Learning signatures of decision making from many individuals playing the same game
arxiv(2023)
摘要
Human behavior is incredibly complex and the factors that drive decision making-from instinct, to strategy, to biases between individuals-often vary over multiple timescales. In this paper, we design a predictive framework that learns representations to encode an individual's 'behavioral style', i.e. long-term behavioral trends, while simultaneously predicting future actions and choices. The model explicitly separates representations into three latent spaces: the recent past space, the short-term space, and the long-term space where we hope to capture individual differences. To simultaneously extract both global and local variables from complex human behavior, our method combines a multi-scale temporal convolutional network with latent prediction tasks, where we encourage embeddings across the entire sequence, as well as subsets of the sequence, to be mapped to similar points in the latent space. We develop and apply our method to a large-scale behavioral dataset from 1,000 humans playing a 3-armed bandit task, and analyze what our model's resulting embeddings reveal about the human decision making process. In addition to predicting future choices, we show that our model can learn rich representations of human behavior over multiple timescales and provide signatures of differences in individuals.
更多查看译文
关键词
Human decision making,self-supervised learning,contrastive learning,multi-arm bandit task,behavior analysis
AI 理解论文
溯源树
样例
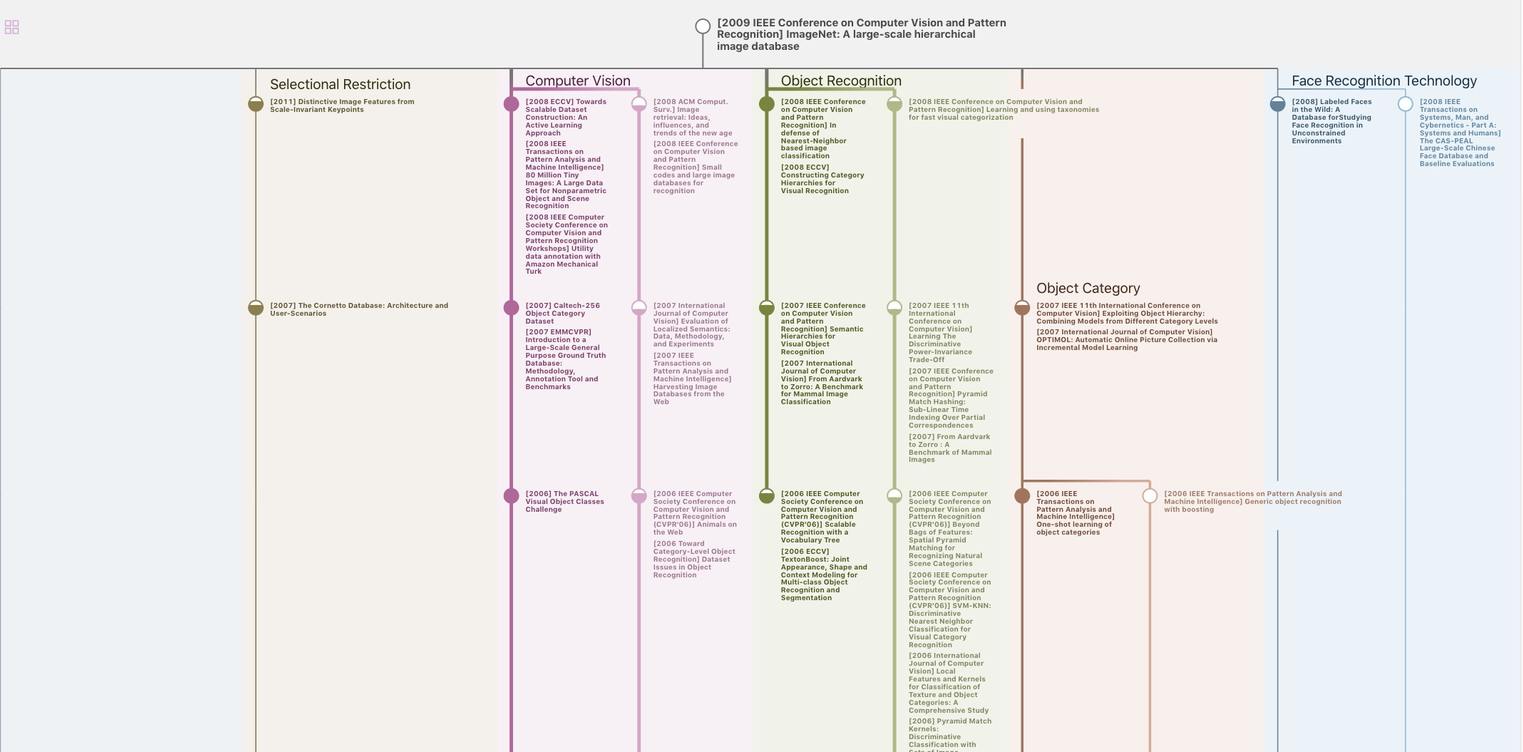
生成溯源树,研究论文发展脉络
Chat Paper
正在生成论文摘要