Auto-weighted collective matrix factorization with graph dual regularization for multi-view clustering
Knowledge-Based Systems(2023)
摘要
Multi-view clustering (MVC) is an attractive clustering paradigm that can incorporate comprehensive information from multiple views. Among the MVC schemes, collective matrix factorization (CMF) has shown its great power in extracting shared information of multi-view data. Based on CMF, we propose a novel unified MVC framework, named Auto-weighted Collective Matrix Factorization with Graph Dual Regularization (ACMF-GDR). Specifically, we assign adaptive weights for each view and incorporate the smoothing cluster structure learning term to construct a unified auto-weighted CMF for MVC. Our ACMF-GDR model can obtain the cluster labels and common representations of the samples in a one-step manner. Furthermore, to make the common representations discriminative, graph dual regularization terms with orthogonality constraints are adopted on multiple views to preserve the geometrical structure of the decomposed factors simultaneously. Experimental results show the superior clustering performance of the proposed method.
更多查看译文
关键词
Multi-view clustering,Nonnegative matrix factorization,Adaptive weight,Graph dual regularization
AI 理解论文
溯源树
样例
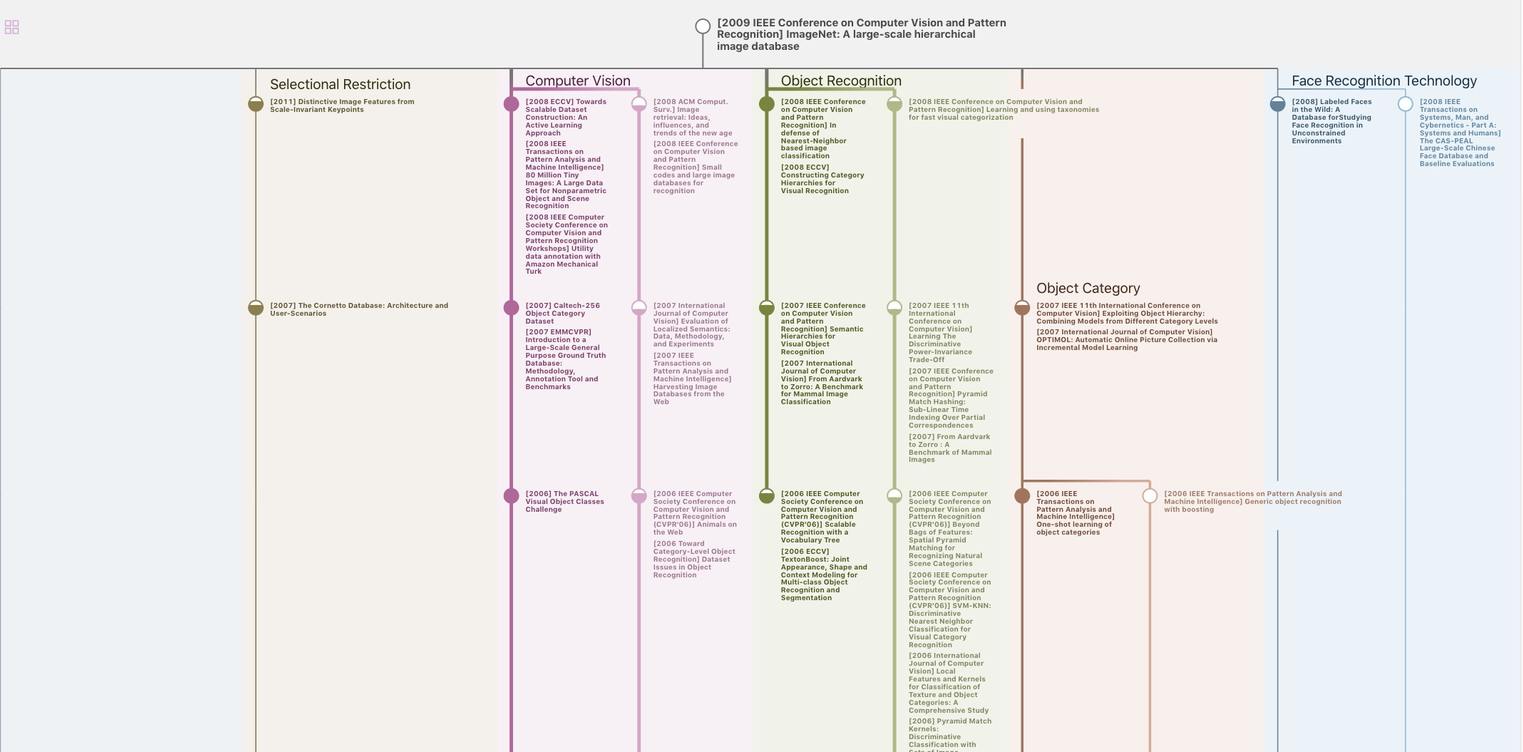
生成溯源树,研究论文发展脉络
Chat Paper
正在生成论文摘要