Multistage Spatial Context Models for Learned Image Compression
arxiv(2023)
摘要
Recent state-of-the-art Learned Image Compression methods feature spatial context models, achieving great rate-distortion improvements over hyperprior methods. However, the autoregressive context model requires serial decoding, limiting runtime performance. The Checkerboard context model allows parallel decoding at a cost of reduced RD performance. We present a series of multistage spatial context models allowing both fast decoding and better RD performance. We split the latent space into square patches and decode serially within each patch while different patches are decoded in parallel. The proposed method features a comparable decoding speed to Checkerboard while reaching the RD performance of Autoregressive and even also outperforming Autoregressive. Inside each patch, the decoding order must be carefully decided as a bad order negatively impacts performance; therefore, we also propose a decoding order optimization algorithm.
更多查看译文
关键词
compression,context,image,models,learned
AI 理解论文
溯源树
样例
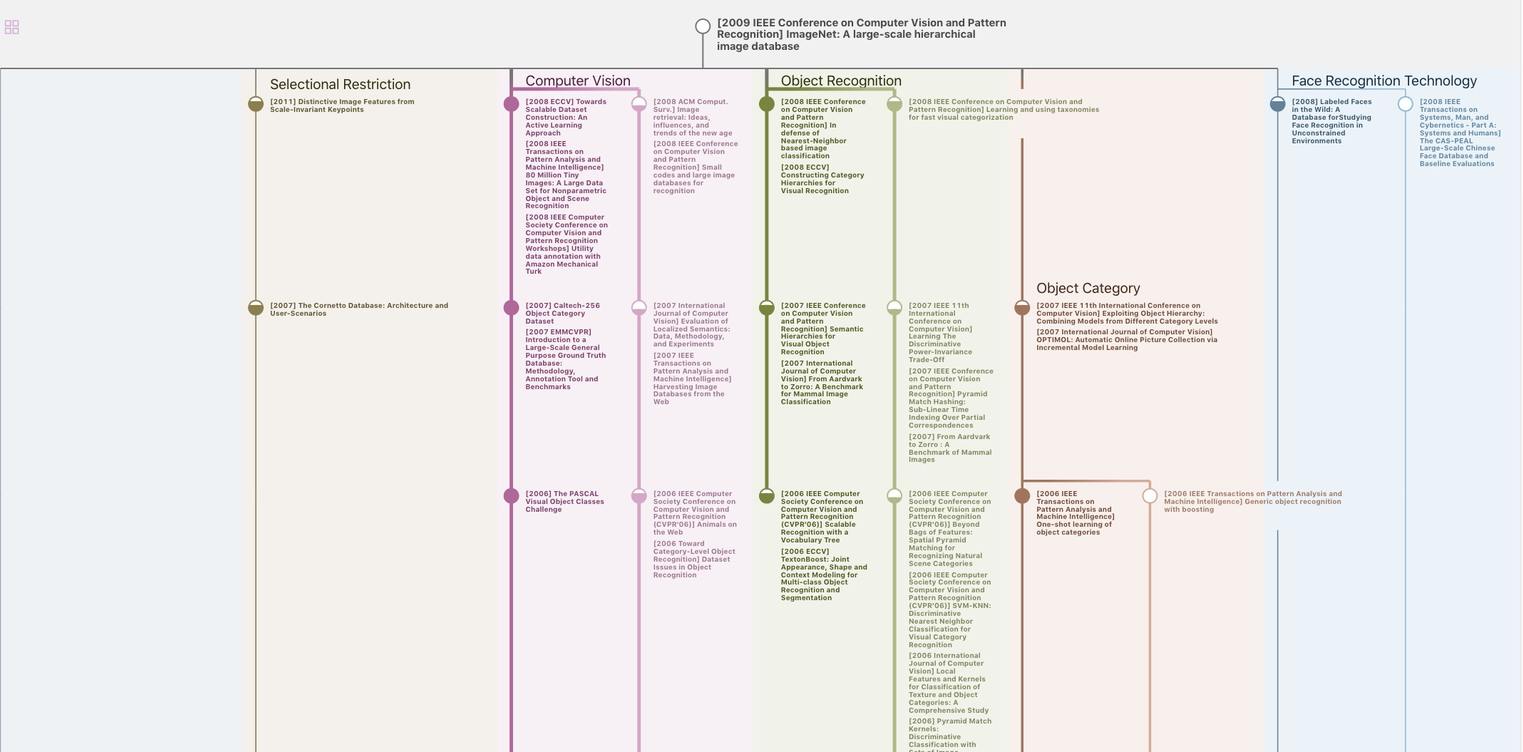
生成溯源树,研究论文发展脉络
Chat Paper
正在生成论文摘要