A Survey of Trustworthy Federated Learning with Perspectives on Security, Robustness and Privacy
COMPANION OF THE WORLD WIDE WEB CONFERENCE, WWW 2023(2023)
摘要
Trustworthy artificial intelligence (AI) technology has revolutionized daily life and greatly benefited human society. Among various AI technologies, Federated Learning (FL) stands out as a promising solution for diverse real-world scenarios, ranging from risk evaluation systems in finance to cutting-edge technologies like drug discovery in life sciences. However, challenges around data isolation and privacy threaten the trustworthiness of FL systems. Adversarial attacks against data privacy, learning algorithm stability, and system confidentiality are particularly concerning in the context of distributed training in federated learning. Therefore, it is crucial to develop FL in a trustworthy manner, with a focus on robustness and privacy. In this survey, we propose a comprehensive roadmap for developing trustworthy FL systems and summarize existing efforts from two key aspects: robustness and privacy. We outline the threats that pose vulnerabilities to trustworthy federated learning across different stages of development, including data processing, model training, and deployment. To guide the selection of the most appropriate defense methods, we discuss specific technical solutions for realizing each aspect of Trustworthy FL (TFL). Our approach differs from previous work that primarily discusses TFL from a legal perspective or presents FL from a high-level, non-technical viewpoint.
更多查看译文
关键词
Federated Learning,Security,Robustness,Privacy
AI 理解论文
溯源树
样例
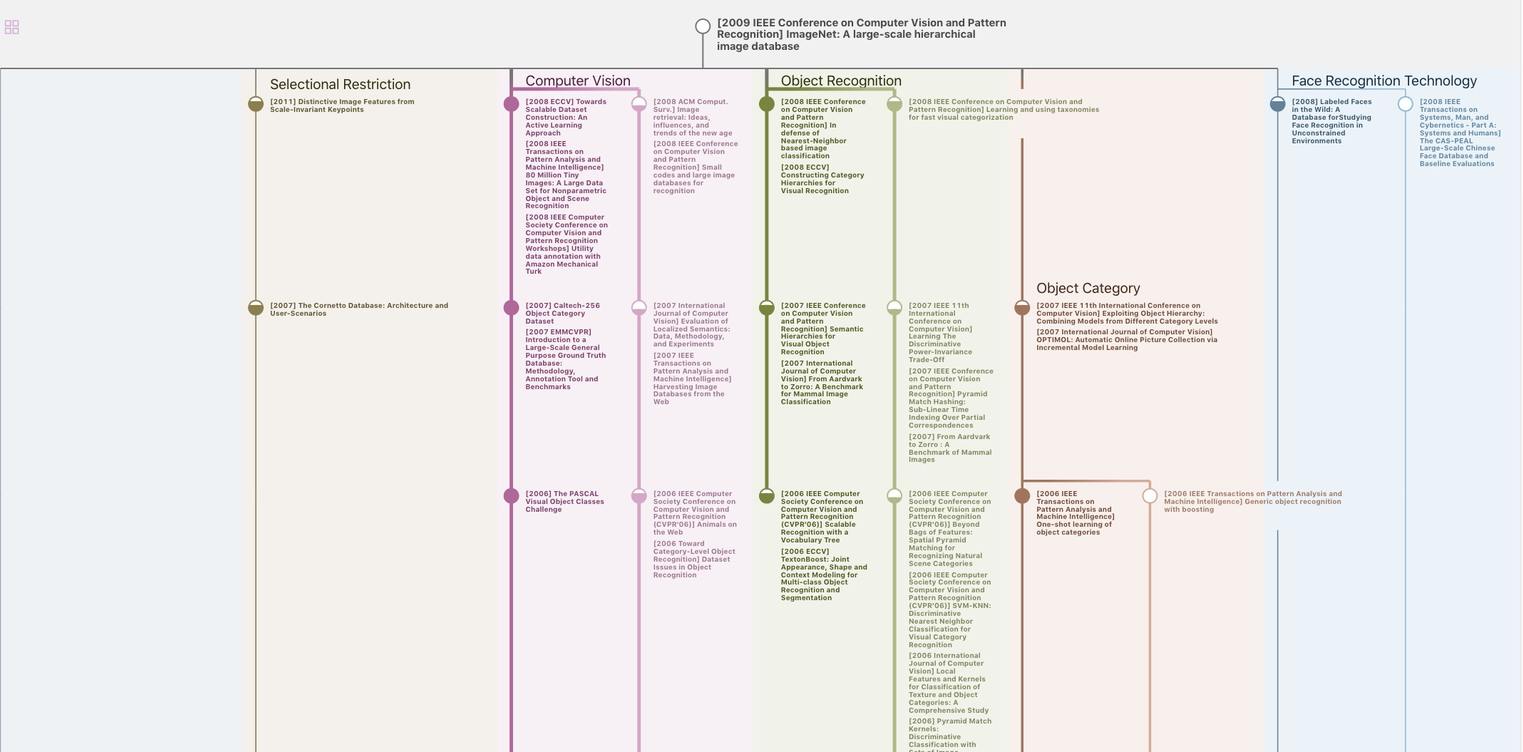
生成溯源树,研究论文发展脉络
Chat Paper
正在生成论文摘要