Position-Based Machine Learning Propagation Loss Model Enabling Fast Digital Twins of Wireless Networks in ns-3
arxiv(2023)
摘要
Digital twins have been emerging as a hybrid approach that combines the benefits of simulators with the realism of experimental testbeds. The accurate and repeatable set-ups replicating the dynamic conditions of physical environments, enable digital twins of wireless networks to be used to evaluate the performance of next-generation networks. In this paper, we propose the Position-based Machine Learning Propagation Loss Model (P-MLPL), enabling the creation of fast and more precise digital twins of wireless networks in ns-3. Based on network traces collected in an experimental testbed, the P-MLPL model estimates the propagation loss suffered by packets exchanged between a transmitter and a receiver, considering the absolute node's positions and the traffic direction. The P-MLPL model is validated with a test suite. The results show that the P-MLPL model can predict the propagation loss with a median error of 2.5 dB, which corresponds to 0.5x the error of existing models in ns-3. Moreover, ns-3 simulations with the P-MLPL model estimated the throughput with an error up to 2.5 Mbit/s, when compared to the real values measured in the testbed.
更多查看译文
关键词
Propagation loss model, Machine learning, Digital twins, Wireless networks, ns-3
AI 理解论文
溯源树
样例
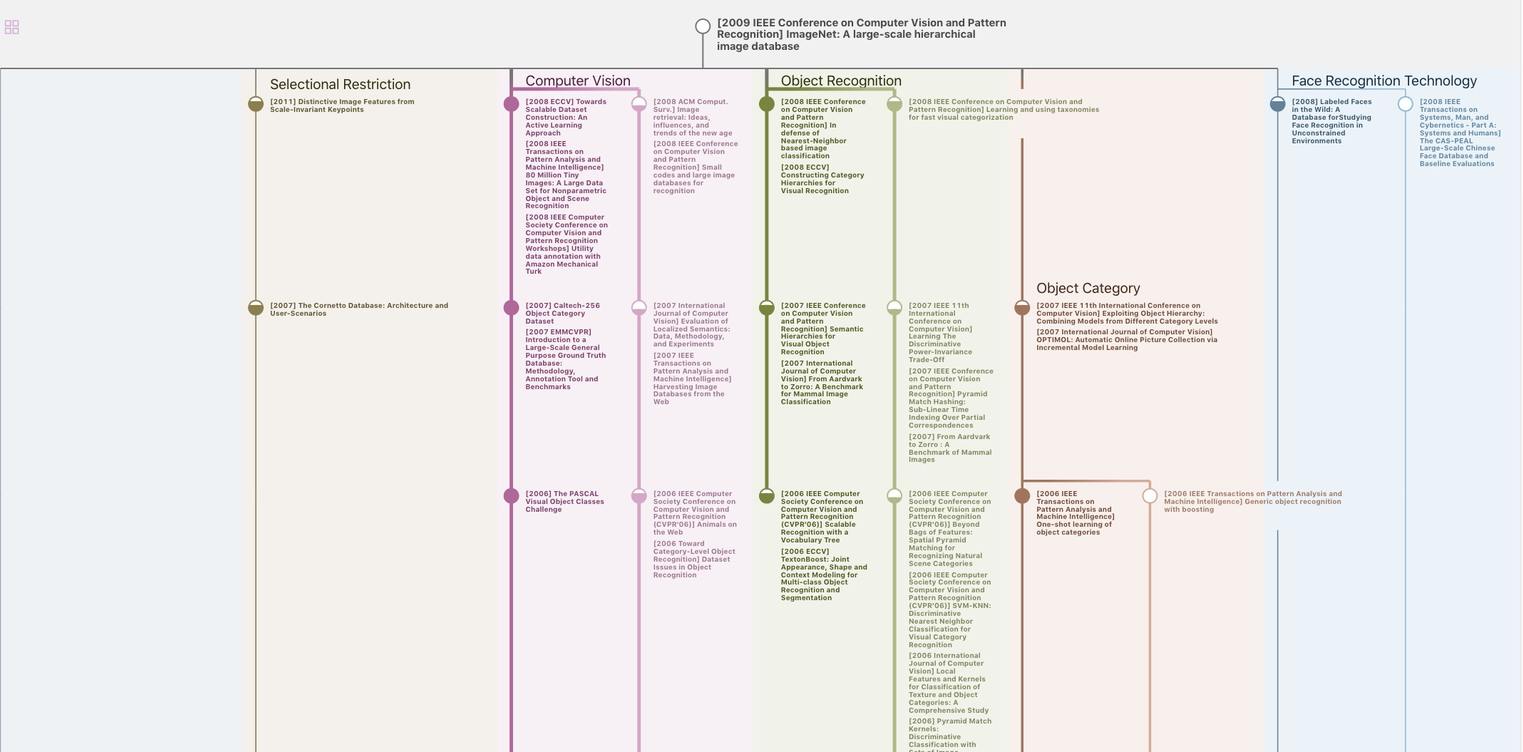
生成溯源树,研究论文发展脉络
Chat Paper
正在生成论文摘要