Optimization of Clustering Strategy and Resource Allocation for Clustered Federated Learning
GLOBECOM(2022)
摘要
Federated learning (FL) framework enables user devices collaboratively train a global model based on their local datasets without privacy leak. However, the training performance of FL is degraded when the data distributions of different devices are incongruent. Fueled by this issue, we consider a clustered FL (CFL) method where the devices are divided into several clusters according to their data distributions and are trained simultaneously. Convergence analysis is conducted, which shows that the clustered model performance depends on cosine similarity, device number per cluster, and device participation probability. Then, aiming at optimizing the model training performance, a joint problem of resource allocation and device clustering is formulated, which is solved by decoupling it into two sub-problems. Specifically, a coalition formation algorithm is proposed for the device clustering sub-problem, and the sub-problem of bandwidth allocation and transmit power control is solved directly due to its convexity. Finally, simulation experiments are conducted on the MNIST dataset to validate the performance of the proposed algorithm in terms of test accuracy.
更多查看译文
关键词
clustering strategy,resource allocation,learning
AI 理解论文
溯源树
样例
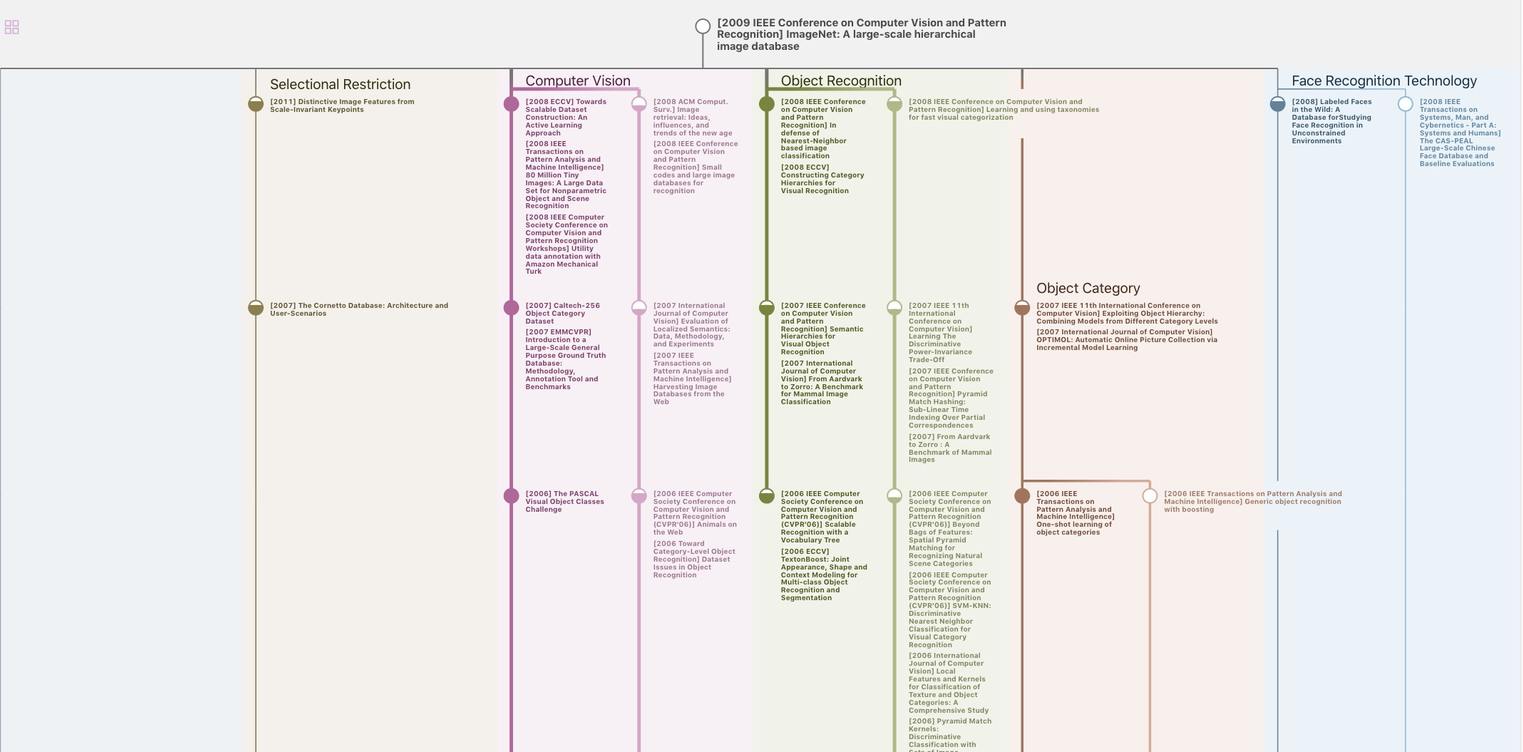
生成溯源树,研究论文发展脉络
Chat Paper
正在生成论文摘要