A Visual Representation-Guided Framework With Global Affinity for Weakly Supervised Salient Object Detection
IEEE TRANSACTIONS ON CIRCUITS AND SYSTEMS FOR VIDEO TECHNOLOGY(2024)
摘要
Fully supervised salient object detection (SOD) methods have made considerable progress in performance, yet these models rely heavily on expensive pixel-wise labels. Recently, to achieve a trade-off between labeling burden and performance, scribble-based SOD methods have attracted increasing attention. Previous scribble-based models directly implement the SOD task only based on SOD training data with limited information, it is extremely difficult for them to understand the image and further achieve a superior SOD task. In this paper, we propose a simple yet effective framework guided by general visual representations with rich contextual semantic knowledge for scribble-based SOD. These general visual representations are generated by self-supervised learning based on large-scale unlabeled datasets. Our framework consists of a task-related encoder, a general visual module, and an information integration module to efficiently combine the general visual representations with task-related features to perform the SOD task based on understanding the contextual connections of images. Meanwhile, we propose a novel global semantic affinity loss to guide the model to perceive the global structure of the salient objects. Experimental results on five public benchmark datasets demonstrate that our method, which only utilizes scribble annotations without introducing any extra label, outperforms the state-of-theart weakly supervised SOD methods. Specifically, it outperforms the previous best scribble-based method on all datasets with an average gain of 5.5% for max f-measure, 5.8% for mean f-measure, 24% for MAE, and 3.1% for E-measure. Moreover, our method achieves comparable or even superior performance to the state-of-the-art fully supervised models.
更多查看译文
关键词
Visualization,Task analysis,Image edge detection,Annotations,Semantics,Training,Object detection,General visual representation,global affinity,salient object detection,scribble,self-supervised transformer
AI 理解论文
溯源树
样例
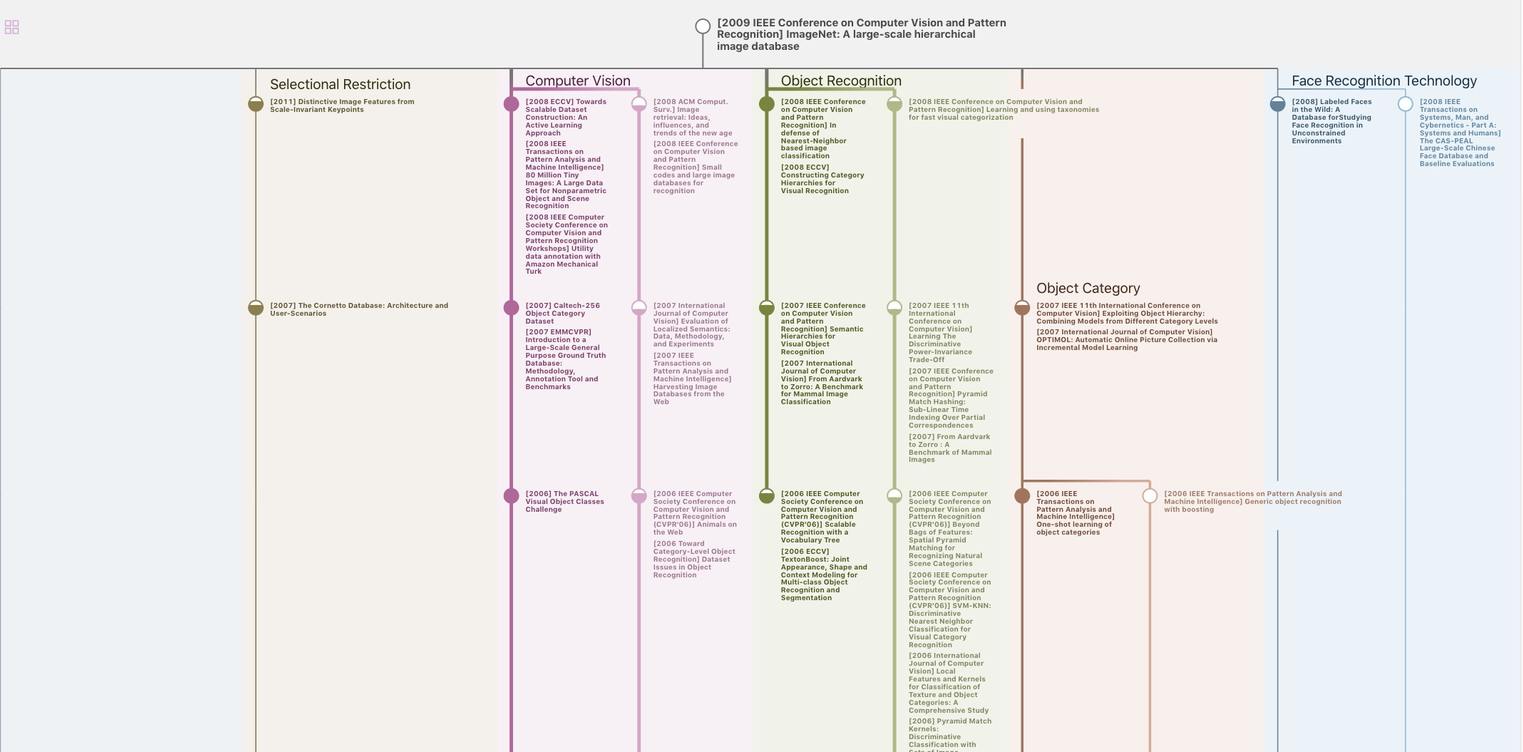
生成溯源树,研究论文发展脉络
Chat Paper
正在生成论文摘要