Improving Scalability of 6G Network Automation with Distributed Deep Q-Networks.
GLOBECOM(2022)
摘要
In recent years, owing to the architectural evolution of 6G towards decentralization, distributed intelligence is being studied extensively for 6G network automation. Distributed intelligence, based on Reinforcement Learning (RL), particularly Q-Learning (QL), has been proposed as a potential direction. The distributed framework consists of independent QL agents, attempting to reach their own individual objectives. The agents need to learn using a sufficient number of training steps before they converge to the optimal performance. After convergence, they can take reliable management actions. However, the scalability of QL could be severely hindered, particularly in the convergence time - when the number of QL agents increases. To overcome the scalability issue of QL, in this paper, we explore the potentials of the Deep Q-Network (DQN) algorithm, a function approximation-based method. Results show that DQN outperforms QL by at least 37% in terms of convergence time. In addition, we highlight that DQN is prone to divergence, which, if solved, could rapidly advance distributed intelligence for 6G.
更多查看译文
关键词
6G,network automation,architecture,resource management,Deep Learning,Reinforcement Learning,DQN
AI 理解论文
溯源树
样例
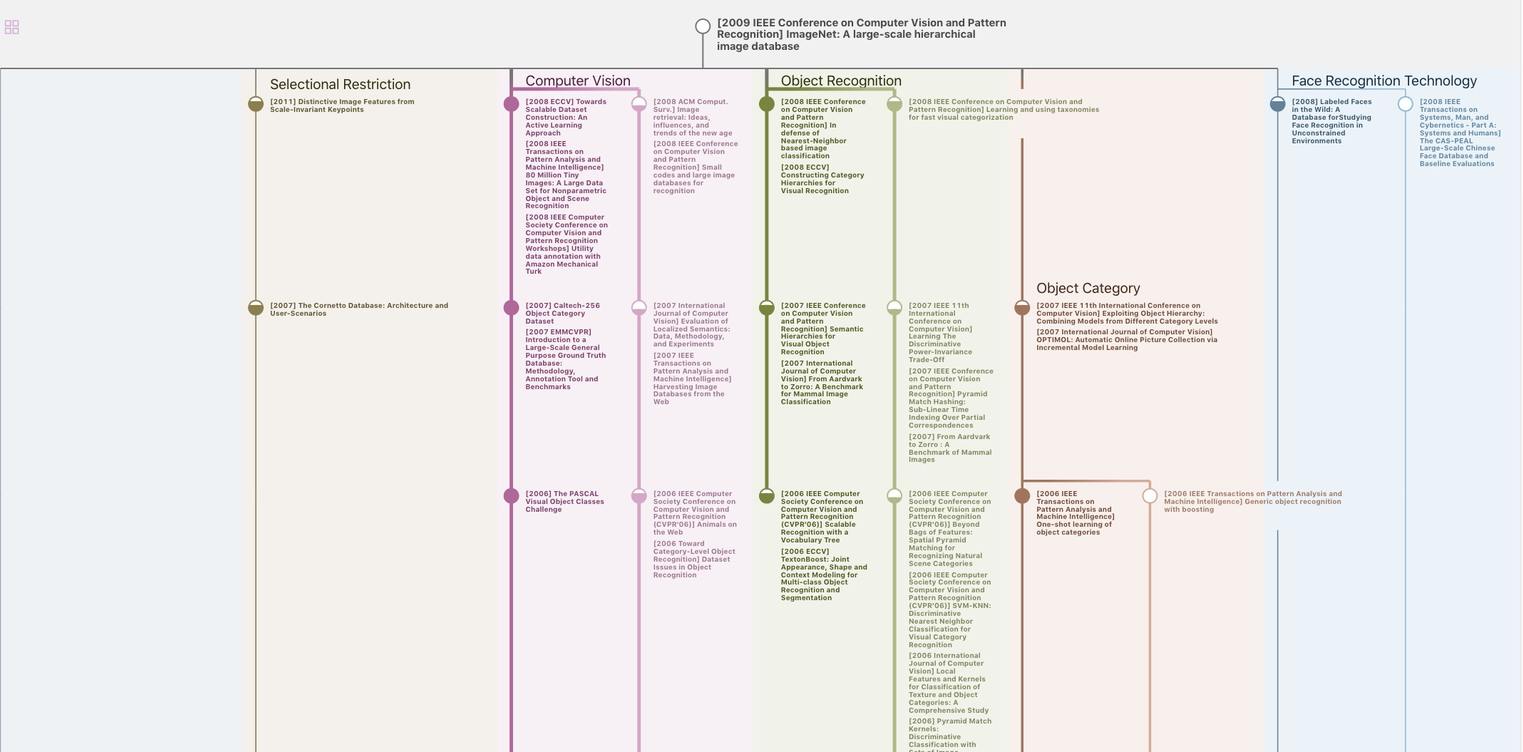
生成溯源树,研究论文发展脉络
Chat Paper
正在生成论文摘要