Structured Bayesian Compression for Deep Neural Networks Based on The Turbo-VBI Approach
arxiv(2023)
摘要
With the growth of neural network size, model compression has attracted increasing interest in recent research. As one of the most common techniques, pruning has been studied for a long time. By exploiting the structured sparsity of the neural network, existing methods can prune neurons instead of individual weights. However, in most existing pruning methods, surviving neurons are randomly connected in the neural network without any structure, and the non-zero weights within each neuron are also randomly distributed. Such irregular sparse structure can cause very high control overhead and irregular memory access for the hardware and even increase the neural network computational complexity. In this paper, we propose a three-layer hierarchical prior to promote a more regular sparse structure during pruning. The proposed three-layer hierarchical prior can achieve per-neuron weight-level structured sparsity and neuron-level structured sparsity. We derive an efficient Turbo-variational Bayesian inferencing (Turbo-VBI) algorithm to solve the resulting model compression problem with the proposed prior. The proposed Turbo-VBI algorithm has low complexity and can support more general priors than existing model compression algorithms. Simulation results show that our proposed algorithm can promote a more regular structure in the pruned neural networks while achieving even better performance in terms of compression rate and inferencing accuracy compared with the baselines.
更多查看译文
关键词
Deep neural networks,group sparsity,model compression,pruning
AI 理解论文
溯源树
样例
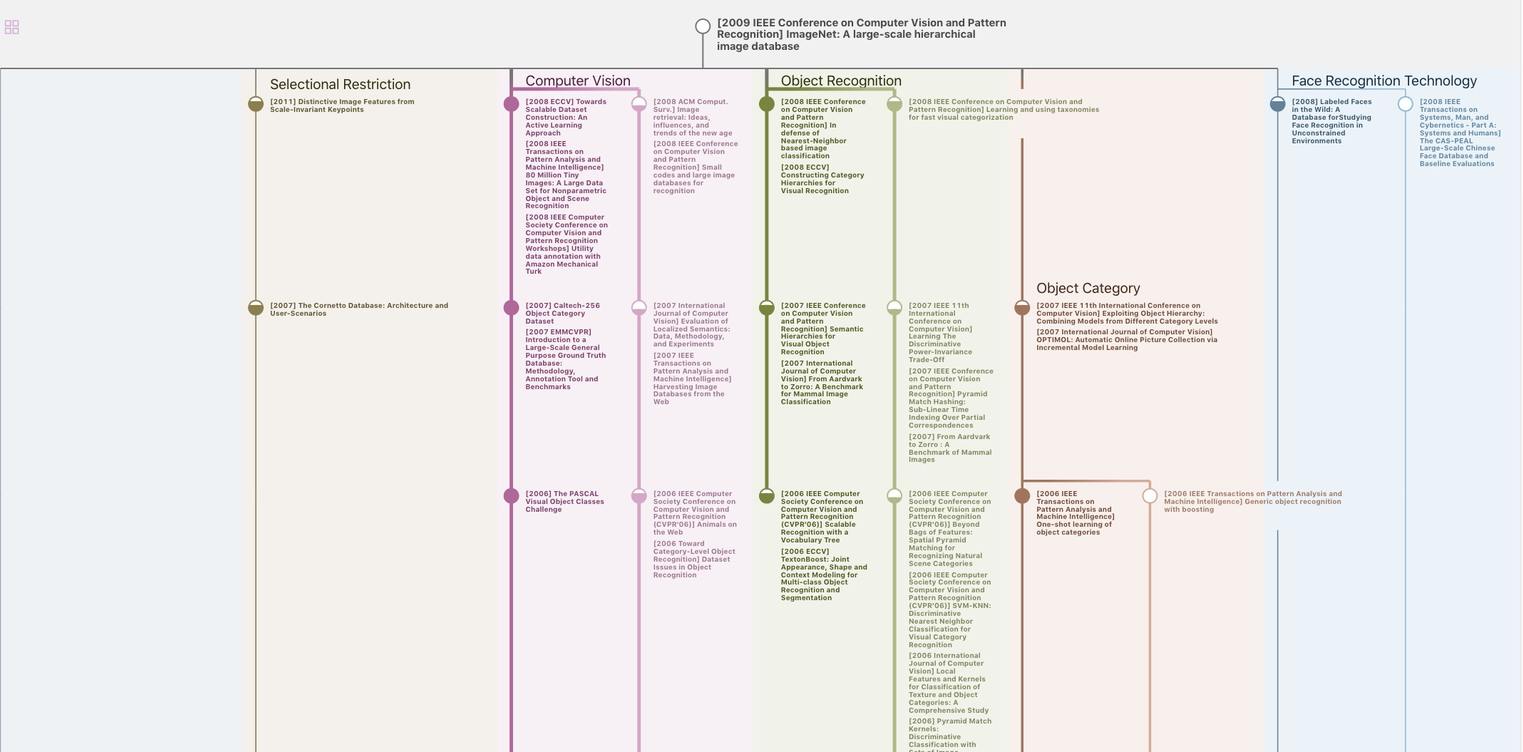
生成溯源树,研究论文发展脉络
Chat Paper
正在生成论文摘要