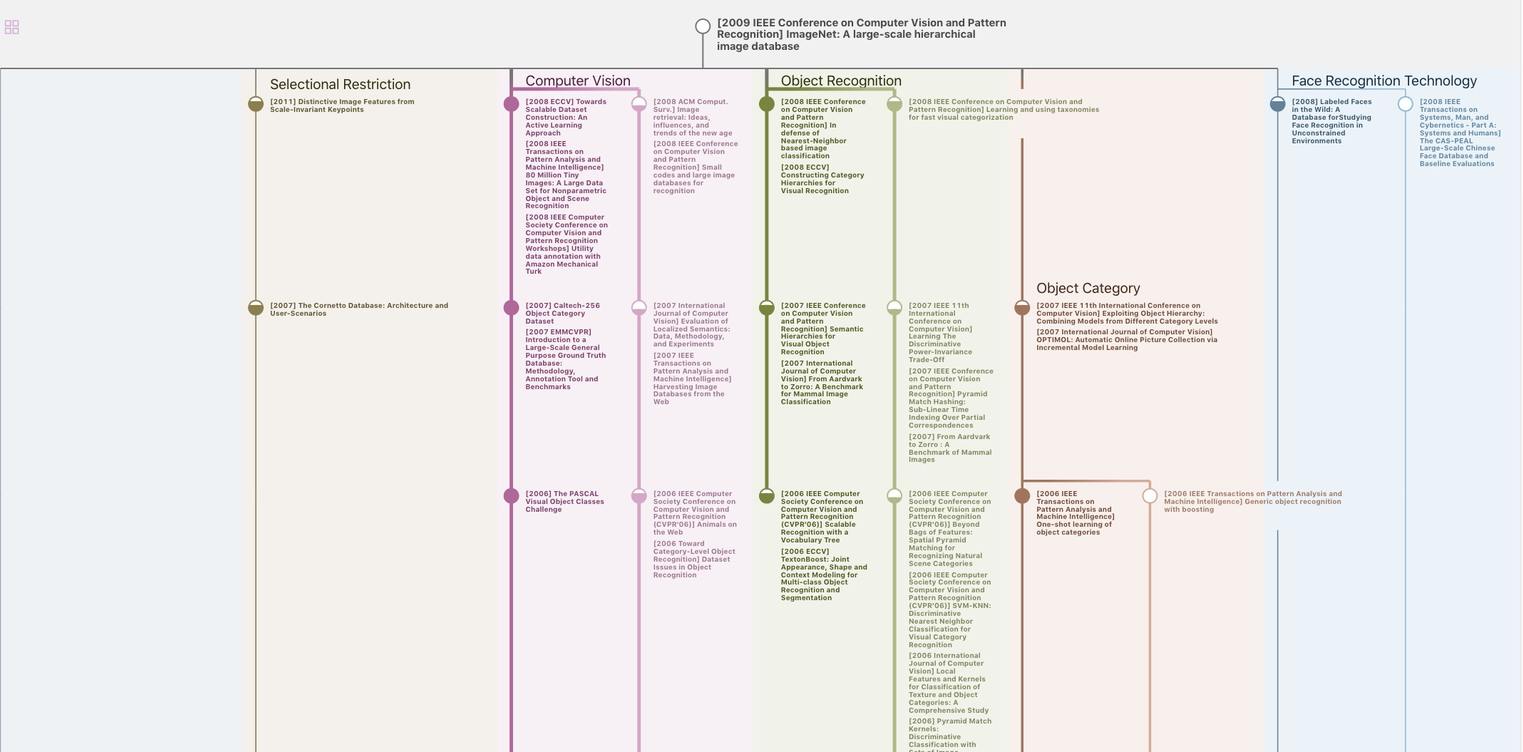
生成溯源树,研究论文发展脉络
Chat Paper
正在生成论文摘要