Group Counselling Optimization-derived Software Bug Prediction Framework by Optimal Feature Selection-based Dimensionality Reduction
2023 13th International Conference on Cloud Computing, Data Science & Engineering (Confluence)(2023)
摘要
Recently, Software Bug Prediction (SBP) has become a serious issue in maintaining as well as developing the software that concerns the overall success of the software. In this case, the prediction of the bug in the software in its earlier phase may enhance the efficiency, quality, and reliability of the software as well as reduce the cost. Moreover, designing a robust software bug prediction model has become a challenging issue as well as several techniques have been recommended in the literature. Initially, the data from the relevant dataset is gathered. Then, the gathered data is fed to the pre-processing stage. The pre-processed data is fed to the optimal feature selection phase, in which the features are optimized with the aid of the Group Counseling Optimization (GCO) algorithm. Then, the Improved Long Short Term Memory (ILSTM) technique is used for prediction and the parameters in LSTM are also optimized with the same GCO algorithm. Then, the bug- predicted outcomes are attained by comparing the model with other conventional techniques including Principal Component Analysis (PCA), Linear Discriminant Analysis (LDA), Kernel-based Support Vector Machine (SVM), etc.
更多查看译文
关键词
Software Bug Prediction,Optimal Feature Selection,Dimensionality Reduction,Group Counseling Optimization,Long Short Term Memory,Linear Discriminant Analysis,Support Vector Machine,principal component analysis
AI 理解论文
溯源树
样例
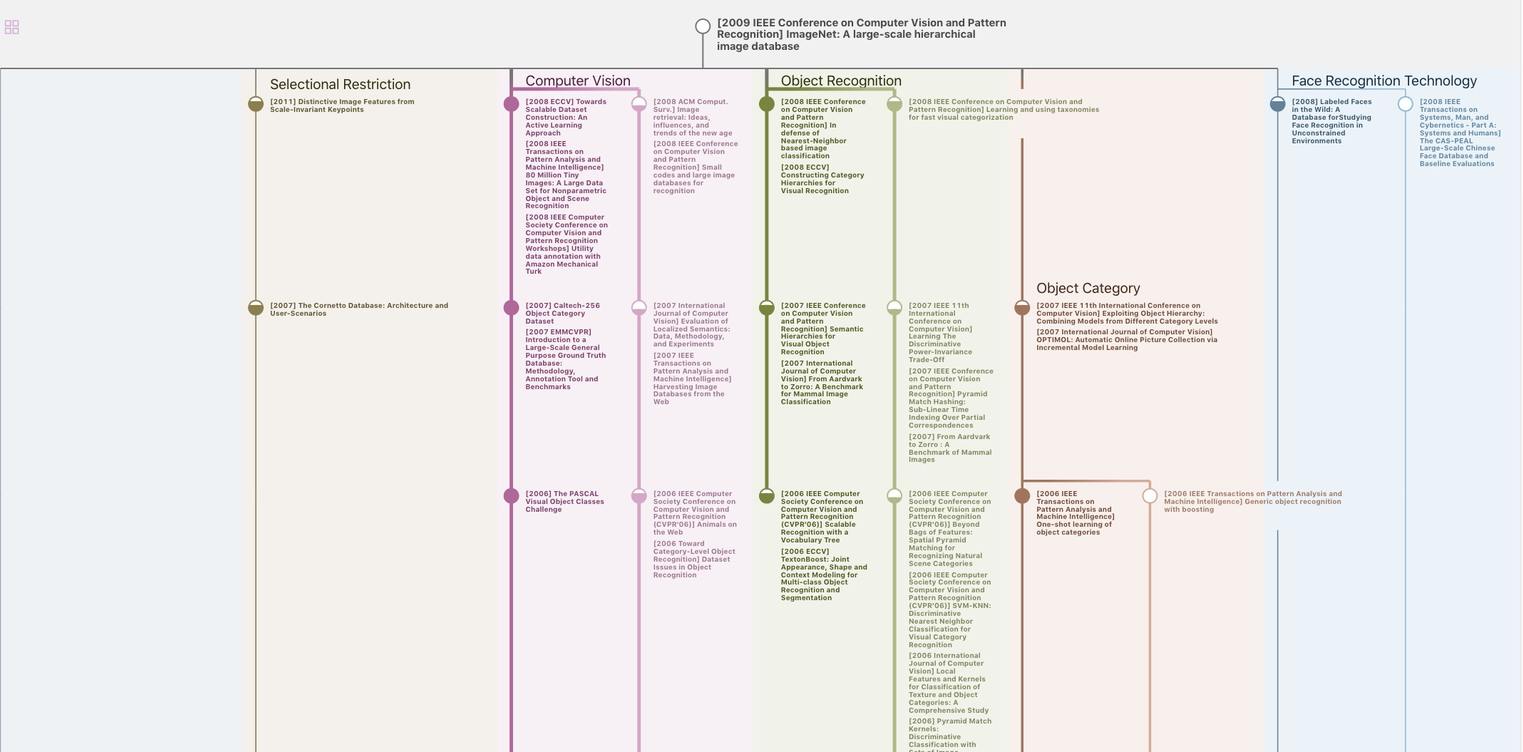
生成溯源树,研究论文发展脉络
Chat Paper
正在生成论文摘要