Benchmarking Interpretability Tools for Deep Neural Networks
arxiv(2023)
摘要
Interpreting deep neural networks is the topic of much current research in AI. However, few interpretability techniques have shown to be competitive tools in practical applications. Inspired by how benchmarks tend to guide progress in AI, we make three contributions. First, we propose trojan rediscovery as a benchmarking task to evaluate how useful interpretability tools are for generating engineering-relevant insights. Second, we design two such approaches for benchmarking: one for feature attribution methods and one for feature synthesis methods. Third, we apply our benchmarks to evaluate 16 feature attribution/saliency methods and 9 feature synthesis methods. This approach finds large differences in the capabilities of these existing tools and shows significant room for improvement. Finally, we propose several directions for future work. Resources are available at https://github.com/thestephencasper/benchmarking_interpretability
更多查看译文
AI 理解论文
溯源树
样例
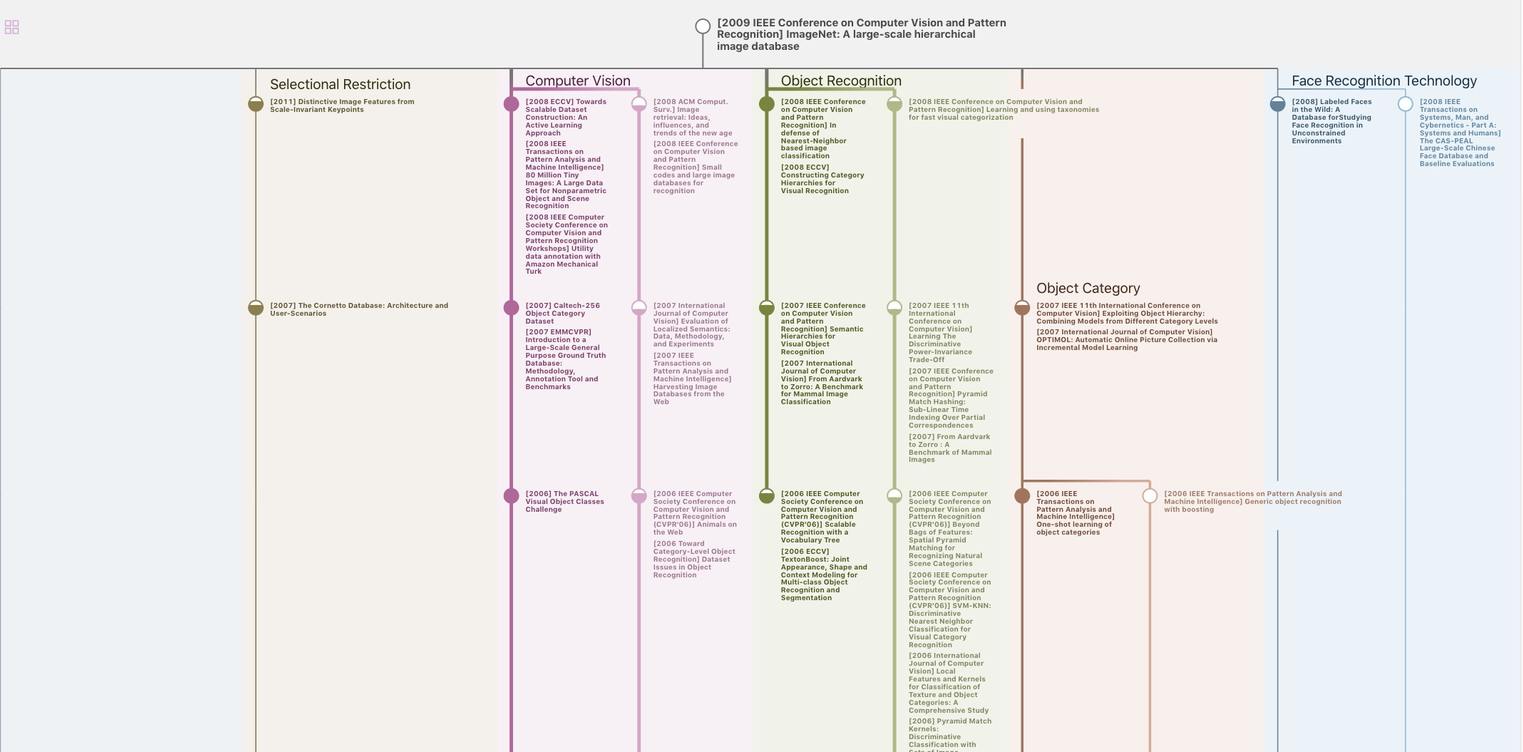
生成溯源树,研究论文发展脉络
Chat Paper
正在生成论文摘要