Towards Understanding the Survival of Patients with High-Grade Gastroenteropancreatic Neuroendocrine Neoplasms: An Investigation of Ensemble Feature Selection in the Prediction of Overall Survival
arxiv(2023)
摘要
Determining the most informative features for predicting the overall survival of patients diagnosed with high-grade gastroenteropancreatic neuroendocrine neoplasms is crucial to improve individual treatment plans for patients, as well as the biological understanding of the disease. Recently developed ensemble feature selectors like the Repeated Elastic Net Technique for Feature Selection (RENT) and the User-Guided Bayesian Framework for Feature Selection (UBayFS) allow the user to identify such features in datasets with low sample sizes. While RENT is purely data-driven, UBayFS is capable of integrating expert knowledge a priori in the feature selection process. In this work we compare both feature selectors on a dataset comprising of 63 patients and 134 features from multiple sources, including basic patient characteristics, baseline blood values, tumor histology, imaging, and treatment information. Our experiments involve data-driven and expert-driven setups, as well as combinations of both. We use findings from clinical literature as a source of expert knowledge. Our results demonstrate that both feature selectors allow accurate predictions, and that expert knowledge has a stabilizing effect on the feature set, while the impact on predictive performance is limited. The features WHO Performance Status, Albumin, Platelets, Ki-67, Tumor Morphology, Total MTV, Total TLG, and SUVmax are the most stable and predictive features in our study.
更多查看译文
关键词
gastroenteropancreatic neuroendocrine neoplasms,ensemble
AI 理解论文
溯源树
样例
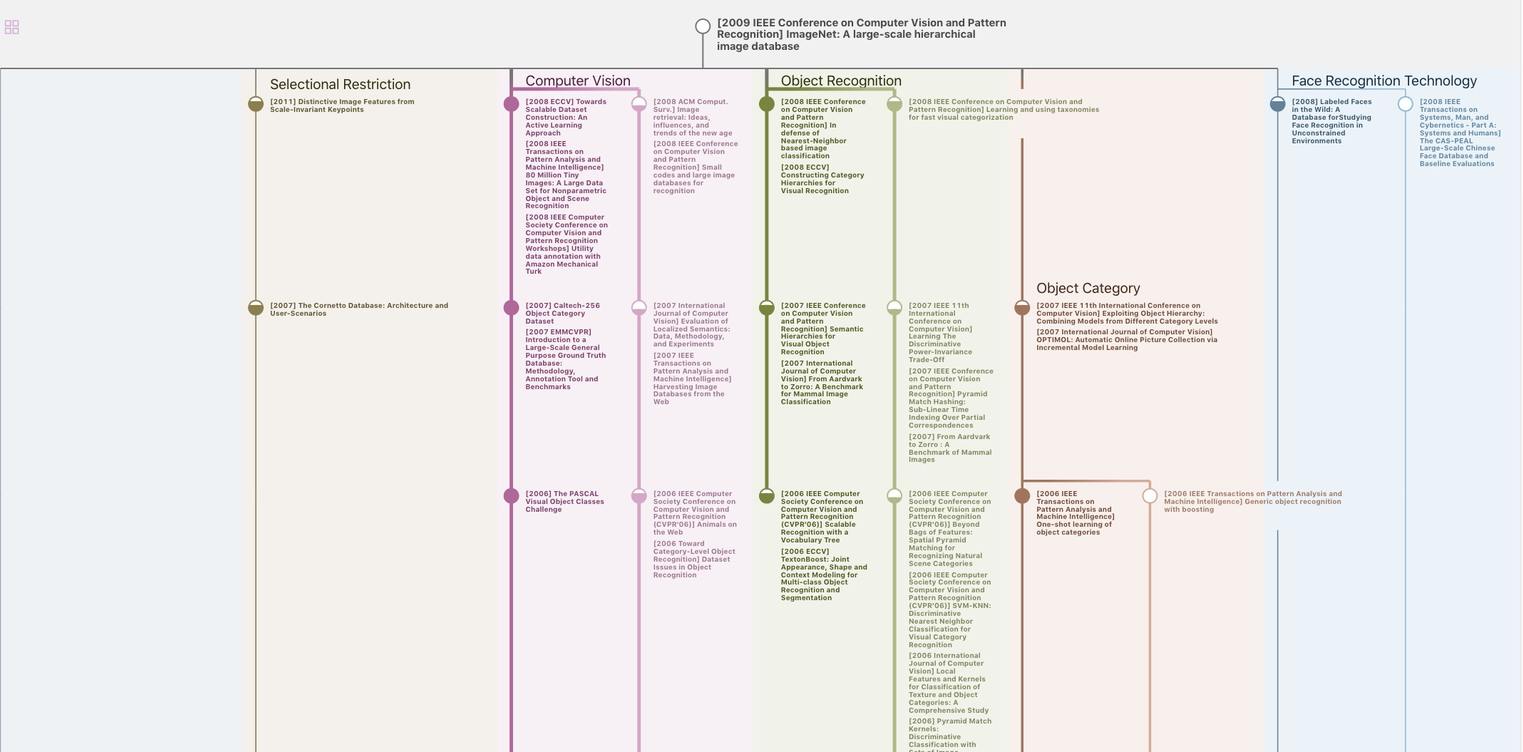
生成溯源树,研究论文发展脉络
Chat Paper
正在生成论文摘要