Distilling Calibrated Student from an Uncalibrated Teacher
arxiv(2023)
摘要
Knowledge distillation is a common technique for improving the performance of a shallow student network by transferring information from a teacher network, which in general, is comparatively large and deep. These teacher networks are pre-trained and often uncalibrated, as no calibration technique is applied to the teacher model while training. Calibration of a network measures the probability of correctness for any of its predictions, which is critical in high-risk domains. In this paper, we study how to obtain a calibrated student from an uncalibrated teacher. Our approach relies on the fusion of the data-augmentation techniques, including but not limited to cutout, mixup, and CutMix, with knowledge distillation. We extend our approach beyond traditional knowledge distillation and find it suitable for Relational Knowledge Distillation and Contrastive Representation Distillation as well. The novelty of the work is that it provides a framework to distill a calibrated student from an uncalibrated teacher model without compromising the accuracy of the distilled student. We perform extensive experiments to validate our approach on various datasets, including CIFAR-10, CIFAR-100, CINIC-10 and TinyImageNet, and obtained calibrated student models. We also observe robust performance of our approach while evaluating it on corrupted CIFAR-100C data.
更多查看译文
关键词
calibrated student,teacher
AI 理解论文
溯源树
样例
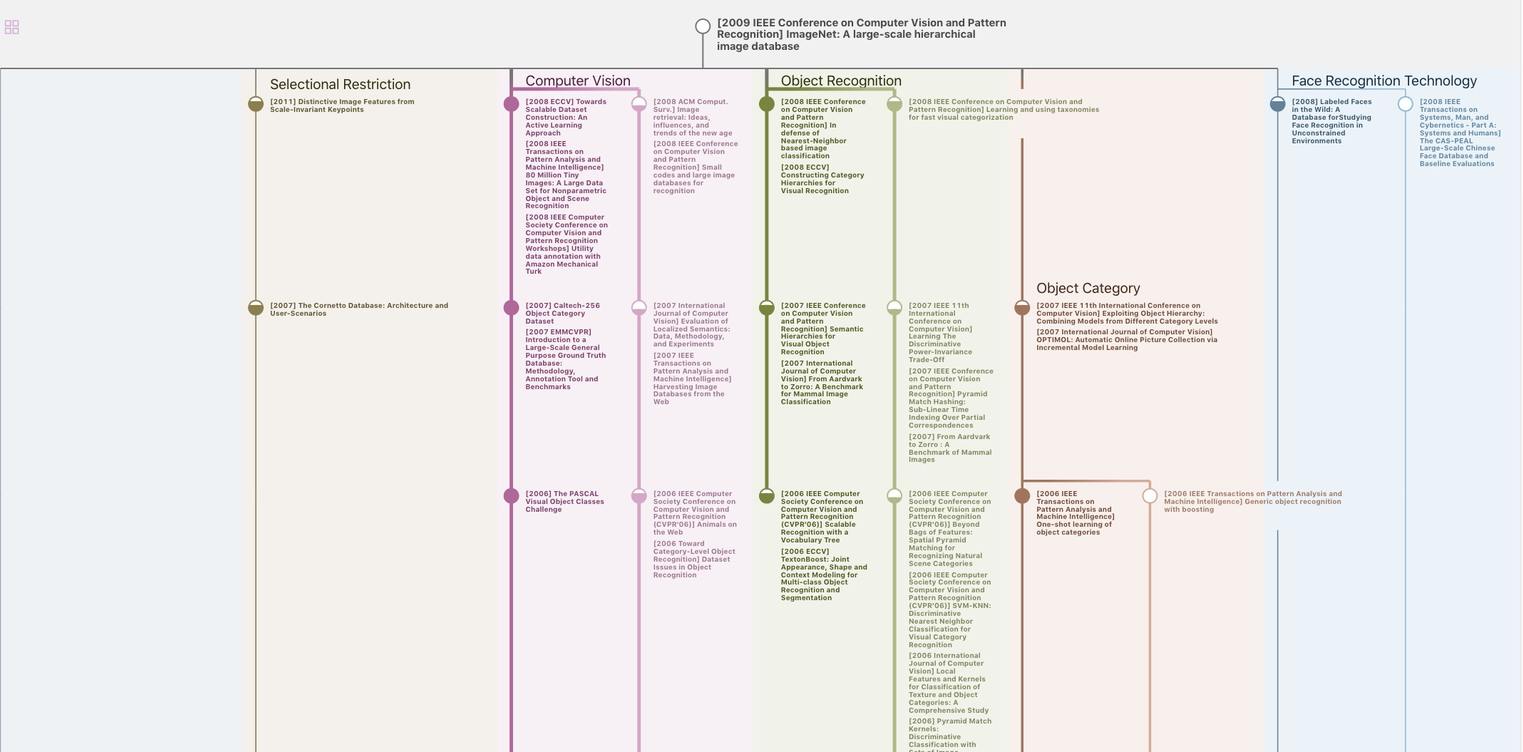
生成溯源树,研究论文发展脉络
Chat Paper
正在生成论文摘要