A DRL Approach for RIS-Assisted Full-Duplex UL and DL Transmission: Beamforming, Phase Shift and Power Optimization.
CoRR(2022)
摘要
In this work, we present a two-stage deep reinforcement learning (DRL) approach for a full-duplex (FD) transmission scenario that predicts the phase-shifts of the reconfigurable intelligent surface (RIS), active beamformers at the base station (BS) and the transmit powers of BS and uplink users to maximize the weighted sum-rate of uplink and downlink users. The proposed approach does not depend on the channel state information (CSI) or the knowledge of residual self-interference (SI) for its prediction. As the residual SI cancellation and beamformer design are coupled problems, the first stage of the two-stage framework uses a least squares method to cancel the residual SI partially and initiate learning. The second stage uses DRL to make predictions and achieve performance close to methods with perfect CSI knowledge. The proposed method also works in scenarios with mobile users. Further, the proposed DRL framework, which predicts the quantized RIS phase shifts, reduces the signaling from BS to the RISs using $32$ times fewer bits than the continuous version. The quantized methods have reduced action space and, therefore, faster convergence; with sufficient training, the UL and DL rates for the quantized phase method are $7.1\%$ and $22.28\%$ better than the continuous phase method.
更多查看译文
关键词
dl transmission
AI 理解论文
溯源树
样例
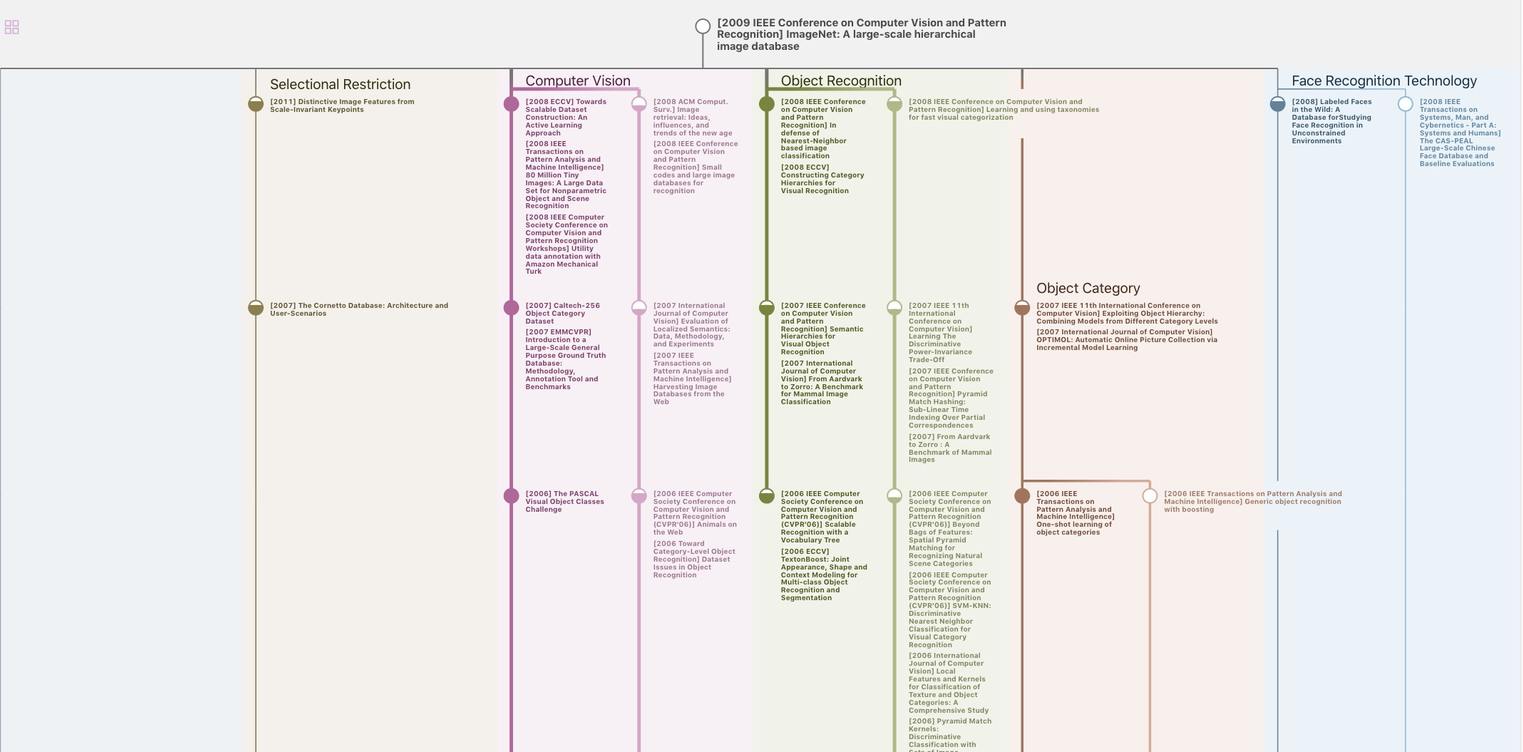
生成溯源树,研究论文发展脉络
Chat Paper
正在生成论文摘要