How to Launch Jamming Attacks on Federated Learning in NextG Wireless Networks.
GLOBECOM (Workshops)(2022)
摘要
We study how to launch jamming attacks on federated learning when performed over wireless channels in NextG systems. Federated learning has emerged as a decentralized learning framework, where clients can collectively train a global model at the server without sharing their training datasets. To support NextG wireless network applications such as spectrum sensing and user equipment identification, federated learning can be used to train machine learning models to classify wireless signals that are collected by clients (spectrum sensors) at different locations while keeping training datasets of clients confidential. Federated learning is known to be susceptible to insider threats by malicious clients. In this paper, we consider external threats in terms of the over-the-air jamming attacks on federated learning in a wireless network. We study three scenarios that an adversary can jam the local model updates transmitted from clients to the server (uplink attack), or jam the global model updates transmitted from the server to clients (downlink attack), or jam both. We impose an attack budget on the number of clients that can be attacked per federated learning round. These clients are selected based on their local model accuracies that are expected in the absence of an attack or ranked by observing the spectrum. This selection may be fixed or change over time. We show that this jamming attack leads to a major loss of performance for federated learning compared to benchmark attacks. These results raise new security concerns for federated learning when executed in NextG wireless networks subject to jamming attacks.
更多查看译文
关键词
jamming attacks,federated learning,networks
AI 理解论文
溯源树
样例
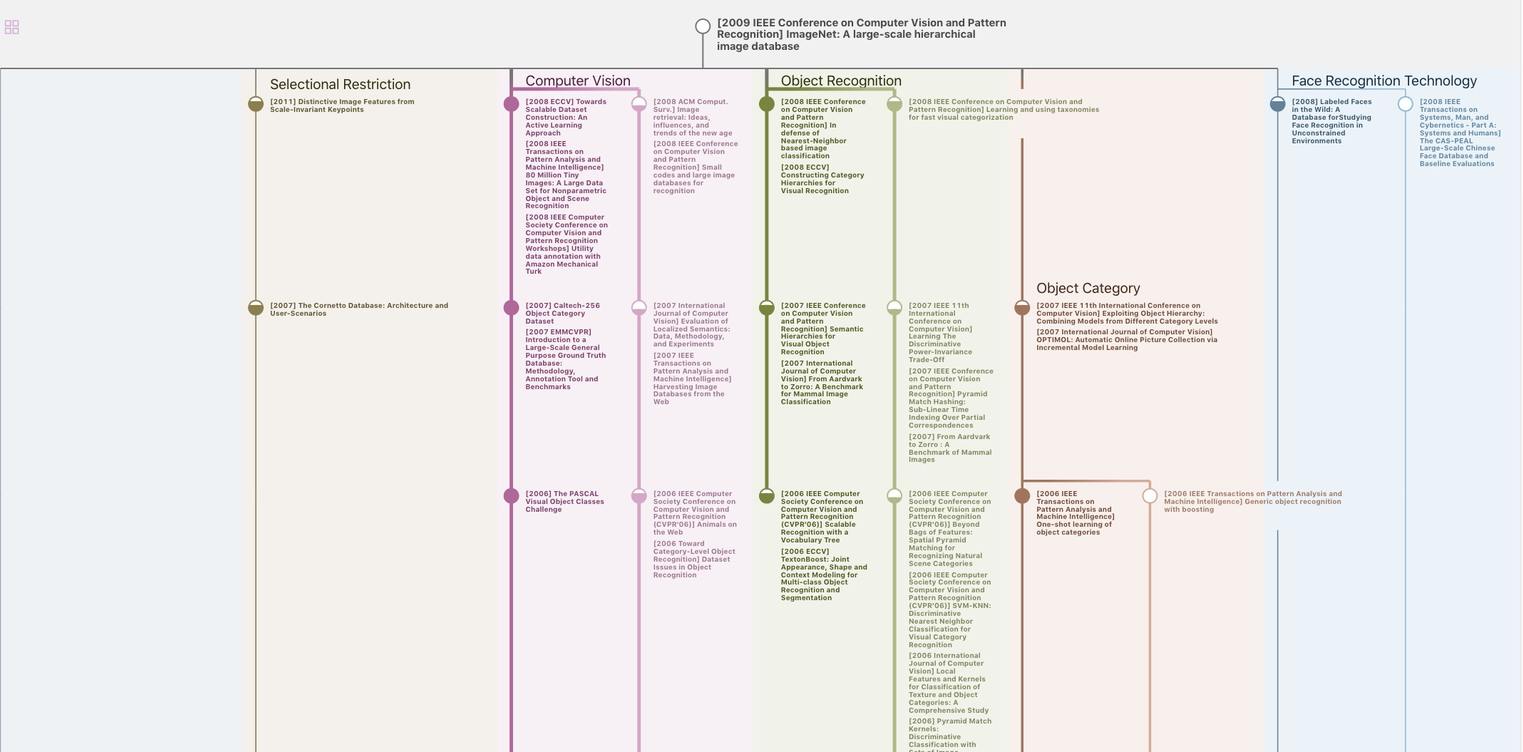
生成溯源树,研究论文发展脉络
Chat Paper
正在生成论文摘要