FedTAR: Task and Resource-Aware Federated Learning for Wireless Computing Power Networks
IEEE Internet of Things Journal(2023)
摘要
In the 6G era, the proliferation of data and data-intensive applications poses unprecedented challenges on the current communication and computing networks. The collaboration among cloud computing, edge computing, and networking is imperative to process such massive data, eventually realizing ubiquitous computing and intelligence. In this article, we propose a wireless computing power network (WCPN) by orchestrating the computing and networking resources of heterogeneous nodes toward specific computing tasks. To enable intelligent service in WCPN, we design a task and resource-aware federated learning model, coined FedTAR, which minimizes the sum energy consumption of all computing nodes by the joint optimization of the computing strategies of individual computing nodes and their collaborative learning strategy. Based on the solution of the optimization problem, the neural network depth of computing nodes and the collaboration frequency among nodes are adjustable according to specific computing task requirements and resource constraints. To further adapt to heterogeneous computing nodes, we then propose an energy-efficient asynchronous aggregation algorithm for FedTAR, which accelerates the convergence speed of federated learning in WCPN. Numerical results show that the proposed scheme outperforms the existing studies in terms of learning accuracy, convergence rate, and energy saving.
更多查看译文
关键词
Federated learning,mobile-edge computing (MEC),neural network,wireless computing power networks (WCPNs)
AI 理解论文
溯源树
样例
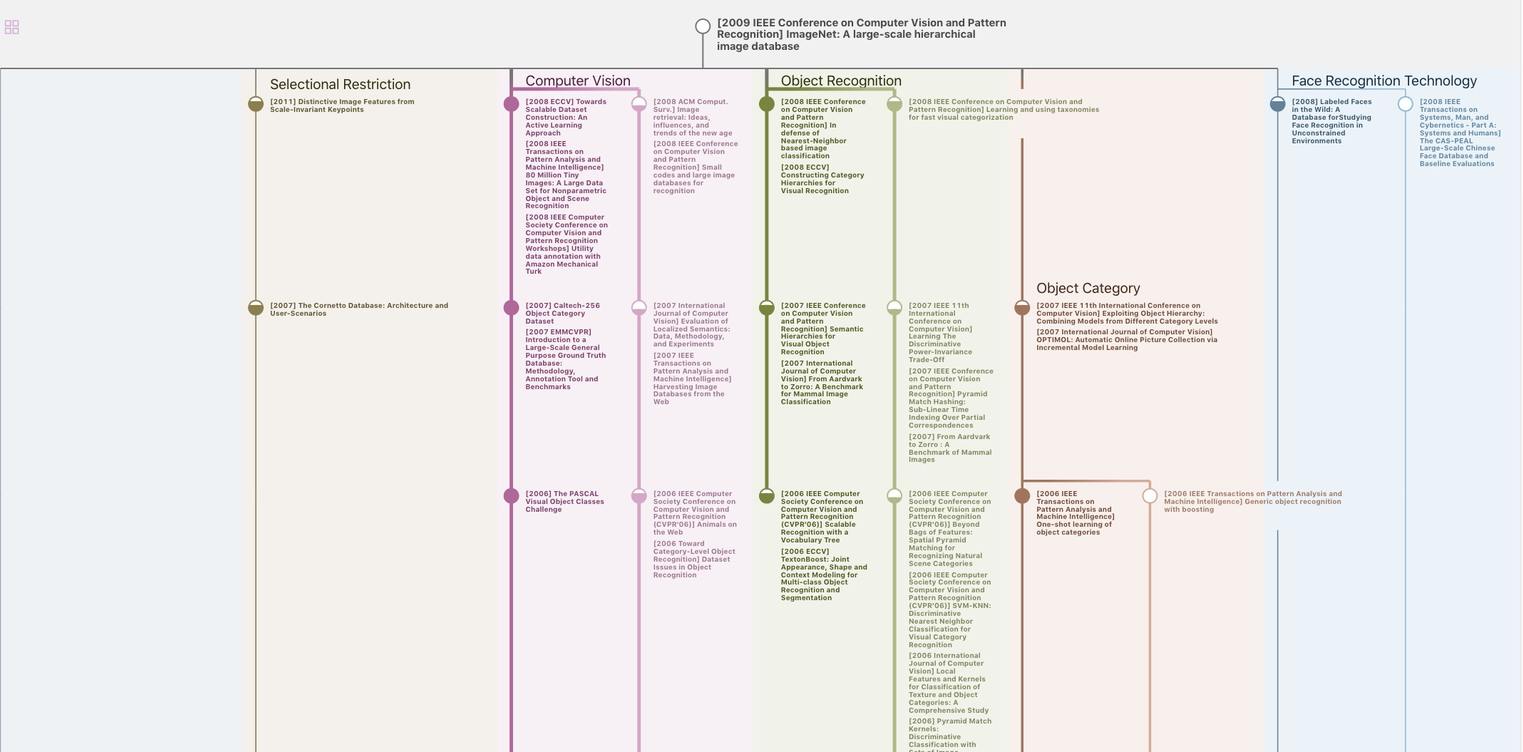
生成溯源树,研究论文发展脉络
Chat Paper
正在生成论文摘要