Predicting structure-dependent Hubbard U parameters via machine learning
MATERIALS FUTURES(2024)
摘要
DFT + U is a widely used treatment in the density functional theory (DFT) to deal with correlated materials that contain open-shell elements, whereby the quantitative and sometimes even qualitative failures of local and semi-local approximations can be corrected without much computational overhead. However, finding appropriate U parameters for a given system and structure is non-trivial and computationally intensive, because the U value has generally a strong chemical and structural dependence. In this work, we address this issue by building a machine learning (ML) model that enables the prediction of material- and structure-specific U values at nearly no computational cost. Using Mn-O system as an example, the ML model is trained by calibrating DFT + U electronic structures with the hybrid functional results of more than 3000 structures. The model allows us to determine an accurate U value (MAE = 0.128 eV, R-2 = 0.97) for any given Mn-O structure. Further analysis reveals that M-O bond lengths are key local structural properties in determining the U value. This approach of the ML U model is universally applicable, to significantly expand and solidify the use of the DFT + U method.
更多查看译文
关键词
DFT plus U,machine learning,Bayesian optimization
AI 理解论文
溯源树
样例
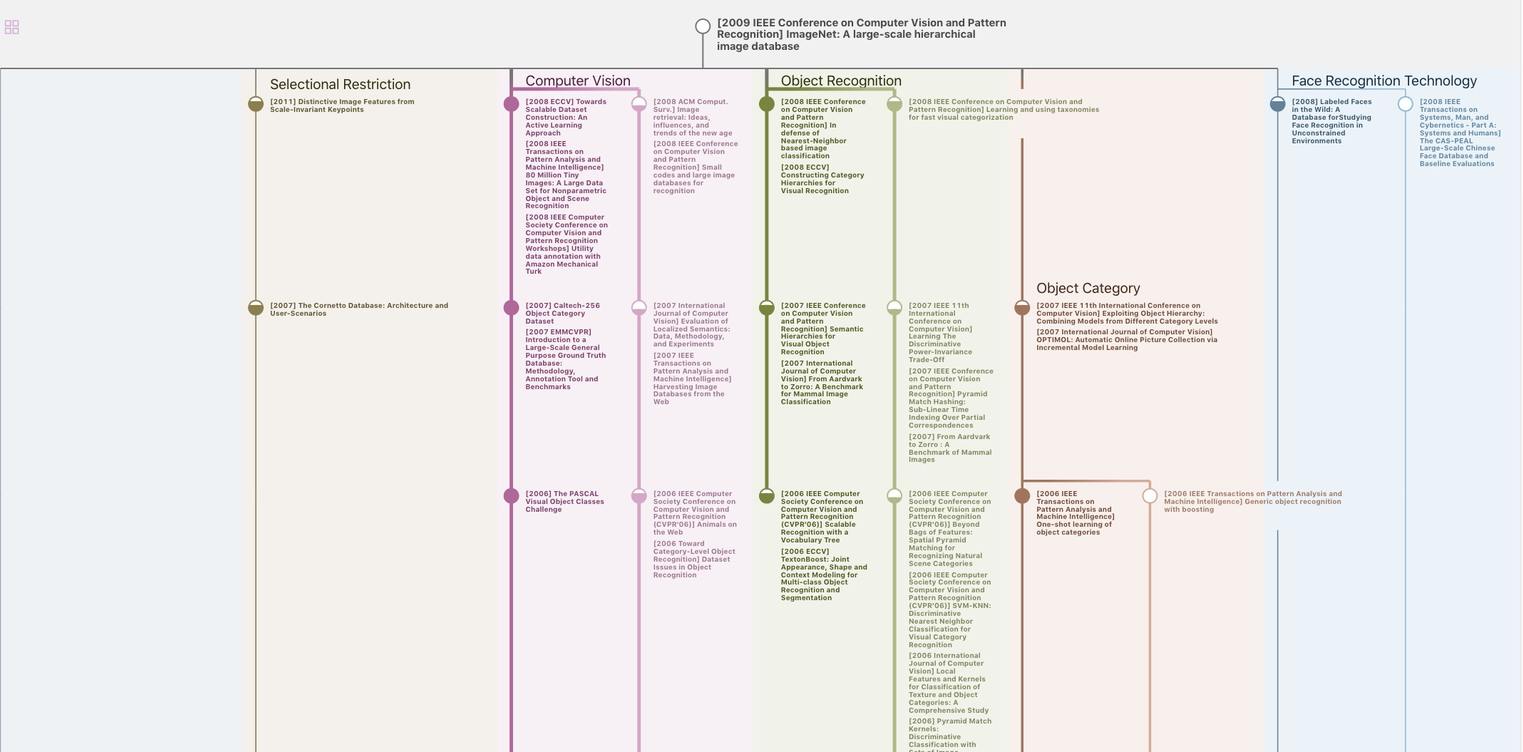
生成溯源树,研究论文发展脉络
Chat Paper
正在生成论文摘要