Neural network Based tuning of the Initial Congestion Window of Thin-streamed Application Traffic.
GLOBECOM (Workshops)(2022)
摘要
The thin-streamed application traffic (such as gaming and Internet of Things IoT) has recently dominated internet traffic. The TCP congestion control mechanisms are mainly designed for large flows to improve the throughput and maintain fairness. The initial congestion window impacts the thin-stream application flow completion time (FCT) to a more considerable extent. We propose a simple Neural network-based initial congestion window (INITCWND) tuning that understands the network conditions and thereby adapts the INITCWND to an optimal value. We evaluate our proposed method with the legacy static INITCWND with the default value. Our simulation and live-air experimental results show that our proposed method enhances the FCT significantly compared to the legacy method. We also offer a per-socket INITCWND tuning that can help the application set different INITCWND based on the type of service.
更多查看译文
关键词
initial congestion window,application traffic,neural network,tuning,thin-streamed
AI 理解论文
溯源树
样例
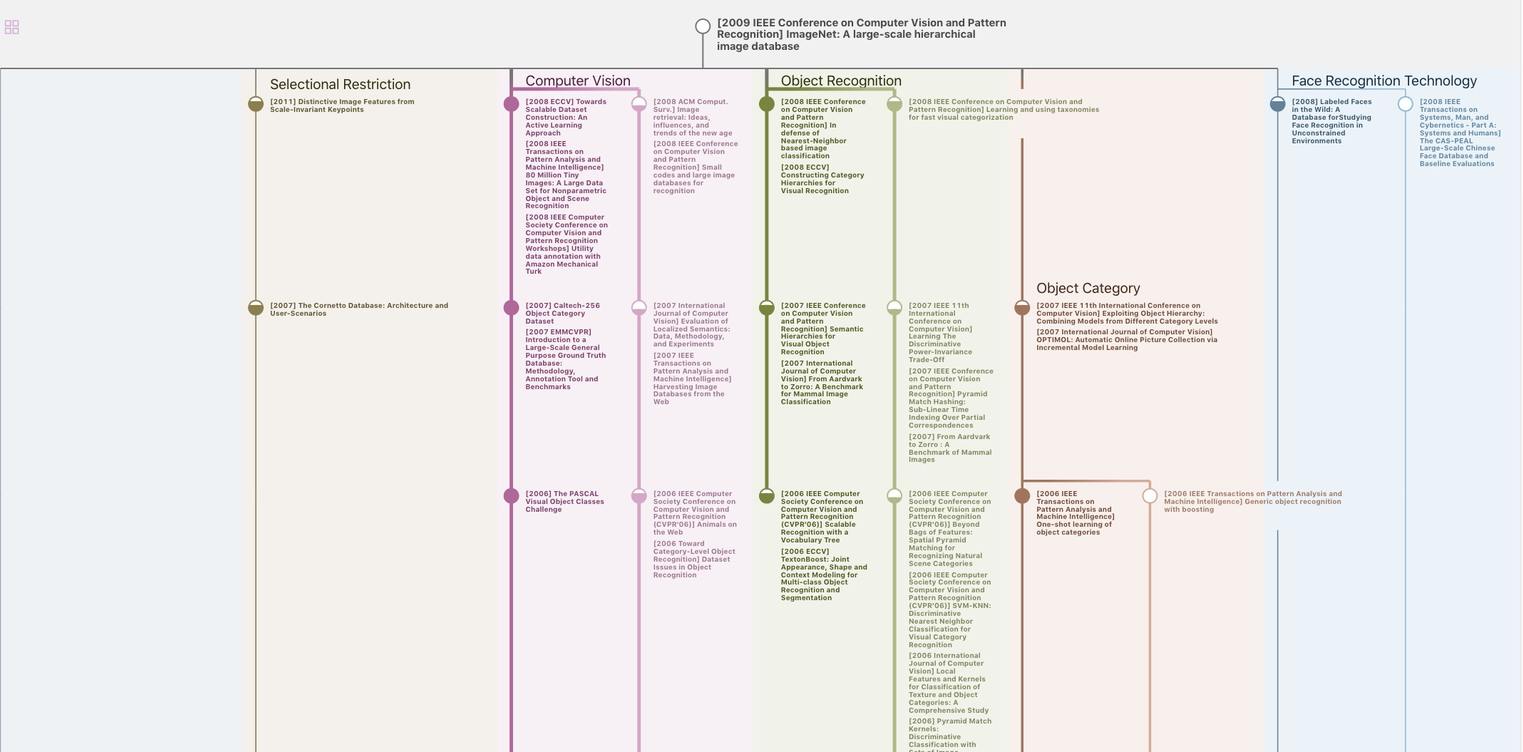
生成溯源树,研究论文发展脉络
Chat Paper
正在生成论文摘要