Universal Morphology Control via Contextual Modulation
arXiv (Cornell University)(2023)
Abstract
Learning a universal policy across different robot morphologies can significantly improve learning efficiency and generalization in continuous control. However, it poses a challenging multi-task reinforcement learning problem, as the optimal policy may be quite different across robots and critically depend on the morphology. Existing methods utilize graph neural networks or transformers to handle heterogeneous state and action spaces across different morphologies, but pay little attention to the dependency of a robot's control policy on its morphology context. In this paper, we propose a hierarchical architecture to better model this dependency via contextual modulation, which includes two key submodules: (1) Instead of enforcing hard parameter sharing across robots, we use hypernetworks to generate morphology-dependent control parameters; (2) We propose a morphology-dependent attention mechanism to modulate the interactions between different limbs in a robot. Experimental results show that our method not only improves learning performance on a diverse set of training robots, but also generalizes better to unseen morphologies in a zero-shot fashion.
MoreTranslated text
Key words
morphology,modulation,universal,control
AI Read Science
Must-Reading Tree
Example
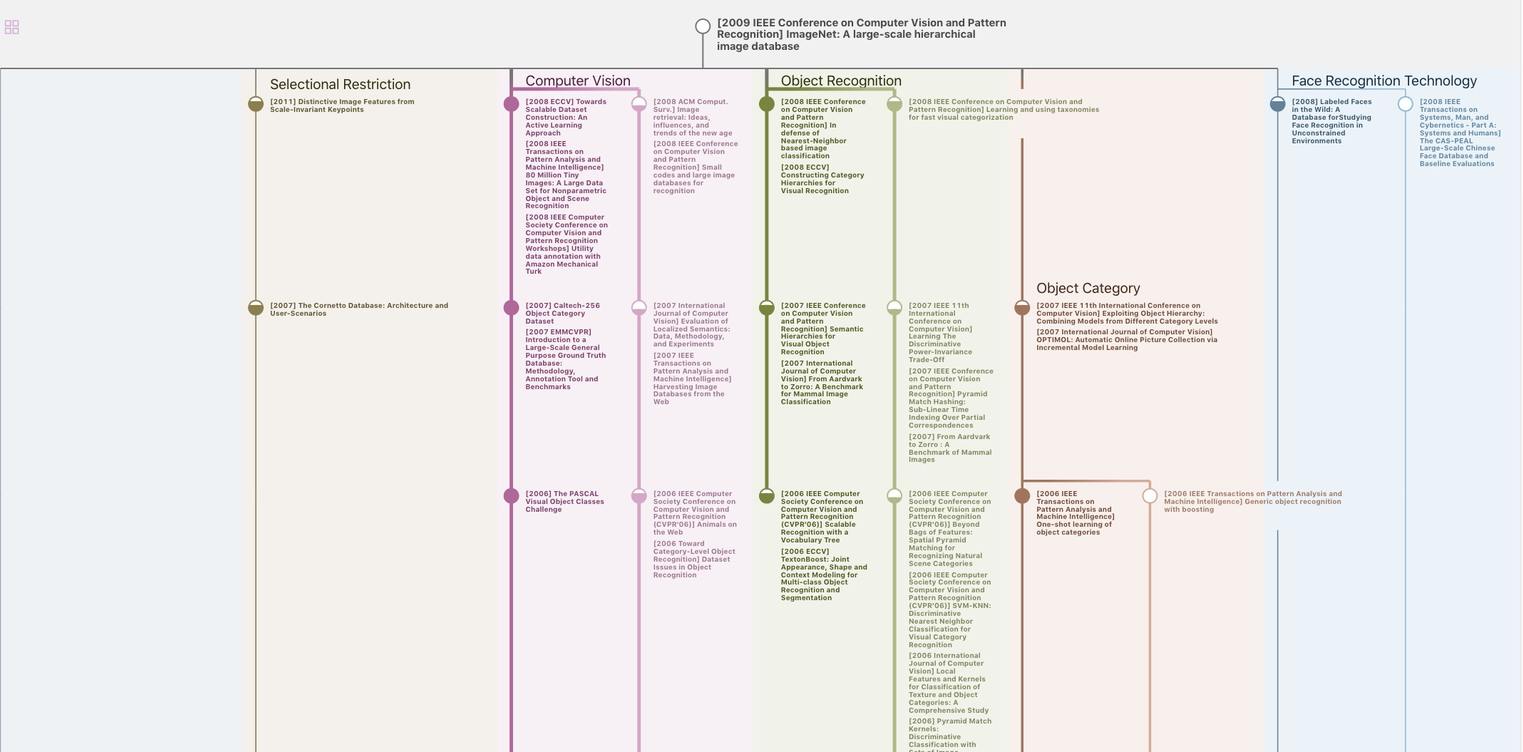
Generate MRT to find the research sequence of this paper
Chat Paper
Summary is being generated by the instructions you defined