A Novel Design of Power System Stabilizer via GWO-tuned Radial-basis Function Neural Network for Damping Power Oscillations
2022 IEEE 10th Power India International Conference (PIICON)(2022)
摘要
Modern power system networks are having complicated structural designs and are subjected to a variety of undesirable occurrences such as lines and generator outages, transmission line faults, power oscillations, etc. An unavoidable phenomenon of a power system is low-frequency oscillations. To ensure the reliable operation of the system, these oscillations must be appropriately dampened. Therefore, in order to improve the stability of overall system by dampening low-frequency oscillations in a single-machine infinite bus test system, this work proposes a grey wolf optimizer (GWO) tuned radial basis function neural network (RBFNN) based design of power system stabilizer (PSS). The performance of RBFNN depends on proper choice of hyperparameters i.e. spread and maximum number of neurons. Here, GWO is used to tune these two hyperparameters of RBFNN in context of design of PSS. Diverse test cases are considered to test the performance of proposed PSS. The results obtained from GWO-RBFNN based PSSs are compared with the results obtained from existing conventional PSSs. The proposed PSS is found to be performing effectively in context of damping power oscillations.
更多查看译文
关键词
Grey wolf optimization,power oscillation,power system stabilizer,radial basis function neural network,single machine infinite bus test system
AI 理解论文
溯源树
样例
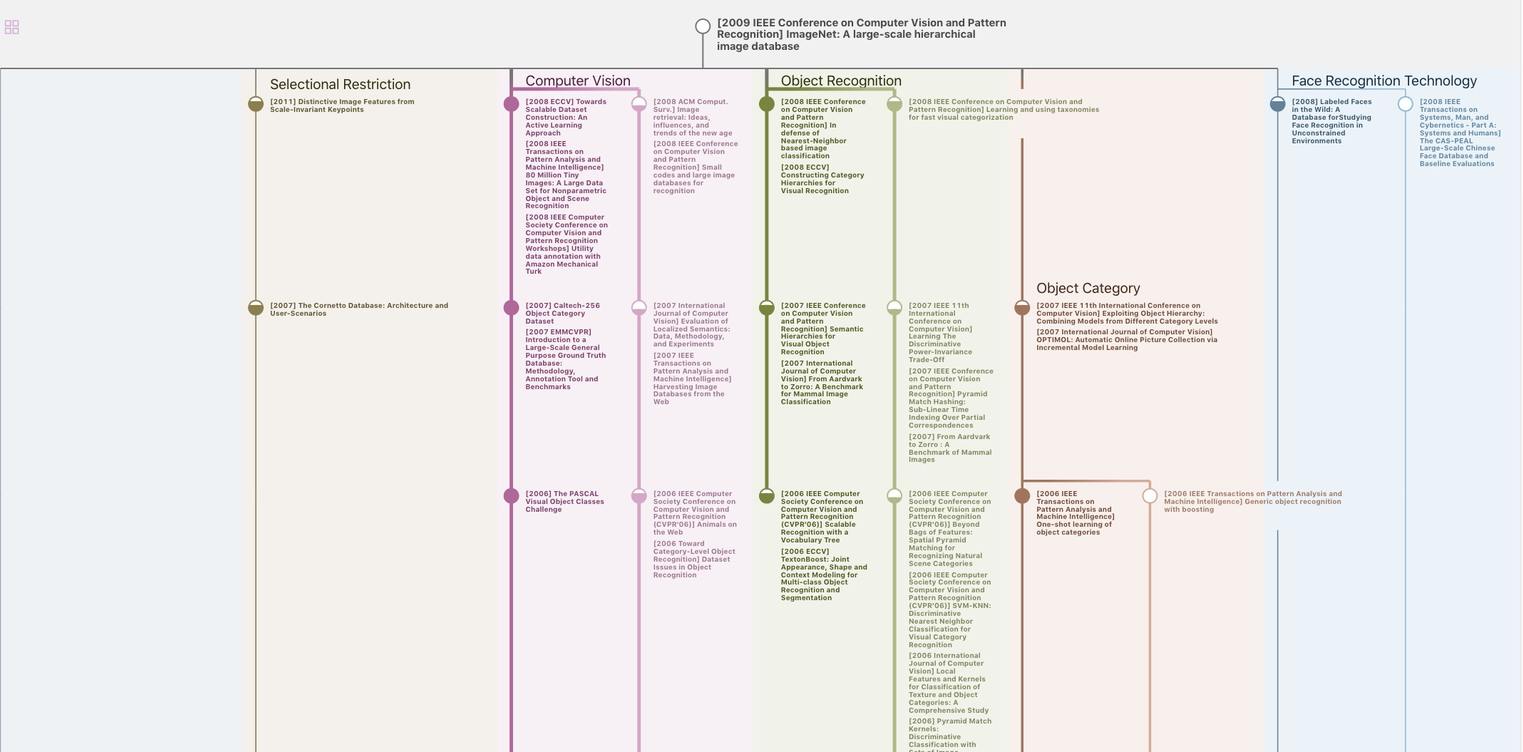
生成溯源树,研究论文发展脉络
Chat Paper
正在生成论文摘要