RainDiffusion: When Unsupervised Learning Meets Diffusion Models for Real-world Image Deraining
CoRR(2023)
摘要
Recent diffusion models show great potential in generative modeling tasks. This motivates us to raise an intriguing question - What will happen when unsupervised learning meets diffusion models for real-world image deraining? Before answering it, we observe two major obstacles of diffusion models in real-world image deraining: the need for paired training data and the limited utilization of multi-scale rain patterns. To overcome the obstacles, we propose RainDiffusion, the first real-world image deraining paradigm based on diffusion models. RainDiffusion is a non-adversarial training paradigm that introduces stable training of unpaired real-world data, rather than weakly adversarial training, serving as a new standard bar for real-world image deraining. It consists of two cooperative branches: Non-diffusive Translation Branch (NTB) and Diffusive Translation Branch (DTB). NTB exploits a cycle-consistent architecture to bypass the difficulty in unpaired training of regular diffusion models by generating initial clean/rainy image pairs. Given initial image pairs, DTB leverages multi-scale diffusion models to progressively refine the desired output via diffusive generative and multi-scale priors, to obtain a better generalization capacity of real-world image deraining. Extensive experiments confirm the superiority of our RainDiffusion over eight un/semi-supervised methods and show its competitive advantages over seven fully-supervised ones.
更多查看译文
AI 理解论文
溯源树
样例
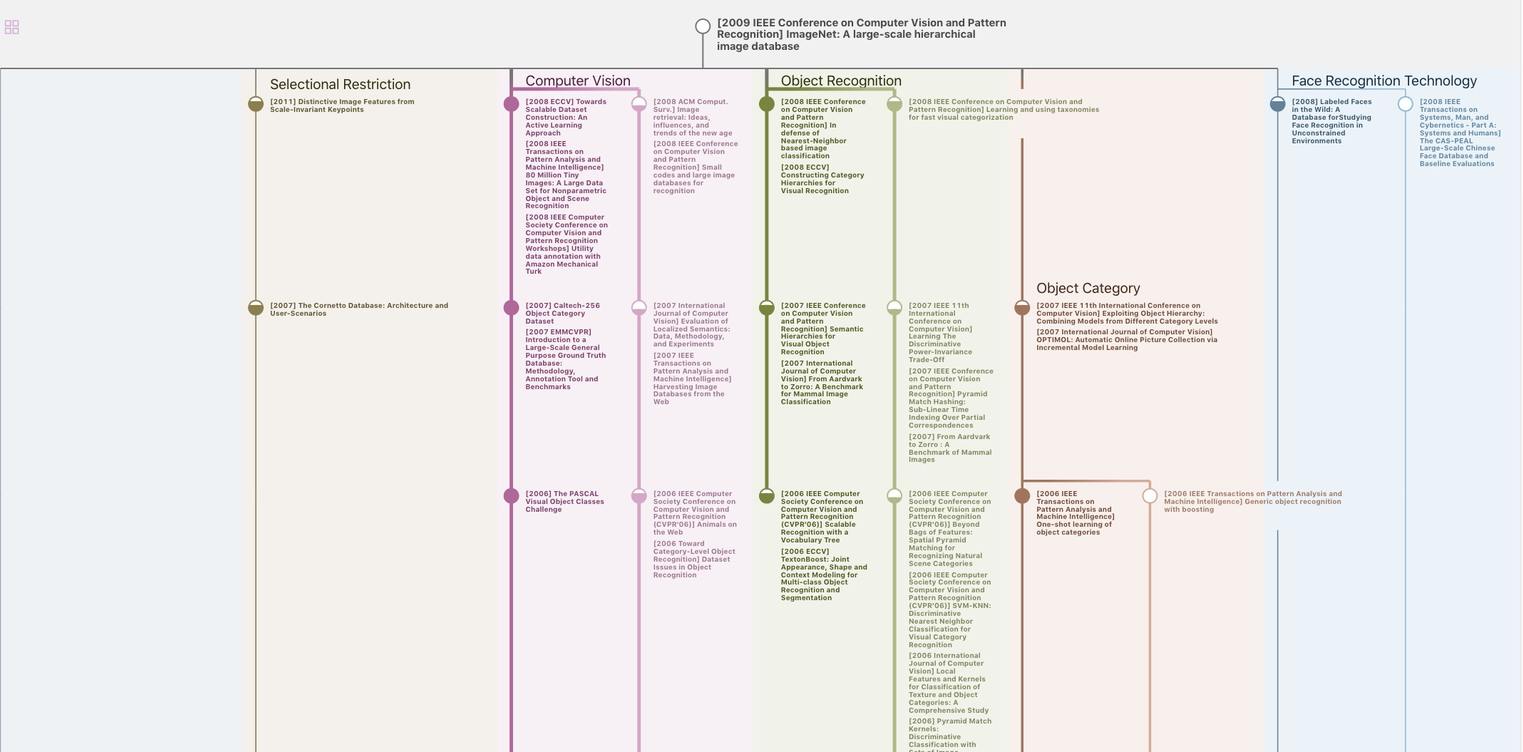
生成溯源树,研究论文发展脉络
Chat Paper
正在生成论文摘要