Unsupervised OmniMVS: Efficient Omnidirectional Depth Inference via Establishing Pseudo-Stereo Supervision
2023 IEEE/RSJ INTERNATIONAL CONFERENCE ON INTELLIGENT ROBOTS AND SYSTEMS (IROS)(2023)
摘要
Omnidirectional multi-view stereo (MVS) vision is attractive for its ultra-wide field-of-view (FoV), enabling machines to perceive 360 degrees 3D surroundings. However, the existing solutions require expensive dense depth labels for supervision, making them impractical in real-world applications. In this paper, we propose the first unsupervised omnidirectional MVS framework based on multiple fisheye images. To this end, we project all images to a virtual view center and composite two panoramic images with spherical geometry from two pairs of back-to-back fisheye images. The two 360 degrees images formulate a stereo pair with a special pose, and the photometric consistency is leveraged to establish the unsupervised constraint, which we term "Pseudo-Stereo Supervision". In addition, we propose Un-OmniMVS, an efficient unsupervised omnidirectional MVS network, to facilitate the inference speed with two efficient components. First, a novel feature extractor with frequency attention is proposed to simultaneously capture the non-local Fourier features and local spatial features, explicitly facilitating the feature representation. Then, a variance-based light cost volume is put forward to reduce the computational complexity. Experiments exhibit that the performance of our unsupervised solution is competitive to that of the state-of-the-art (SoTA) supervised methods with better generalization in real-world data. The code will be available at https://github.com/Chen-z-s/Un-OmniMVS.
更多查看译文
AI 理解论文
溯源树
样例
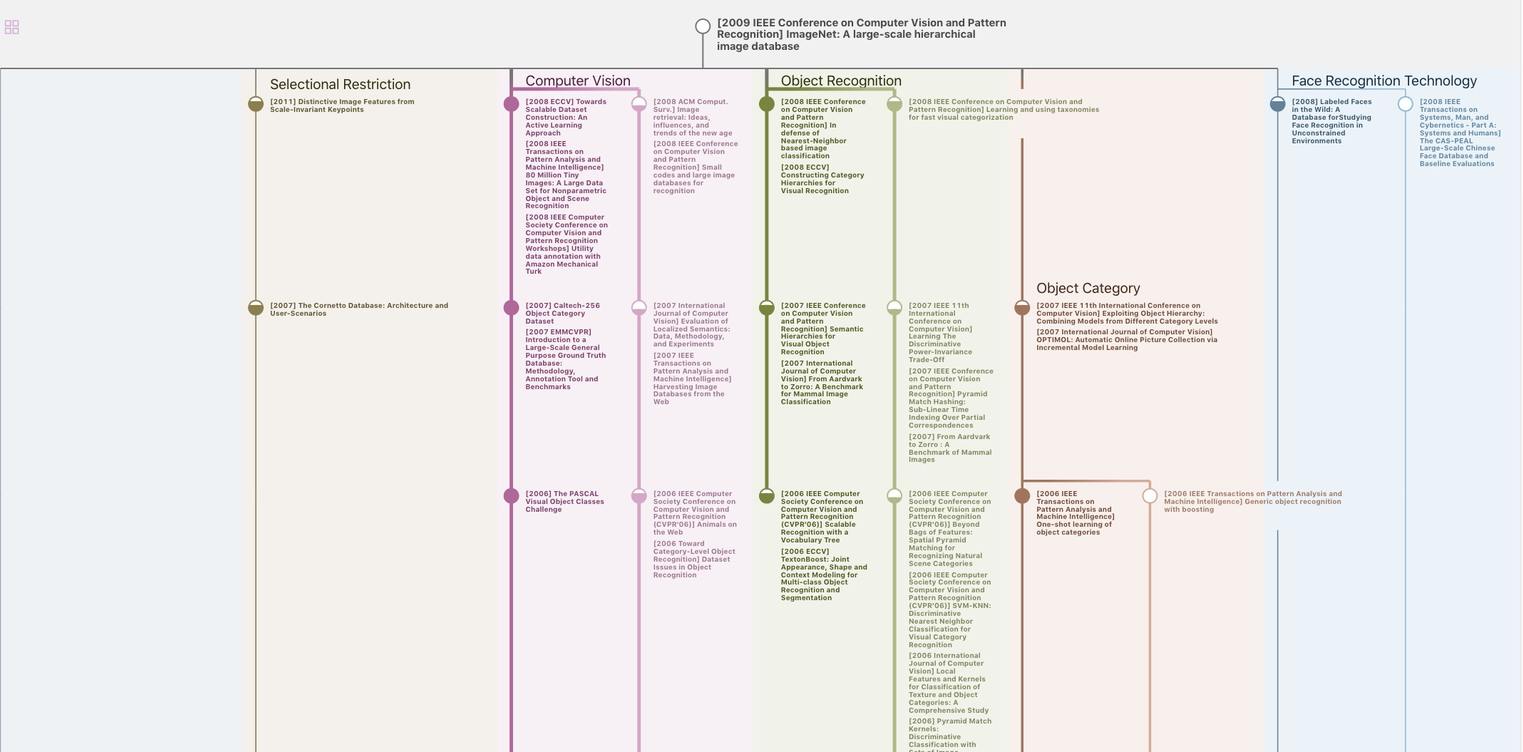
生成溯源树,研究论文发展脉络
Chat Paper
正在生成论文摘要