Enhancing Dyadic Relations with Homogeneous Graphs for Multimodal Recommendation.
CoRR(2023)
摘要
User-item interaction data in recommender systems is a form of dyadic relation, reflecting user preferences for specific items. To generate accurate recommendations, it is crucial to learn representations for both users and items. Recent multimodal recommendation models achieve higher accuracy by incorporating multimodal features, such as images and text descriptions. However, our experimental findings reveal that current multimodality fusion methods employed in state-of-the-art models may adversely affect recommendation performance without compromising model architectures. Moreover, these models seldom investigate internal relations between item-item and user-user interactions. In light of these findings, we propose a model that enhances the dyadic relations by learning Dual RepresentAtions of both users and items via constructing homogeneous Graphs for multimOdal recommeNdation. We name our model as DRAGON. Specifically, DRAGON constructs user-user graphs based on commonly interacted items and item-item graphs derived from item multimodal features. Graph learning on both the user-item heterogeneous and homogeneous graphs is used to obtain dual representations of users and items. To capture information from each modality, DRAGON employs an effective fusion method, attentive concatenation. Extensive experiments on three public datasets and eight baselines show that DRAGON can outperform the strongest baseline by 21.41% on average. Our code is available at https://github.com/hongyurain/DRAGON.
更多查看译文
关键词
dyadic relations,homogeneous graphs,recommendation
AI 理解论文
溯源树
样例
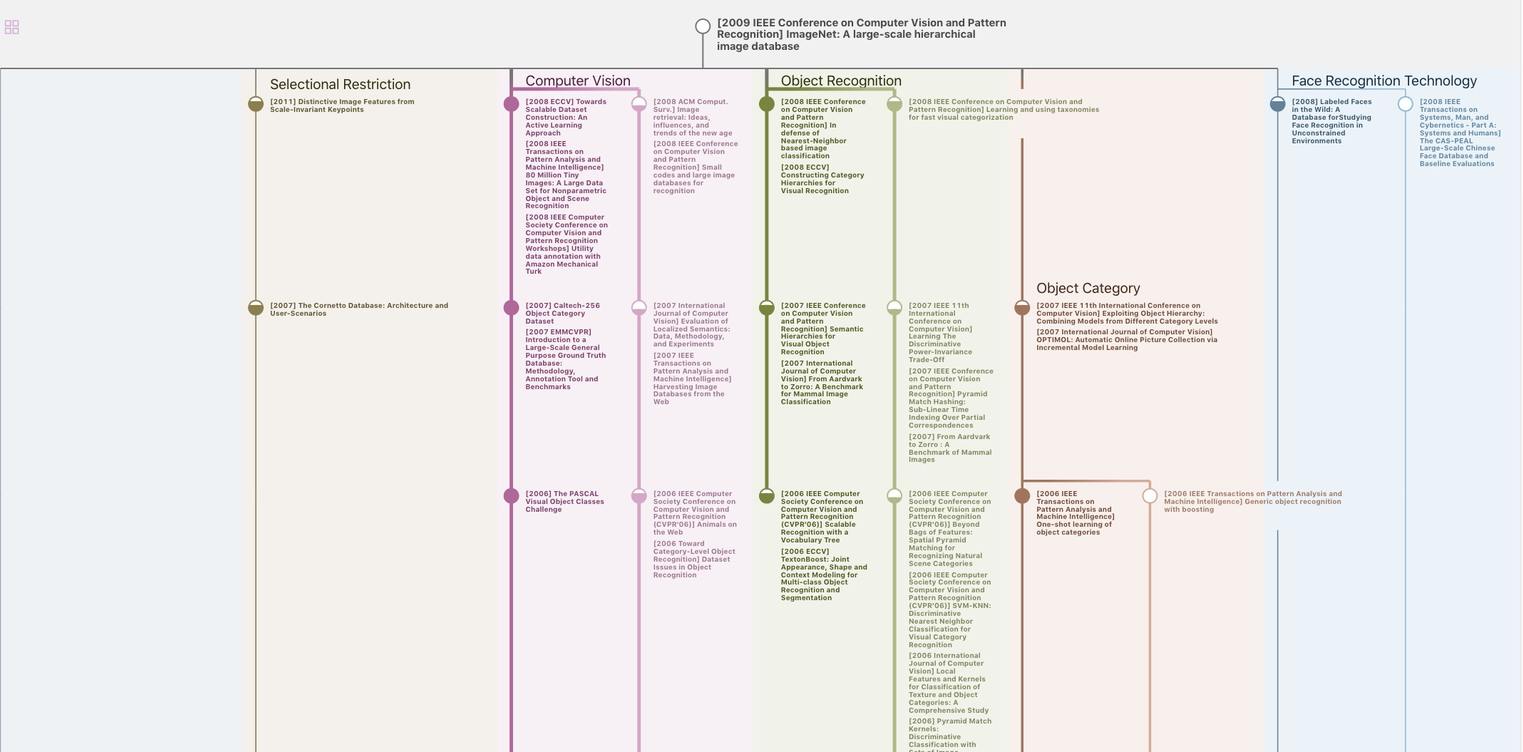
生成溯源树,研究论文发展脉络
Chat Paper
正在生成论文摘要