Region Probability Map-Guided Fast Wide-Area Multiobject Detection.
IEEE Trans. Instrum. Meas.(2023)
摘要
Detecting multiobjects in a wide-area scenario efficiently is a critical technology for industry and security applications. The detection performance has benefited enormously from the probability estimation of the unknown environment. Representative methods, such as particle filter (PF), construct the probability distribution model and iteratively locate target objects. However, randomly sampling and detecting image patches in the large covering field-of-view (FOV) make these methods inefficient and computation costly. To address these issues, we propose a region probability map (RPM)-guided fast wide-area detection system that can simultaneously detect multiobjects from a large FOV at 300 frames per second (fps) through a coarse-to-fine-grained detection paradigm. Specifically, a segmentation-based RPM generation module is introduced to assign probability measurements to different regions of the panoramic image, which models how likely the desired objects will occur in these regions. Then, based on the generated probability map of the whole scene, a novel RPM-guided PF framework is proposed to speed up the detection process by concentrating the detection on high-probability areas. Finally, a rapid and low-latency active detection system based on a wide-angle camera, a high-speed camera, and an ultrafast galvano mirror is implemented, which gains a 15.38% efficiency improvement while achieving more accurate detection compared with existing methods. Extensive experimental results verify the robustness and effectiveness of our proposed system.
更多查看译文
关键词
Cameras,Real-time systems,Object detection,Feature extraction,Search problems,Detectors,Task analysis,High-speed vision,object detection,particle filter (PF),region probability map (RPM),wide-area surveillance
AI 理解论文
溯源树
样例
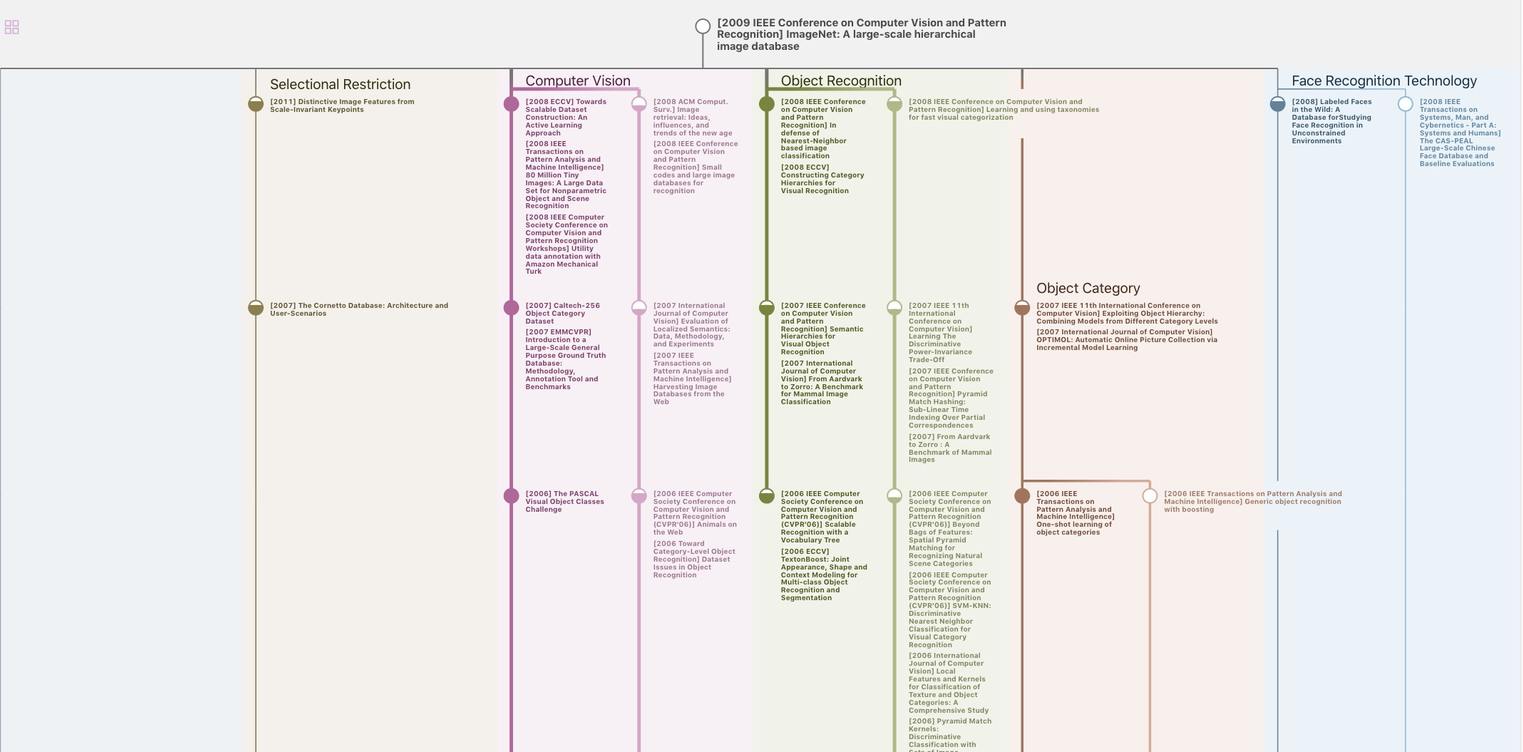
生成溯源树,研究论文发展脉络
Chat Paper
正在生成论文摘要