A Deep Generative Model-Integrated Framework for 3-D Time-Difference Electrical Impedance Tomography
IEEE Trans. Instrum. Meas.(2023)
摘要
The time-difference image reconstruction problem of electrical impedance tomography (EIT) refers to reconstructing the conductivity change in a human body part between two time points using the boundary impedance measurements. Conventionally, the problem can be formulated as a linear inverse problem. However, due to the physical property of the forward process, the inverse problem is seriously ill-posed. As a result, traditional regularized least-squares (LSs)-based methods usually produce low-resolution images that are difficult to interpret. This work proposes a framework that uses a deep generative model (DGM) to constrain the unknown conductivity. Specifically, this framework allows the inclusion of a constraint that describes a mathematical relationship between the generative model and the unknown conductivity. The resultant constrained minimization problem is solved using an extended alternating direction method of multipliers (ADMM). The effectiveness of the framework is demonstrated by the example of 3-D time-difference chest EIT imaging. Numerical experiment shows a significant improvement of the image quality compared with total variation (TV)-regularized LSs method (peak signal-to-noise ratio (PSNR) is improved by 4.3% for 10% noise and 4.6% for 30% noise; structural similarity (SSIM) is improved by 4.8% for 10% noise and 6.0% for 30% noise). Human experiments show improved correlation between the reconstructed images and images from reference techniques.
更多查看译文
关键词
Electrical impedance tomography,Conductivity,Inverse problems,Image reconstruction,Imaging,Conductivity measurement,Lung,3-D imaging,alternating direction method of multipliers (ADMM),constrained minimization,deep generative models (DGMs),electrical impedance tomography (EIT),functional imaging,lung imaging
AI 理解论文
溯源树
样例
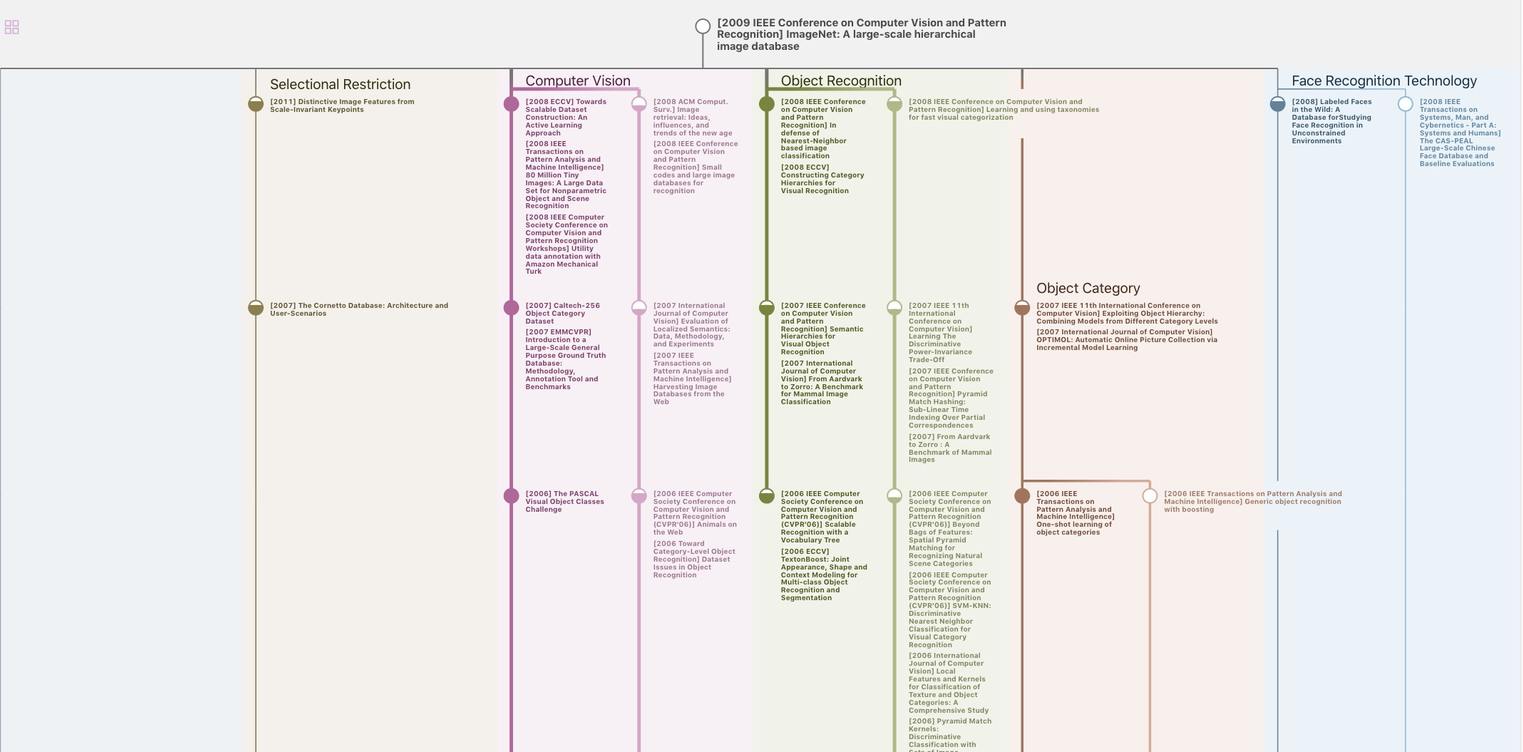
生成溯源树,研究论文发展脉络
Chat Paper
正在生成论文摘要