Online evolutionary neural architecture search for multivariate non-stationary time series forecasting
APPLIED SOFT COMPUTING(2023)
摘要
Time series forecasting (TSF) is one of the most important tasks in data science. TSF models are usually pre-trained with historical data and then applied on future unseen datapoints. However, real-world time series data is usually non-stationary and models trained offline usually face problems from data drift. Models trained and designed in an offline fashion can not quickly adapt to changes quickly or be deployed in real-time. To address these issues, this work presents the Online NeuroEvolution-based Neural Architecture Search (ONE-NAS) algorithm, which is a novel neural architecture search method capable of automatically designing and dynamically training recurrent neural networks (RNNs) for online forecasting tasks. Without any pre-training, ONE-NAS utilizes populations of RNNs that are continuously updated with new network structures and weights in response to new multivariate input data. ONE-NAS is tested on real-world, large-scale multivariate wind turbine data as well as the univariate Dow Jones Industrial Average (DJIA) dataset. Results demonstrate that ONE-NAS outperforms traditional statistical time series forecasting methods, including online linear regression, fixed long short-term memory (LSTM) and gated recurrent unit (GRU) models trained online, as well as state-of-the-art, online ARIMA strategies. Additionally, results show that utilizing multiple populations of RNNs which are periodically repopulated provide significant performance improvements, allowing this online neural network architecture design and training to be successful.(c) 2023 Elsevier B.V. All rights reserved.
更多查看译文
关键词
Time series forecasting,Online learning,Neural architecture search,Recurrent neural networks,Neuroevolution
AI 理解论文
溯源树
样例
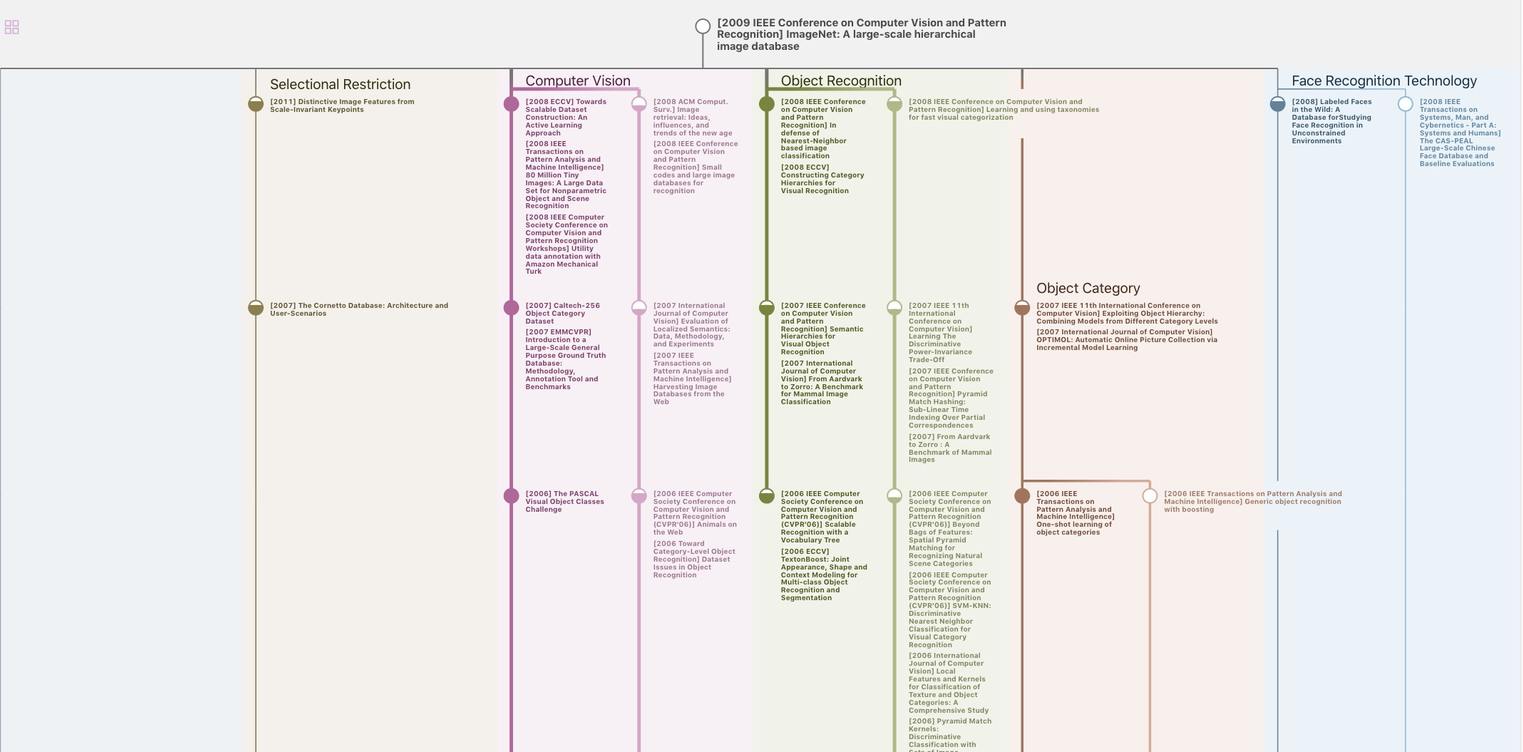
生成溯源树,研究论文发展脉络
Chat Paper
正在生成论文摘要