Distributed Reinforcement Learning for Low-delay Uplink User Scheduling in Multicell Networks.
GLOBECOM(2022)
摘要
In this paper we investigate the problem of uplink scheduling in a multicell system in order to minimize the total queuing delay at the mobile devices. The proposed setting introduces an environment with multiple interacting decision makers: Base Stations are considered as agents who have partial view of the system and interact with each other through interference generated by their scheduled devices for uplink transmissions. In addition, since traffic and channel processes are unknown, finding the optimal global scheduling policy can be modeled as a multiagent reinforcement learning problem. In this work, we propose distributed learning algorithms based on policy gradient to tackle this problem and investigate the impact of information exchange between Base Stations. Our results illustrate that the proposed algorithm outperforms standard schedulers, such as Proportional Fair and MaxWeight and that information exchange is crucial for challenging problem instances, such as topologies with many devices on the cell edge and/or high traffic demands.
更多查看译文
关键词
reinforcement learning,networks,low-delay
AI 理解论文
溯源树
样例
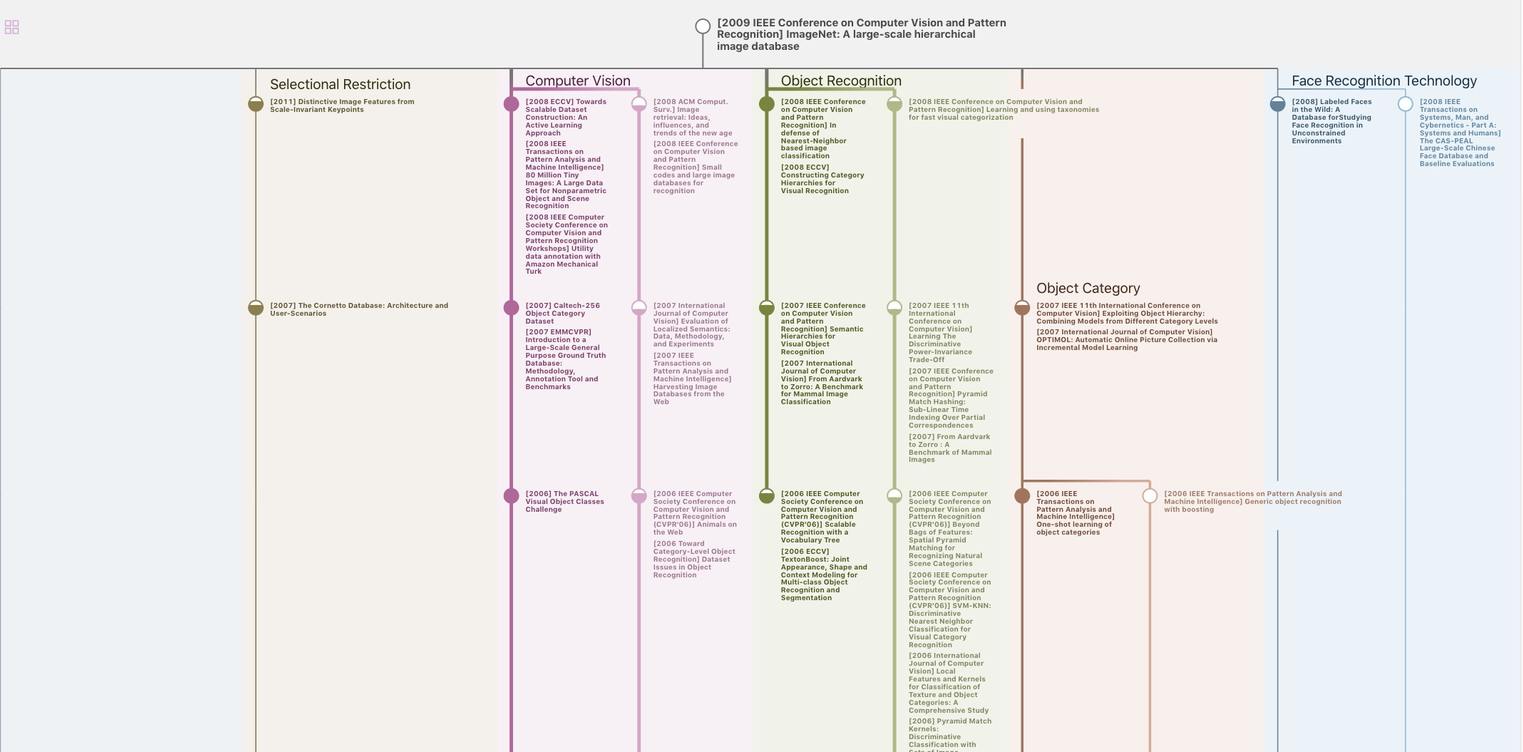
生成溯源树,研究论文发展脉络
Chat Paper
正在生成论文摘要