Probabilistic Simplex Component Analysis by Importance Sampling
arxiv(2023)
摘要
In this paper we consider the problem of linear unmixing hidden random variables defined over the simplex with additive Gaussian noise, also known as probabilistic simplex component analysis (PRISM). Previous solutions to tackle this challenging problem were based on geometrical approaches or computationally intensive variational methods. In contrast, we propose a conventional expectation maximization (EM) algorithm which embeds importance sampling. For this purpose, the proposal distribution is chosen as a simple surrogate distribution of the target posterior that is guaranteed to lie in the simplex. This distribution is based on the Gaussian linear minimum mean squared error (LMMSE) approximation which is accurate at high signal-to-noise ratio. Numerical experiments in different settings demonstrate the advantages of this adaptive surrogate over state-of-the-art methods.
更多查看译文
关键词
sampling,component,importance
AI 理解论文
溯源树
样例
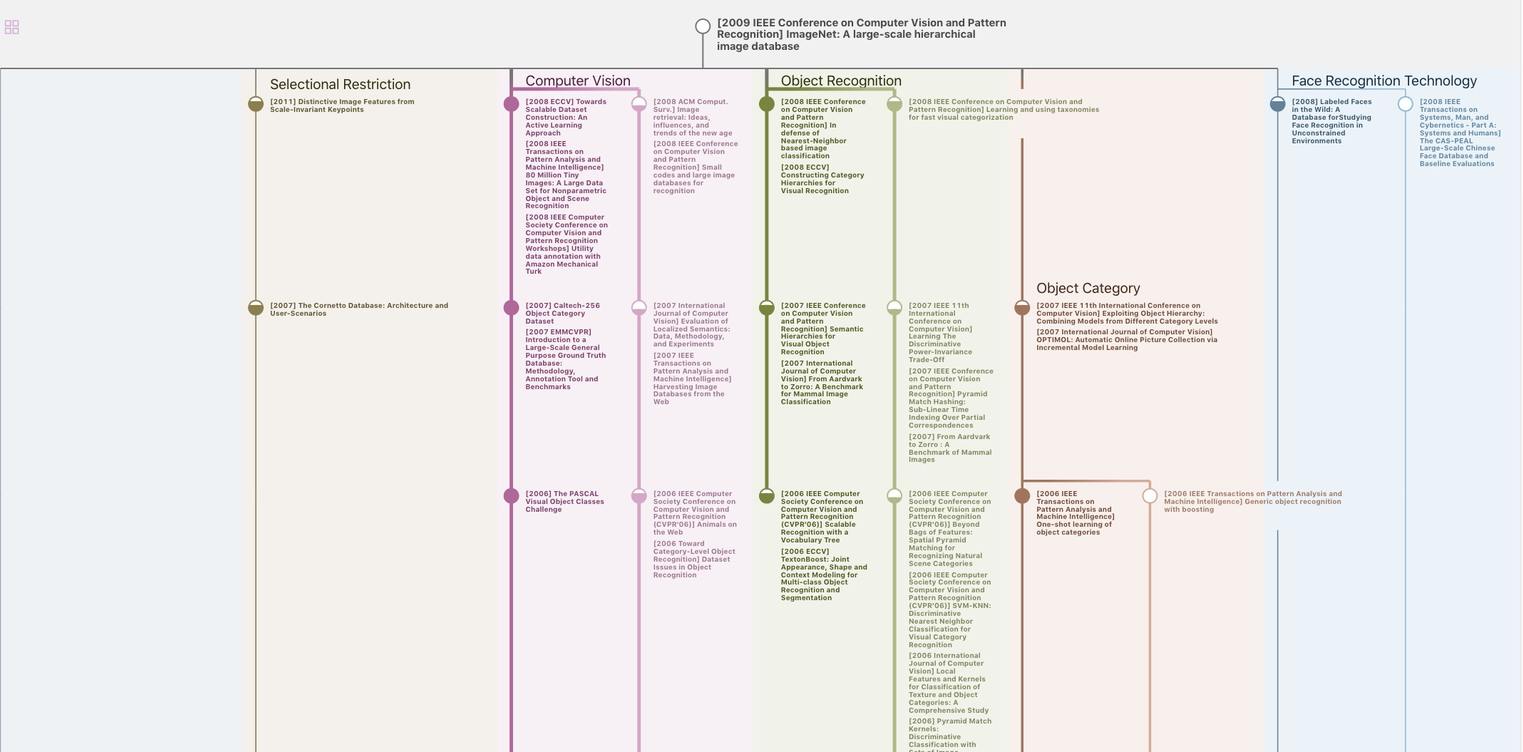
生成溯源树,研究论文发展脉络
Chat Paper
正在生成论文摘要